Serendipity-Oriented Clustering Method for Recommender Systems Based on Collaborative Filtering
Advanced Technologies, Systems, and Applications VII(2022)
摘要
Online shopping is becoming more common, and customers need a recommendation from the system for their needs. Providing accurate predictions is of utmost importance for the success of recommender systems. Various factors may affect the quality of the prediction. Besides the quality of prediction, efficiency is also vital for the success of recommender systems. A similarity metric is used to create a neighborhood in a dataset. In this study, we investigated the effects of binary similarity metrics on the accuracy and efficiency of the prediction. Additionally, we compared how the number of neighborhoods impacts the result and the quality of prediction. We conduct various real data-based experiments to determine the optimal number of neighborhoods and the optimal binary similarity measure. Our experimental outcomes show that the Jaccard similarity metric provides the best result when compared to the Ochiai and Kulczynski. These similarity measurements are implemented with a serendipity approach in mind. The Serendipity approach contains novelty, unexpectedness, and relevance components. In this study, we implemented the above-mentioned similarity measurements to calculate the relevance component whereas most of the researchers used cosine and Pearson similarity measurements.
更多查看译文
关键词
Serendipity, Collaborative Filtering, Clustering, Binary similarity measurements, Recommendation systems
AI 理解论文
溯源树
样例
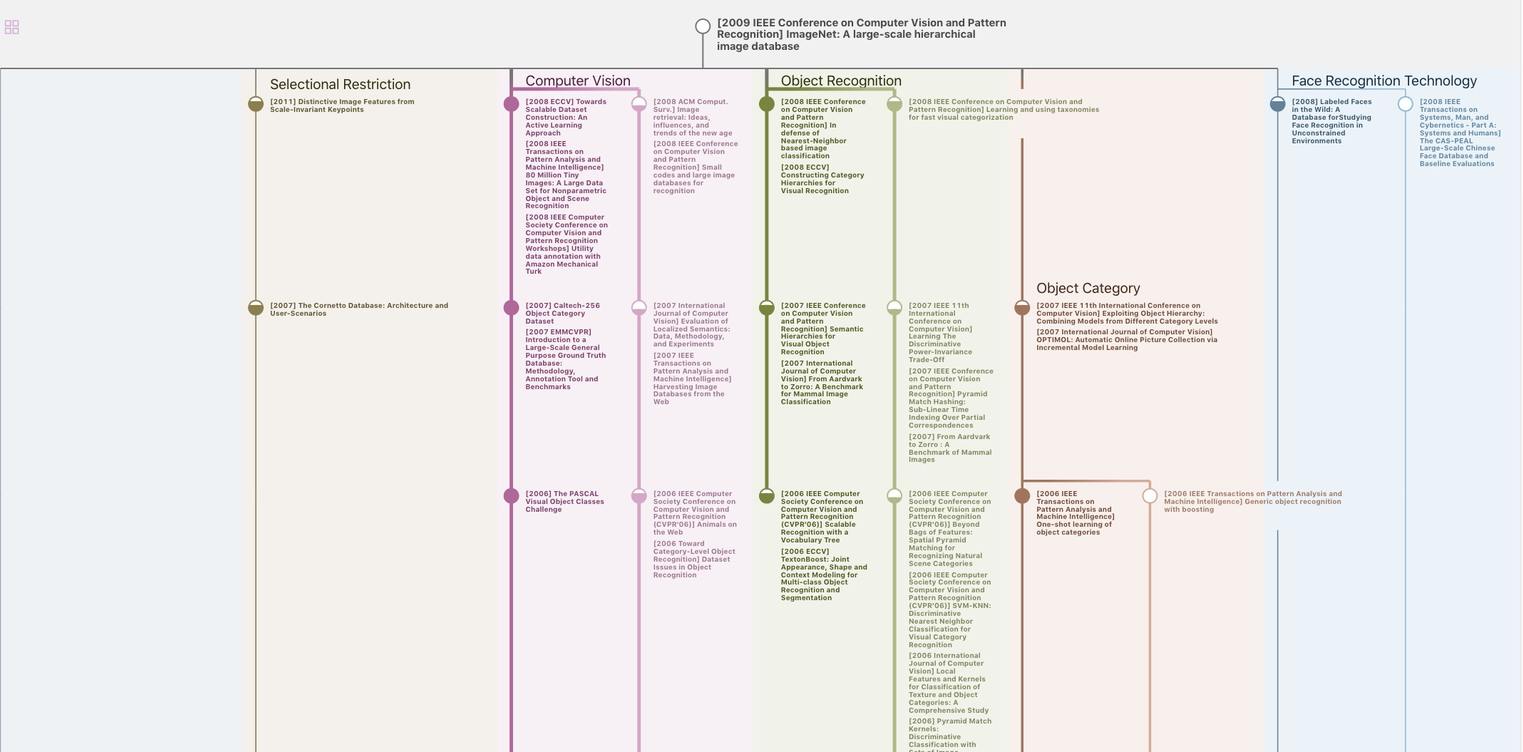
生成溯源树,研究论文发展脉络
Chat Paper
正在生成论文摘要