DnA: Improving Few-Shot Transfer Learning with Low-Rank Decomposition and Alignment
Computer Vision – ECCV 2022(2022)
摘要
Self-supervised (SS) learning has achieved remarkable success in learning strong representation for in-domain few-shot and semi-supervised tasks. However, when transferring such representations to downstream tasks with domain shifts, the performance degrades compared to its supervised counterpart, especially at the few-shot regime. In this paper, we proposed to boost the transferability of the self-supervised pre-trained models on cross-domain tasks via a novel self-supervised alignment step on the target domain using only unlabeled data before conducting the downstream supervised fine-tuning. A new reparameterization of the pre-trained weights is also presented to mitigate the potential catastrophic forgetting during the alignment step. It involves low-rank and sparse decomposition, that can elegantly balance between preserving the source domain knowledge without forgetting (via fixing the low-rank subspace), and the extra flexibility to absorb the new out-of-the-domain knowledge (via freeing the sparse residual). Our resultant framework, termed Decomposition-and-Alignment (DnA), significantly improves the few-shot transfer performance of the SS pre-trained model to downstream tasks with domain gaps. (The code is released at
https://github.com/VITA-Group/DnA
).
更多查看译文
关键词
Self-supervised learning, Transfer few-shot, Low-rank
AI 理解论文
溯源树
样例
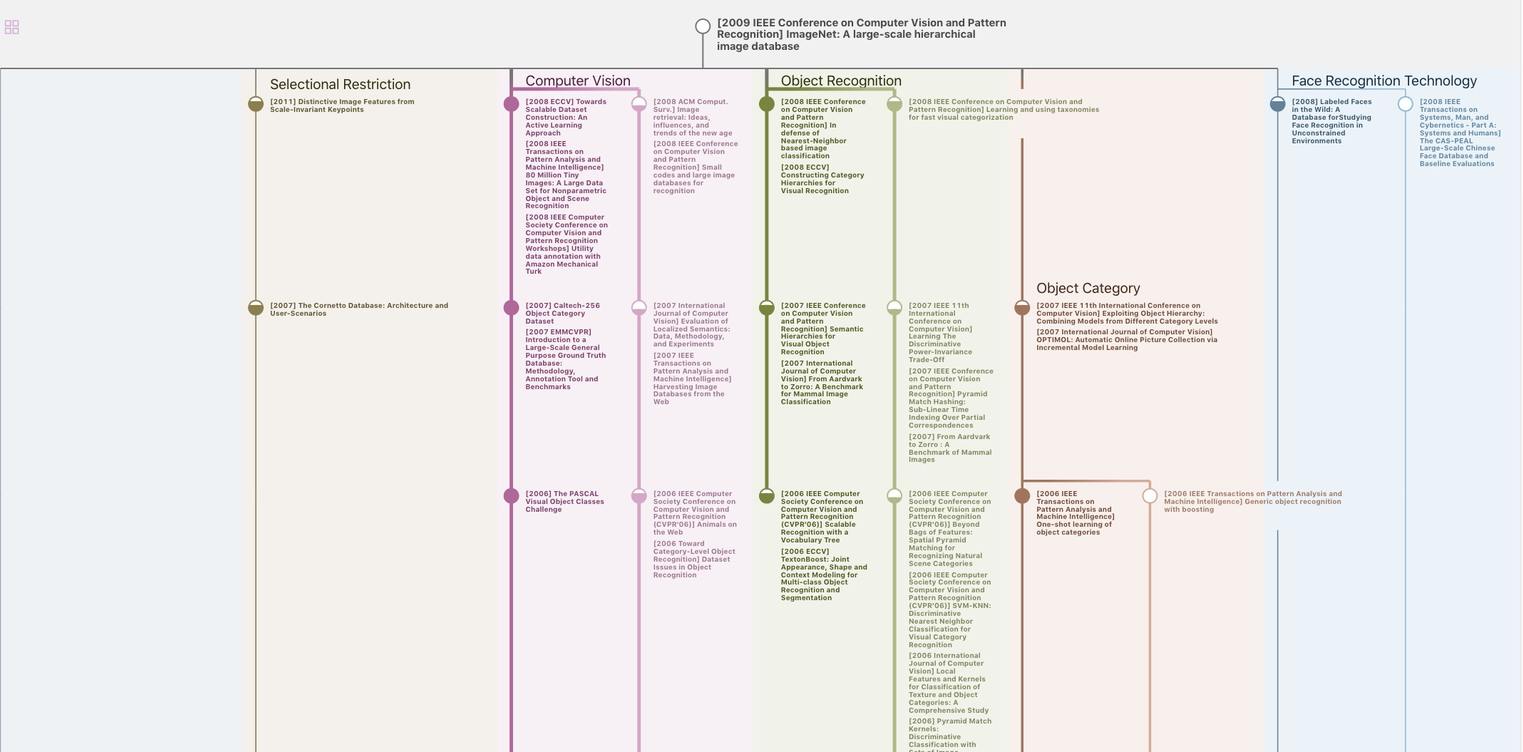
生成溯源树,研究论文发展脉络
Chat Paper
正在生成论文摘要