Data fusion strategies for improving resilience to sensor noise in cable-stayed tower monitoring
2022 25th International Conference on Information Fusion (FUSION)(2022)
摘要
Power outages pose meaningful economic and social impacts on communities around the world. However, society's increasing reliance on electricity reduces the tolerance for power outages and consequently highlights the need to enhance the power grid resilience against natural hazards. For example, power lines based on cable-stayed towers must take special care to avoid cable loosening or foundation settlement, leading to tower collapse and cascading power failures. Our work uses a data fusion strategy to improve the inference quality of faulty or noisy sensors in remote monitoring. Machine Learning (ML) models based on Feedforward Neural Networks (FNN) and Principal Component Analysis (PCA) are used to predict expected values based on correlated sensor data. Our experiments compare the data fusion approaches with the ground truth values of inclination and cable tension. We show that the strategies with PCA and FNN and only with FNN reduced the Mean Absolute Percentage Error (MAPE) for cable tension estimation by 54% and 65% on average, respectively, with a corresponding error reduction of 37% and 54% on average for tower displacement estimation.
更多查看译文
关键词
data fusion,sensor noise,improving data sensor
AI 理解论文
溯源树
样例
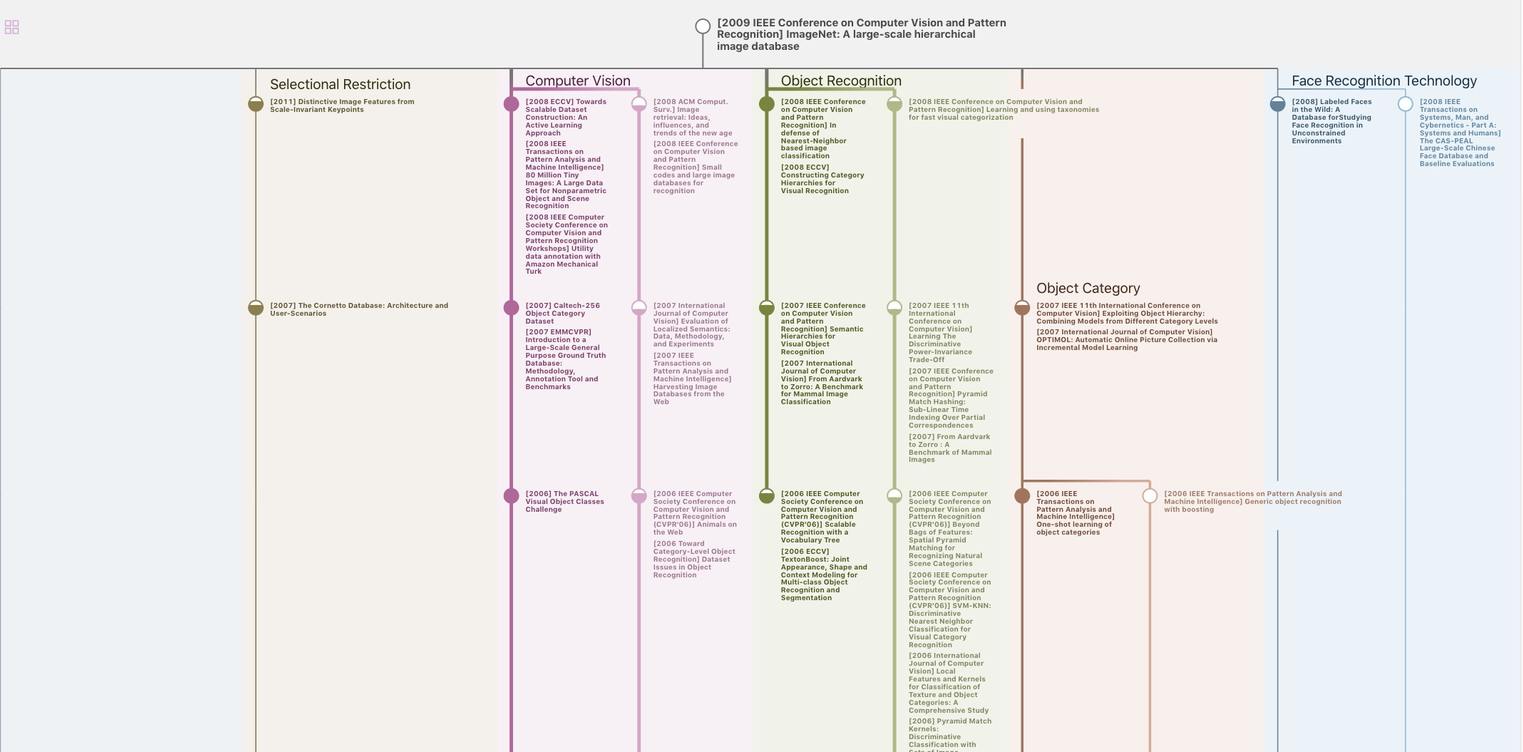
生成溯源树,研究论文发展脉络
Chat Paper
正在生成论文摘要