Light field image super-resolution based on raw data with transformers
Journal of the Optical Society of America A(2022)
摘要
Light field (LF) image super-resolution (SR) can improve the limited spatial resolution of LF images by using com-plementary information from different perspectives. However, current LF image SR methods only use the RGB data to implicitly exploit the information among different perspectives, without paying attention to the informa-tion loss from raw data to RGB data and the explicit structure information utilization. To address the first issue, a data generation pipeline is developed to collect LF raw data for LF image SR. In addition, to make full use of the multiview information, an end-to-end convolutional neural network architecture (namely, LF-RawSR) is proposed for LF image SR. Specifically, an aggregated module is first used to fuse the angular information based on a volume transformer with plane sweep volume. Then the aggregated feature is warped to all LF views using a cross-view transformer for nonlocal dependencies utilization. The experimental results demonstrate that our method outper-forms existing state-of-the-art methods with a comparative computational cost, and fine details and clear structures can be restored.(c) 2022 Optica Publishing Group
更多查看译文
关键词
transformers,raw data,super-resolution
AI 理解论文
溯源树
样例
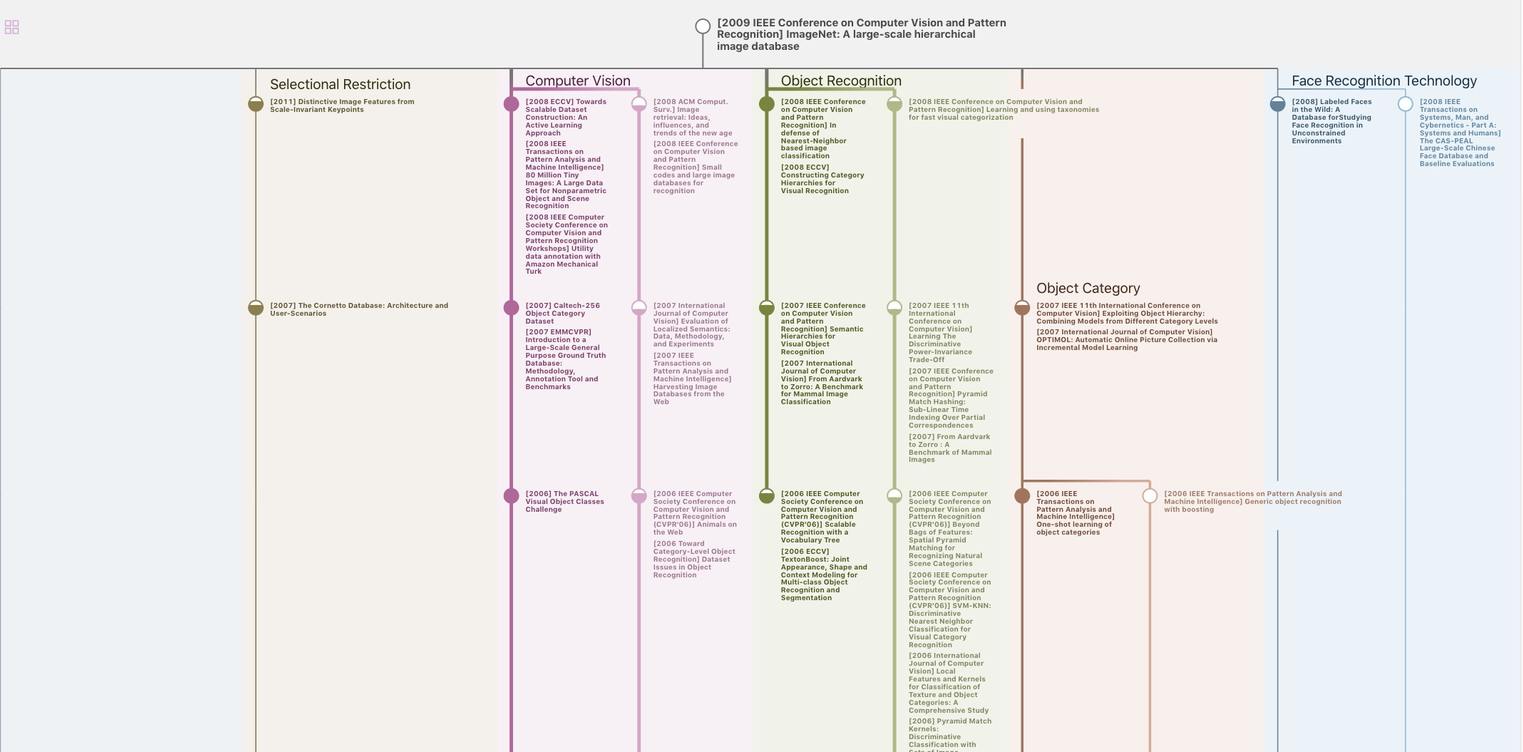
生成溯源树,研究论文发展脉络
Chat Paper
正在生成论文摘要