Apply an optimized NN model to low-dimensional format speech recognition and exploring the performance with restricted factors
MEASUREMENT & CONTROL(2023)
摘要
The SCD (speech control detection) have received a lot of attention in recent years. A framework established by employing DNN-LSTM (deep neural network-long-short term memory) model for speech and text recognition is implemented in the current article. The performance of the build framework is analyzed with many different merits which consider many features, such as (with and without) noise, track number of speeches (ST (single track) and DT (double track)), and dropout ratio of data training. On the other hand, the speech discriminator model is developed and implemented with the DNN-LSTM framework, and the data sets are collected by four different persons. The adopted model performance is evaluated using the four different datasets, and each with 400-5000 training times. There are three parameters considered as the dominators for the performance evaluation of the completed speech platform. The results from the experiment with DT channel case clearly show that it outperforms the case with ST channel. It can see that the accuracy of the DNN-LSTM model increases from 0.3339 to 0.9696 and the loss rate decreases from 1.09984 to 0.19298 after adjusting the dropout ratio during the training step. This shows that the dropout ratio also dominates the accuracy and loss rate. Eventually, the results indicate that the used model compared to other similar methods, Bi-LSTM (bi-directional LSTM), achieves a more efficient preserving a high accuracy level.
更多查看译文
关键词
Bi-LSTM, DNN-LSTM, dropout ratio, machine learning, SCD
AI 理解论文
溯源树
样例
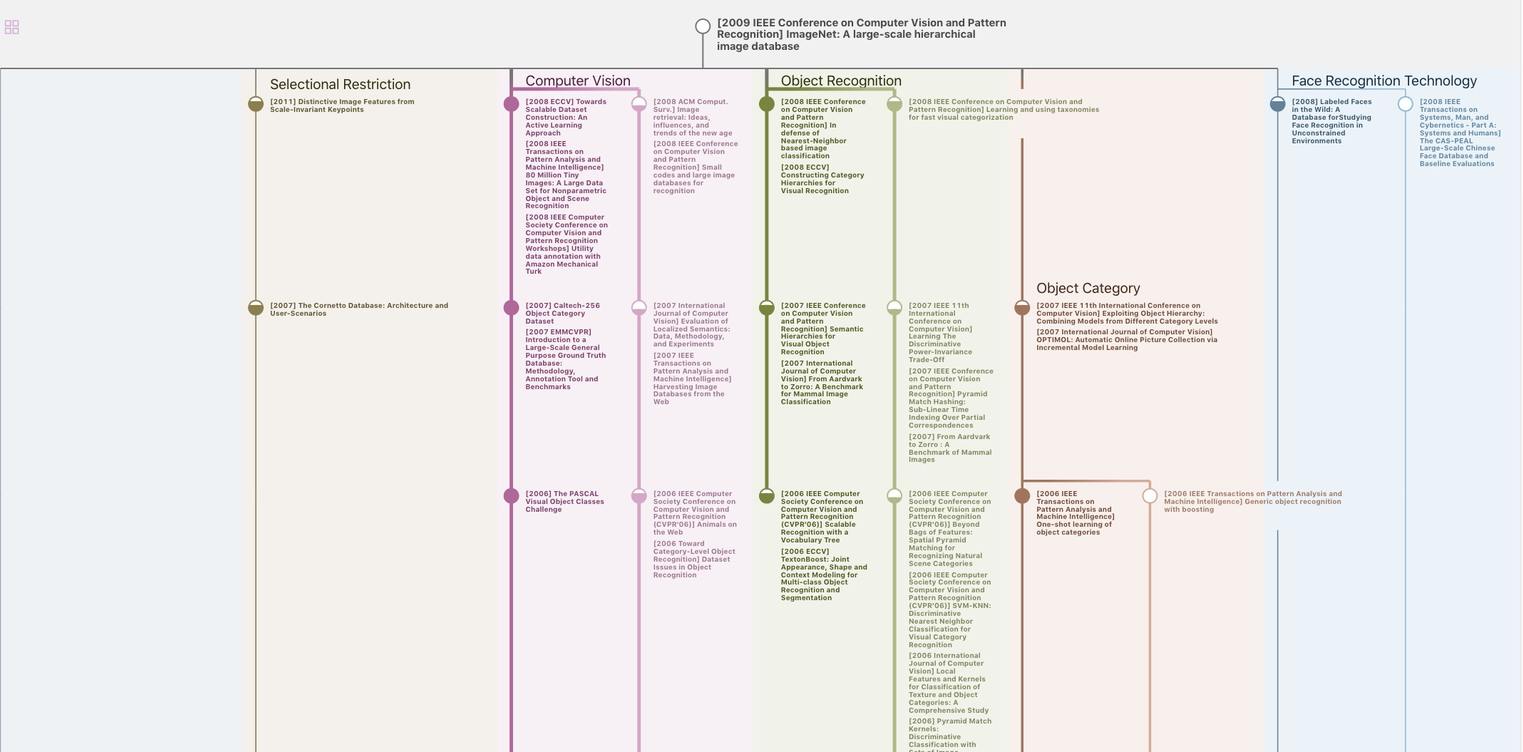
生成溯源树,研究论文发展脉络
Chat Paper
正在生成论文摘要