Feature disentanglement and tendency retainment with domain adaptation for Lithium-ion battery capacity estimation.
Reliab. Eng. Syst. Saf.(2023)
摘要
Online capacity estimation of lithium-ion batteries plays an important role in battery management systems. Accurate estimation of the current capacity of the battery is helpful for predictive maintenance. Data-driven capacity estimation methods have become increasingly popular in recent years. However, most data-driven approaches assume that the battery degradation data used to train and test obeys the same distribution. In practical applications, there is often a discrepancy in the distributions of the training set and the test set due to differences in the internal chemistry of batteries and various operating conditions. The existing methods for domain adaptive capacity estimation force the global features of the source and target domains to be aligned, but do not take into account the domain-specific information. Thus, models may not extract domain-invariant representation. To address this problem, We propose a feature Disentanglement and tendency Retainment network (DR-Net) for domain adaptive capacity estimation. Specifically, our DR-Net can disentangle the extracted features from raw data into domain-invariant shared features and domain-specific private features while retaining degradation trend information. Experimental results show that the proposed method achieves more competitive results in both estimation accuracy and robustness than other state-of-the-art methods. Our code is available at: https://github.com/wang-fujin/battery_capacity_estimation.
更多查看译文
关键词
domain adaptation,capacity estimation,tendency retainment,feature,lithium-ion
AI 理解论文
溯源树
样例
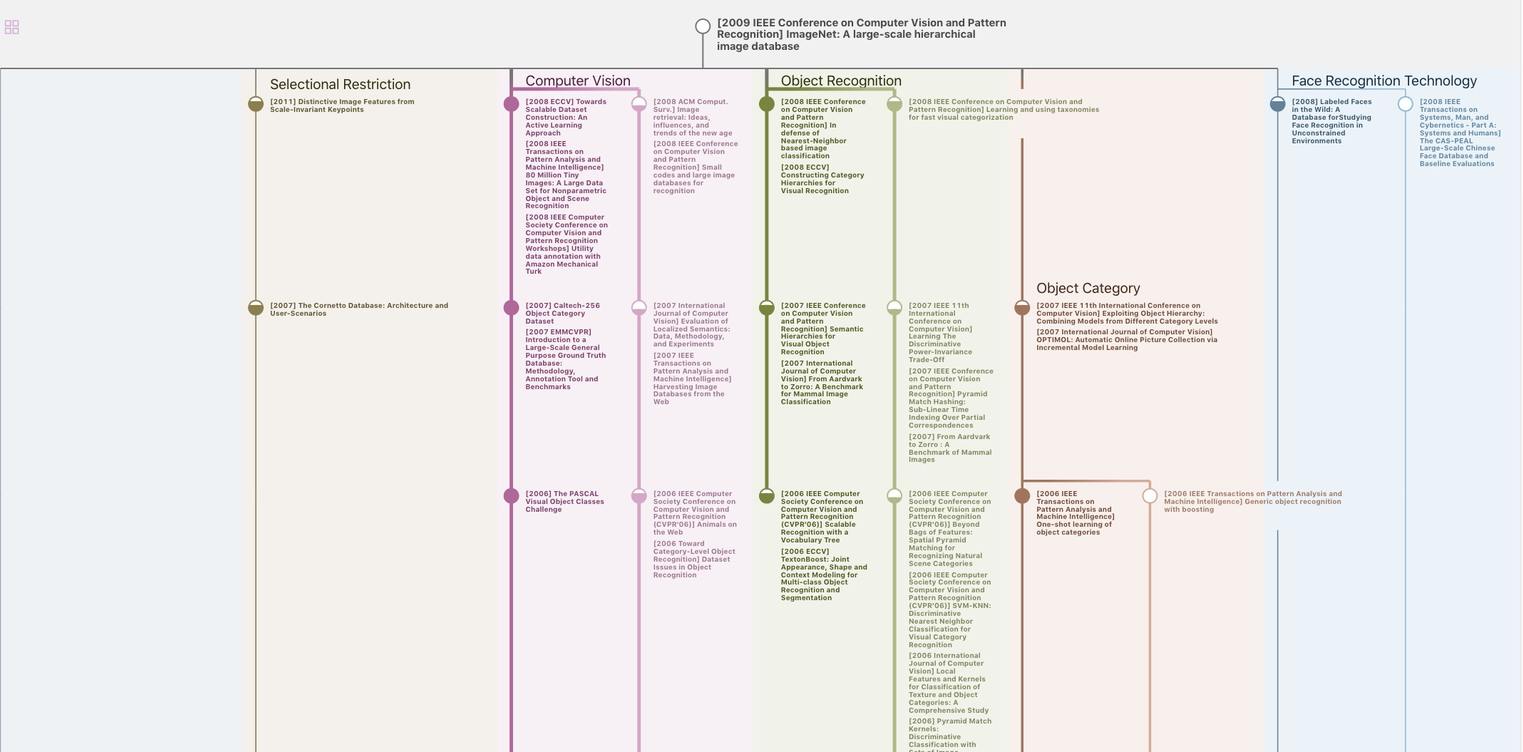
生成溯源树,研究论文发展脉络
Chat Paper
正在生成论文摘要