Lifelong Online Learning from Accumulated Knowledge
ACM Transactions on Knowledge Discovery from Data(2022)
摘要
In this paper, we formulate lifelong learning as an online transfer learning procedure over consecutive tasks, where learning a given task depends on the accumulated knowledge. We propose a novel theoretical principled framework, lifelong online learning, where the learning process for each task is in an incremental manner. Specifically, our framework is composed of two-level predictions: the prediction information that solely from current task; and the prediction from knowledge base by previous tasks. Moreover, this paper tackled several fundamental challenges: arbitrary or even non-stationary task generation process, unknown number of instances in each task, and constructing an efficient accumulated knowledge base. Notably, we provide a provable bound of the proposed algorithm, which offers insights on the how the accumulated knowledge improves the predictions. Finally, empirical evaluations on both synthetic and real datasets validate the effectiveness of proposed algorithm.
更多查看译文
关键词
Online learning,lifelong learning theory,transfer learning,multi-task learning
AI 理解论文
溯源树
样例
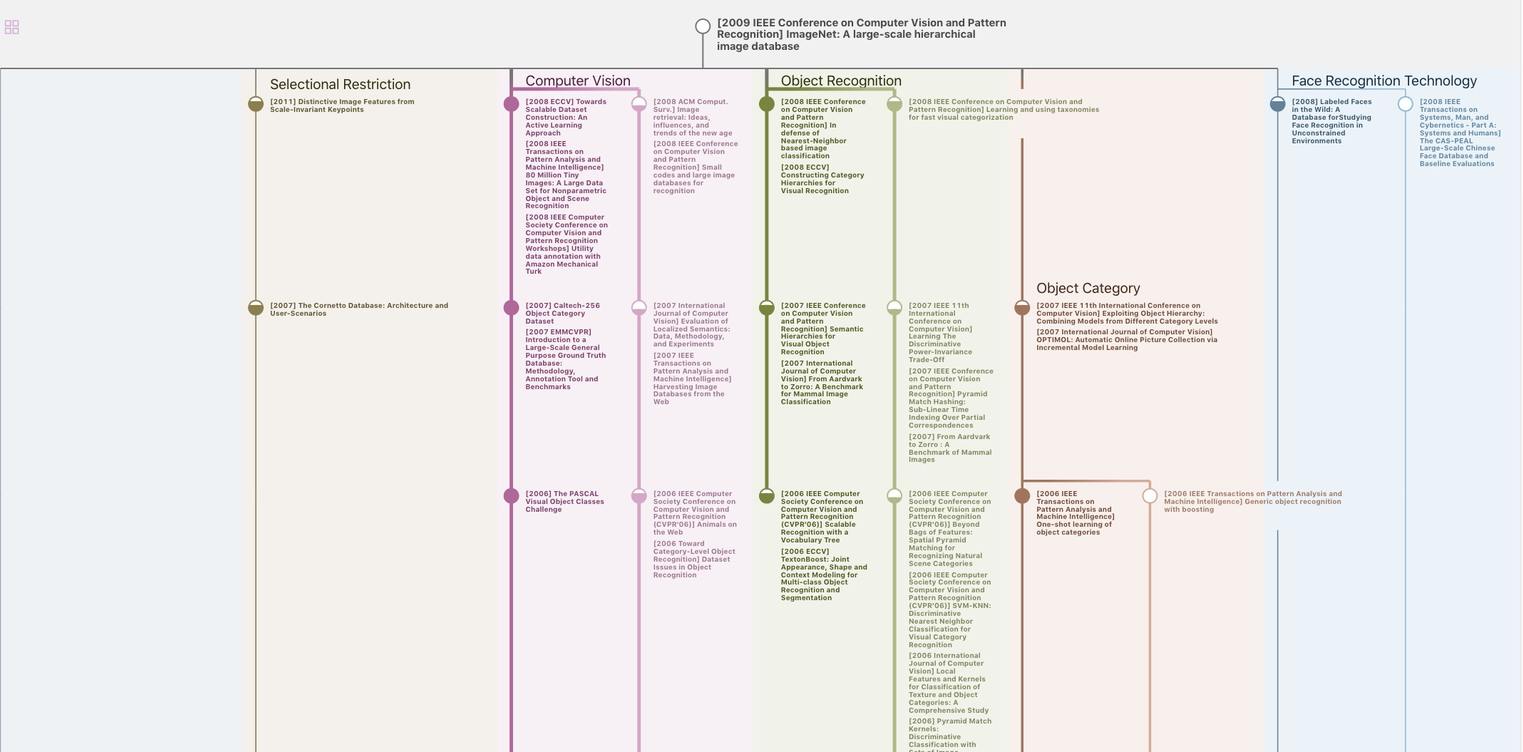
生成溯源树,研究论文发展脉络
Chat Paper
正在生成论文摘要