Physics-Aware Fast Learning and Inference for Predicting Active Set of DC-OPF
2022 IEEE Power & Energy Society Innovative Smart Grid Technologies Conference (ISGT)(2022)
摘要
DC-OPF stands as the cornerstone for efficient and secure operations of power systems. The grid operators need to solve DC-OPF repeatedly and in large numbers to maintain the balance of electricity supply and demand, especially under high penetration of renewable energies. Recently, research efforts have been made in predicting the optimal active sets as a key component in learning-based solvers for DC-OPF. In this paper, we investigate the classifiers that inherently exploit a key physical property of the optimal solutions of DC-OPF: the input space corresponding to an optimal active set is a polyhedron, and the classes of different active sets are linearly separable. In particular, we investigate the effectiveness of linear discriminant analysis (LDA) classifiers for predicting the optimal active sets for DC-OPF. This is because LDA, as a natural multi-class classifier, by definition guarantees that the decision regions for all the classes are polyhedrons. Simulations are conducted on the IEEE–162 bus test case with a 50% renewable penetration level provided by 37 renewable power producers. We examine LDA as well as other classifier candidates, namely support vector machines, neural networks, and gradient boosted decision trees. The numerical results suggest that LDA a) achieves a testing performance in accuracy and in run-time similar to carefully trained neural networks, and b) is also much faster and easier to train than the other more complicated algorithms compared. Given the highly competitive testing accuracy, extremely fast training and testing, and the straightforward application to any problem setting without the need of algorithm tuning, we advocate that LDA is a top choice of learning-based algorithm for predicting the optimal active set for DC-OPF.
更多查看译文
关键词
grid operators,gradient boosted decision trees,neural networks,support vector machines,renewable power producers,IEEE-162 bus test case,LDA classifiers,linear discriminant analysis classifiers,learning-based solvers,renewable energies,physics-aware fast learning,optimal active set,DC-OPF
AI 理解论文
溯源树
样例
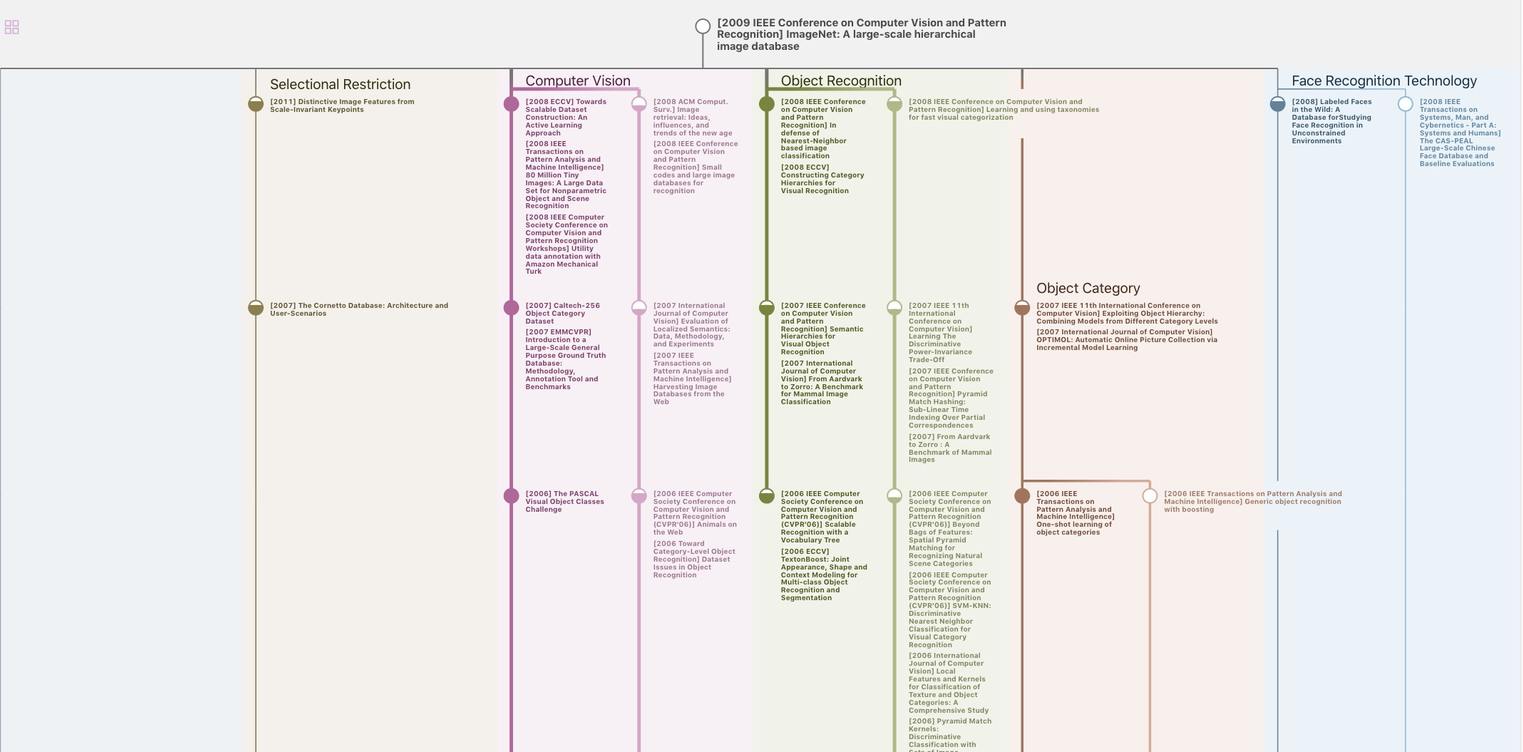
生成溯源树,研究论文发展脉络
Chat Paper
正在生成论文摘要