Limitations of the Use of Neural Networks in Black Box Cryptanalysis
Innovative Security Solutions for Information Technology and Communications(2022)
摘要
In this work, we first abstract a block cipher to a set of parallel Boolean functions. Then, we establish the conditions that allow a multilayer perceptron (MLP) neural network to correctly emulate a Boolean function. We extend these conditions to the case of any block cipher. The modeling of the block cipher is performed in a black box scenario with a set of random samples, resulting in a single secret key chosen plaintext/ciphertext attack. Based on our findings we explain the reasons behind the success and failure of relevant related cases in the literature. Finally, we conclude by estimating what are the resources to fully emulate 2 rounds of AES-128, a task that has never been achieved by means of neural networks. Despite the presence of original results and observations, we remark the systematization of knowledge nature of this work, whose main point is to explain the reason behind the inefficacy of the use of neural networks for black box cryptanalysis.
更多查看译文
关键词
Black-box, Cryptanalysis, Neural networks, Cipher emulation, AES
AI 理解论文
溯源树
样例
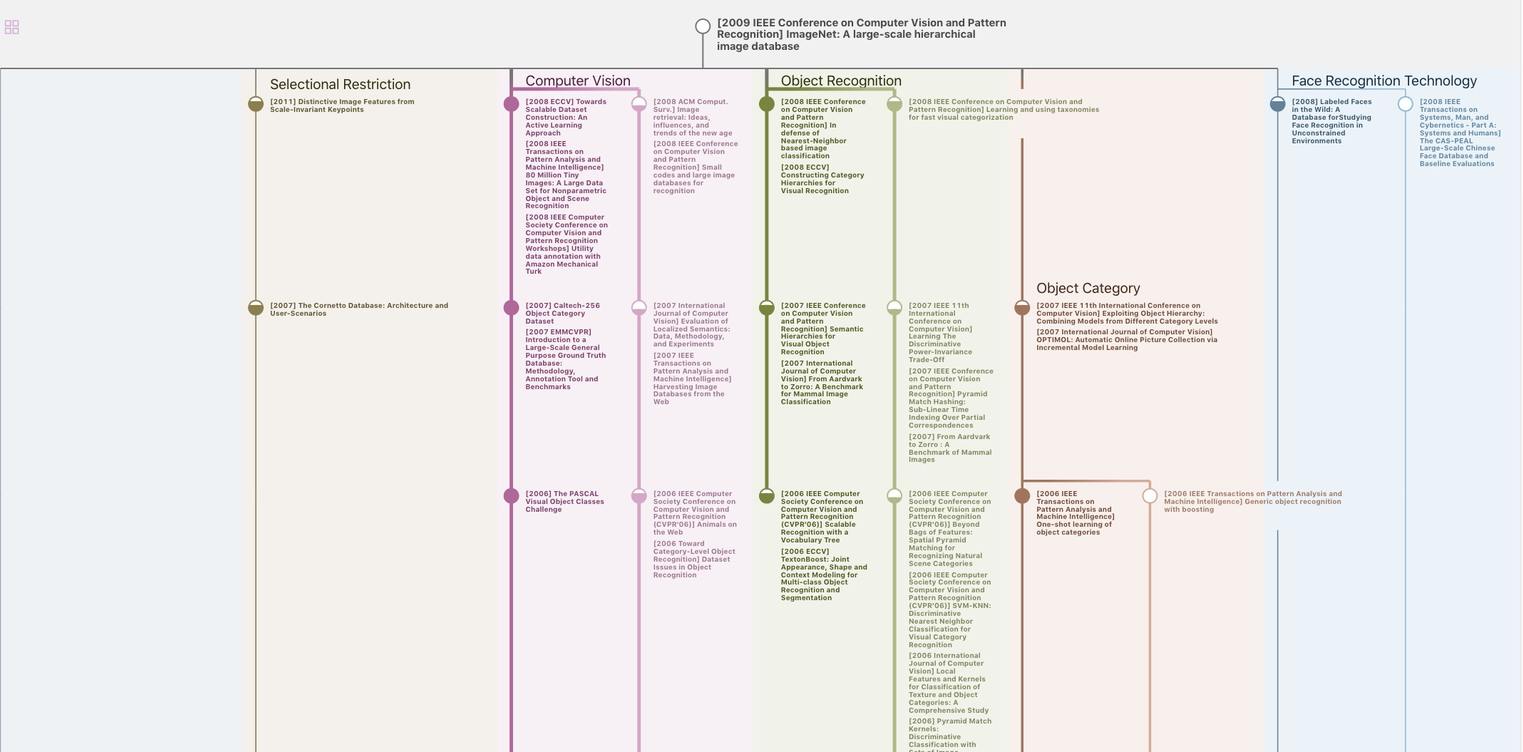
生成溯源树,研究论文发展脉络
Chat Paper
正在生成论文摘要