Distribution Shift Detection for Deep Neural Networks
ICLR 2023(2022)
摘要
To deploy and operate deep neural models in production, the quality of their predictions, which might be contaminated benignly or manipulated maliciously by input distributional deviations, must be monitored and assessed. Specifically, we study the case of monitoring the healthy operation of a deep neural network (DNN) receiving a stream of data, with the aim of detecting input distributional deviations over which the quality of the network's predictions is potentially damaged. Using selective prediction principles, we propose a distribution deviation detection method for DNNs. The proposed method is derived from a tight coverage generalization bound computed over a sample of instances drawn from the true underlying distribution. Based on this bound, our detector continuously monitors the operation of the network over a test window and fires off an alarm whenever a deviation is detected. This novel detection method consistently and significantly outperforms the state of the art with respect to the CIFAR-10 and ImageNet datasets, thus establishing a new performance bar for this task, while being substantially more efficient in time and space complexities.
更多查看译文
关键词
Selective classification,Window based shift detection
AI 理解论文
溯源树
样例
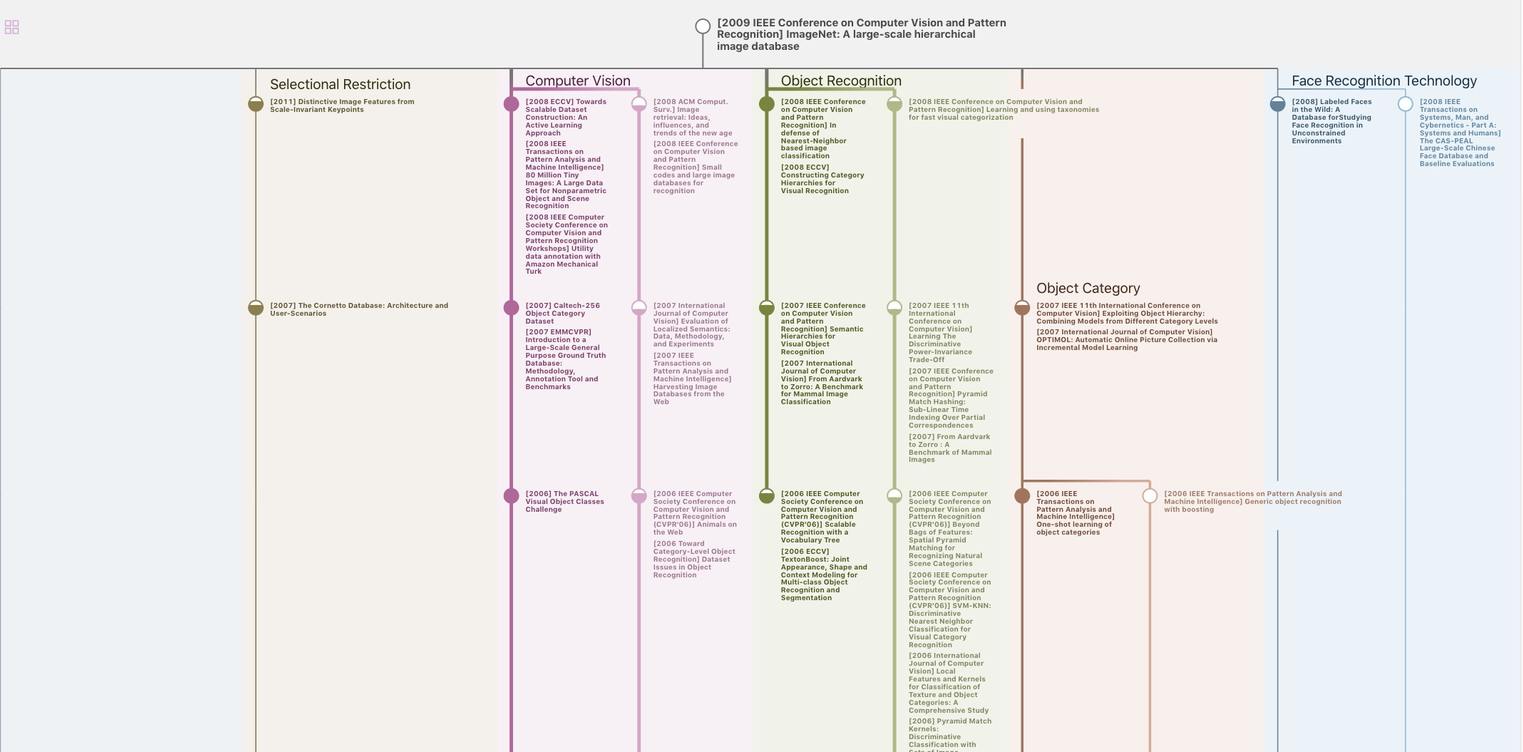
生成溯源树,研究论文发展脉络
Chat Paper
正在生成论文摘要