A Policy-based Approach to the SpecAugment Method for Low Resource E2E ASR
arxiv(2022)
摘要
SpecAugment is a very effective data augmentation method for both HMM and E2E-based automatic speech recognition (ASR) systems. Especially, it also works in low-resource scenarios. However, SpecAugment masks the spectrum of time or the frequency domain in a fixed augmentation policy, which may bring relatively less data diversity to the low-resource ASR. In this paper, we propose a policy-based SpecAugment (Policy-SpecAugment) method to alleviate the above problem. The idea is to use the augmentation-select policy and the augmentation-parameter changing policy to solve the fixed way. These policies are learned based on the loss of validation set, which is applied to the corresponding augmentation policies. It aims to encourage the model to learn more diverse data, which the model relatively requires. In experiments, we evaluate the effectiveness of our approach in low-resource scenarios, i.e., the 100 hours librispeech task. According to the results and analysis, we can see that the above issue can be obviously alleviated using our proposal. In addition, the experimental results show that, compared with the state-of-the-art SpecAugment, the proposed Policy-SpecAugment has a relative WER reduction of more than 10% on the Test/Dev-clean set, more than 5% on the Test/Dev-other set, and an absolute WER reduction of more than 1% on all test sets.
更多查看译文
关键词
augmentation-parameter changing policy,augmentation-select policy,data augmentation,HMM,low resource E2E ASR,low-resource automatic speech recognition,low-resource ASR,policy-based SpecAugment method,Policy-SpecAugment
AI 理解论文
溯源树
样例
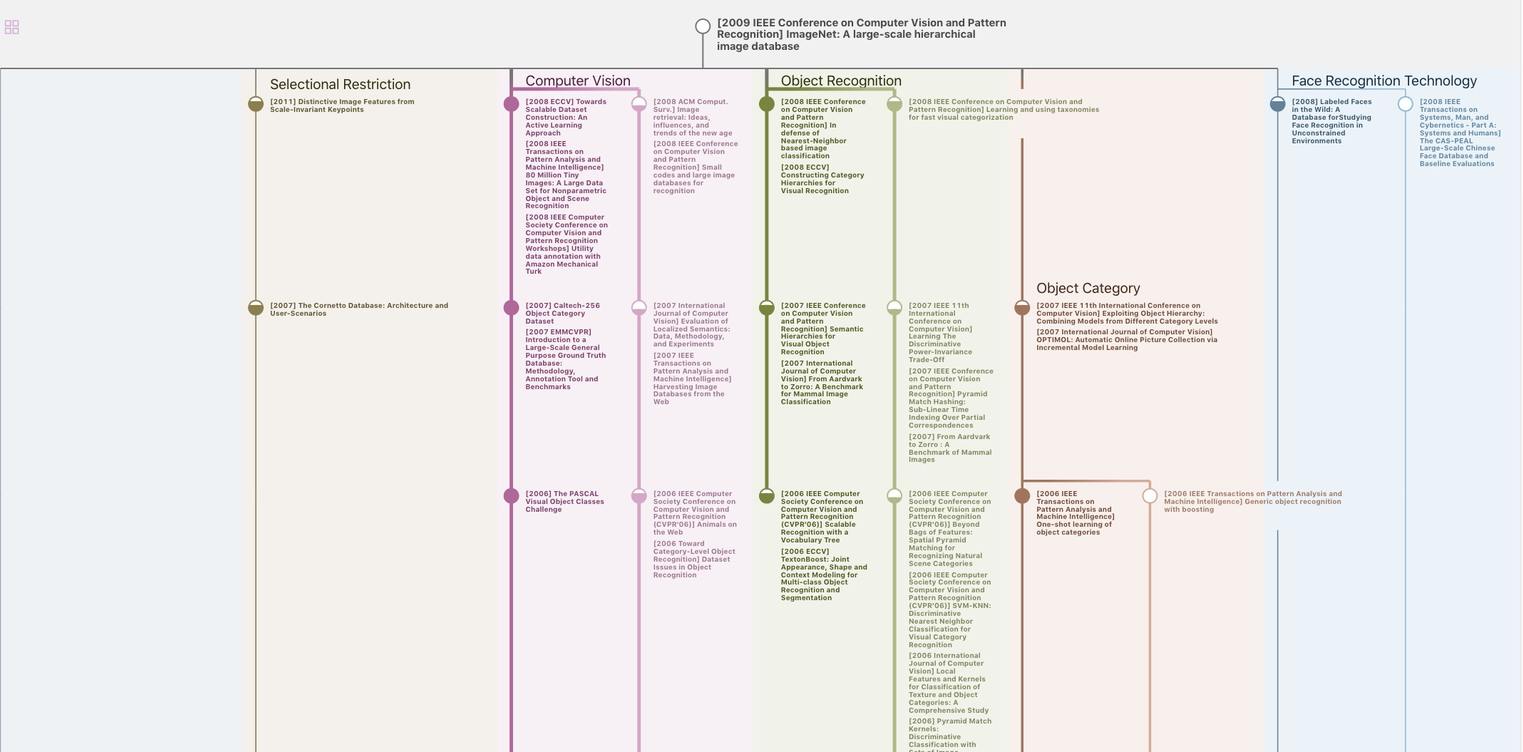
生成溯源树,研究论文发展脉络
Chat Paper
正在生成论文摘要