Non-Submodular Maximization via the Greedy Algorithm and the Effects of Limited Information in Multi-Agent Execution.
IEEE/RJS International Conference on Intelligent RObots and Systems (IROS)(2022)
摘要
We provide theoretical bounds on the worst case performance of the greedy algorithm in seeking to maximize a normalized, monotone, but not necessarily submodular objective function under a simple partition matroid constraint. We also provide worst case bounds on the performance of the greedy algorithm in the case that limited information is available at each planning step. We specifically consider limited information as a result of unreliable communications during distributed execution of the greedy algorithm. We utilize notions of curvature for normalized, monotone set functions to develop the bounds provided in this work. To demonstrate the value of the bounds provided in this work, we analyze a variant of the benefit of search objective function and show, using real-world data collected by an autonomous underwater vehicle, that theoretical approximation guarantees are achieved despite non-submodularity of the objective function.
更多查看译文
关键词
greedy algorithm,monotone set functions,multiagent execution,nonsubmodular maximization,nonsubmodularity,simple partition matroid constraint,submodular objective function,theoretical bounds,worst case performance
AI 理解论文
溯源树
样例
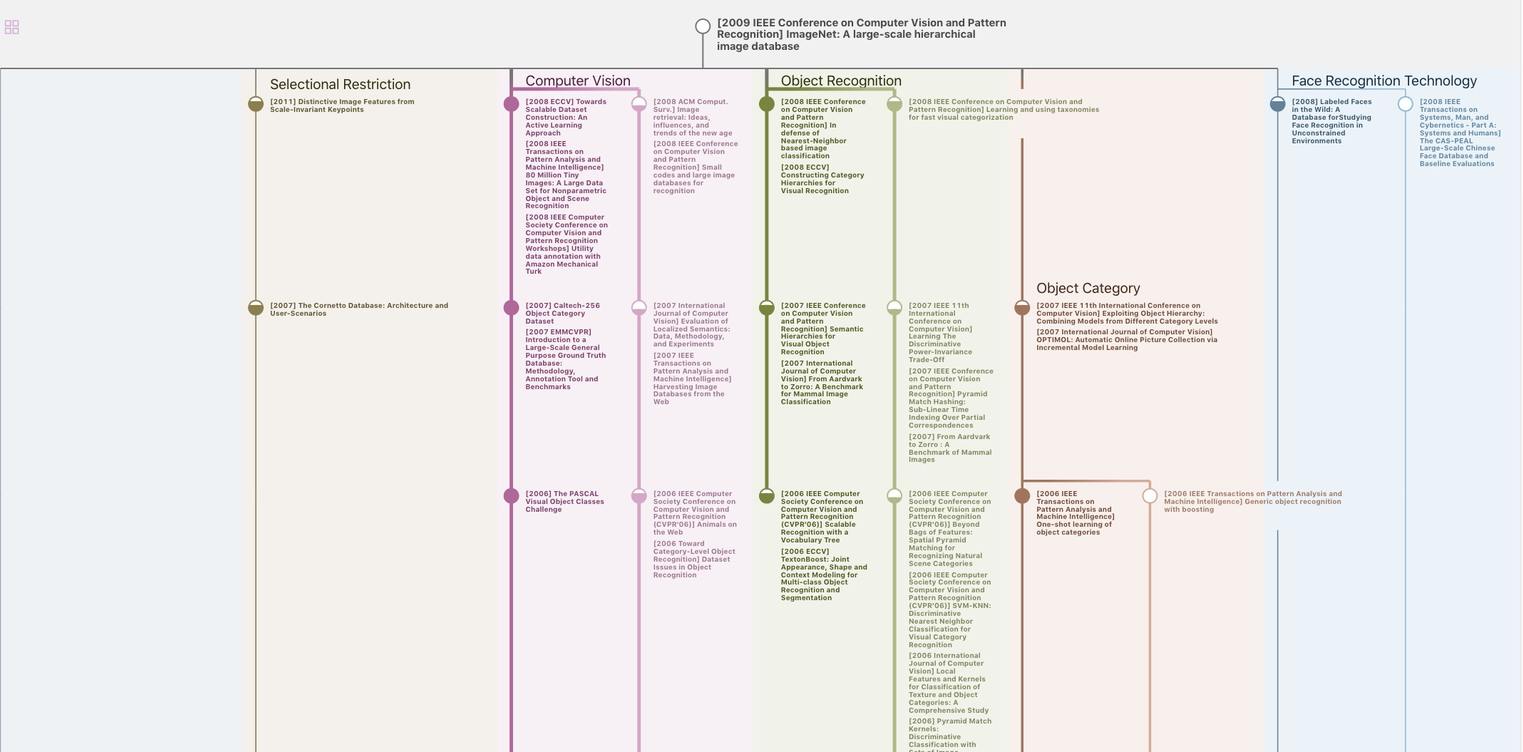
生成溯源树,研究论文发展脉络
Chat Paper
正在生成论文摘要