Fast Algorithm for Big Data Summarization with Knapsack and Partition Matroid Constraints
2022 International Conference on INnovations in Intelligent SysTems and Applications (INISTA)(2022)
摘要
As an effective tool to extract representative summary from big data, data summarization is often cast into a submodular maximization problem. Although submodular maximization problem has a long research history, and many related algorithms have been born, these algorithms often have high computational complexity and are difficult to apply to the field of big data. Therefore, in recent years, research on low-time complexity algorithms has attracted extensive attention. In this paper, we mainly focus on the non-monotone submodular maximization problem under the setting of a knapsack and a partition matroid constraints. To solve it, we design a practical, effective and efficient algorithm called FASKP, that can achieve an approximation ratio of near 7.2 + ϵ using near linear runing time. As far as we know, the FASKP algorithm achieves the best approximate guarantee compared to existing algorithms with low-time complexity. Furthermore, we demonstrate how to apply FASKP in three real data summarization applications: image summarization (10K images), movie recommendation (11K movies), and revenue maximization on social networks (Youtube). Experimental results in real scenarios show that, compared with existing algorithms, the FASKP algorithm can consistently obtain the highest utility, which validates its superiority.
更多查看译文
关键词
big data summarization,submodular optimization,approximation algorithm
AI 理解论文
溯源树
样例
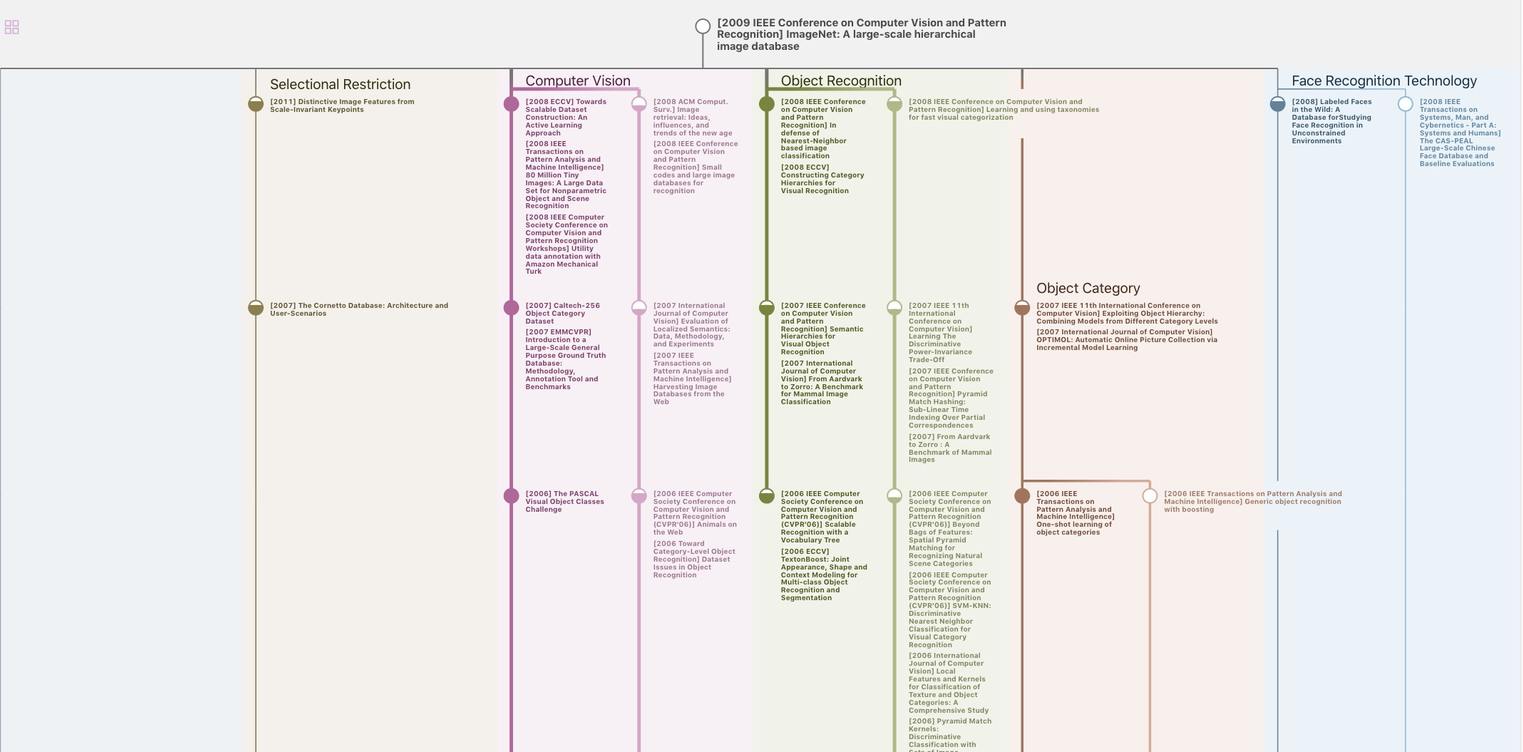
生成溯源树,研究论文发展脉络
Chat Paper
正在生成论文摘要