Information block multi-head subspace based long short-term memory networks for sentiment analysis
Applied Intelligence(2022)
摘要
Sentiment analysis is a vital task in the domain of natural language processing for semantic handling. Numerous neural network systems are introduced into sentiment analysis by researchers, some of which deal with textual datasets. These models are frequently built using LSTM sequence models and attention mechanism. However, these models have some obvious flaws. For LSTM family networks, if the sequence is too long, the information about the long-distance sequence will be lost, and the gradient explosion will almost certainly occur. Additionally, these methods directly connect bidirectional hidden vectors, resulting in information redundancy. Multi-head attention is a variant of attention mechanism, and it is also widely used to process textual information in parallel. However, multi-head subspace information is also used in a mixed linear mapping, resulting in insufficient information usage. Further, multi-head attention calculates a long sequence of text, which has a large complexity overhead. To address these issues and boost performance, firstly, a complete sentence is divided into multiple information blocks. Then, we designed a unique model to input the information block into multi-head attention for parallel multi-space feature extraction; Furthermore, the output multi-subspace information is fed into the LSTM network that processes the subspace information to fully flow the message and to obtain the hidden states at each information block time step in each subspace. Blocking the sequence can not only reduce the number of cycles of LSTM, but also reduce the computational complexity of multi-head attention. Eventually, a dual fusion mechanism is presented that allows for the fusion and seizing of each subspace significant information. Experiments on real-world datasets demonstrate that our proposed model outperforms the majority of existing methods.
更多查看译文
关键词
Multi-head subspace,Information block,Self-attention,Bidirectional long short-term memory,A dual fusion mechanism,Sentiment analysis
AI 理解论文
溯源树
样例
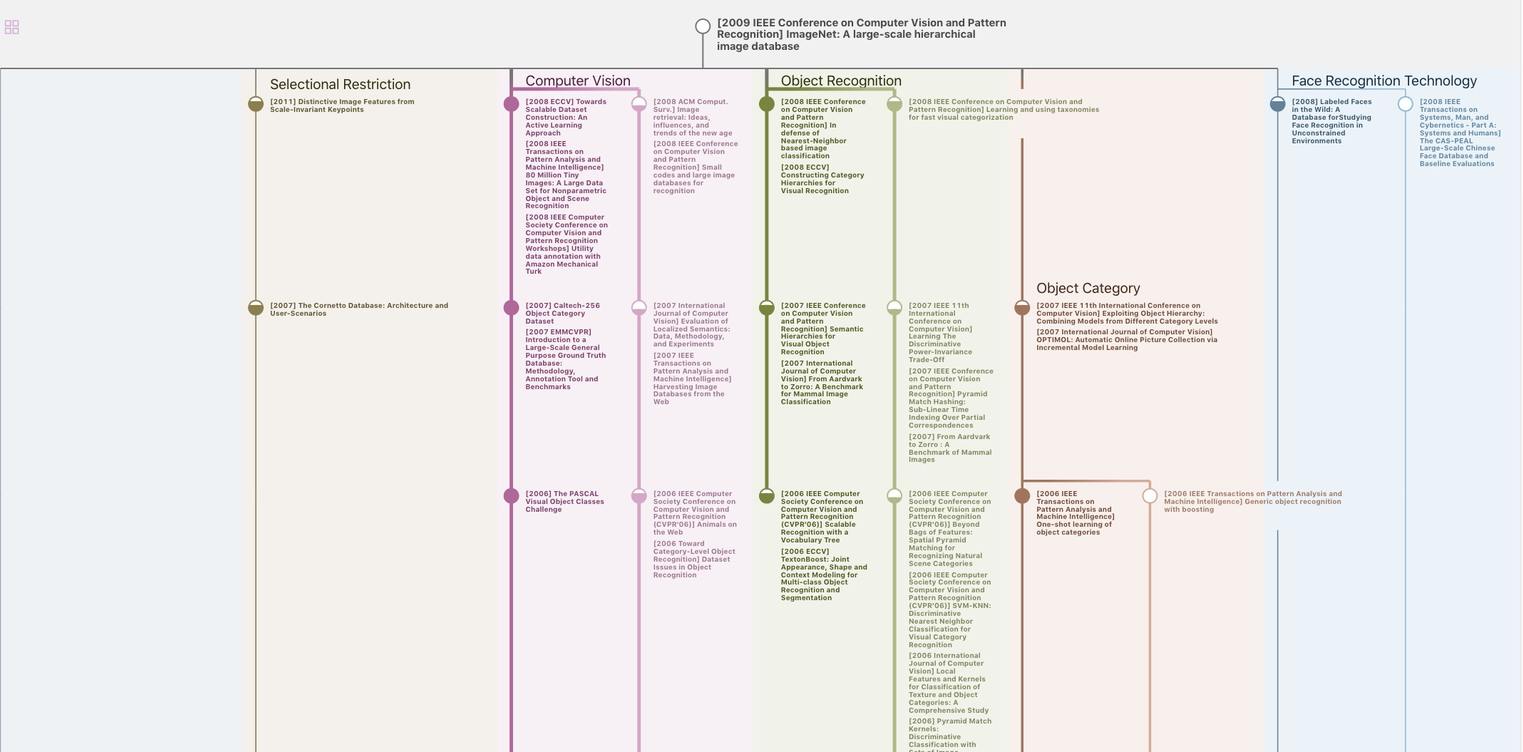
生成溯源树,研究论文发展脉络
Chat Paper
正在生成论文摘要