The Semantic Processing Pipeline: Quantifying the Network-Wide Impact of Security Tools
2020 Workshop in DYnamic and Novel Advances in Machine Learning and Intelligent Cyber Security(2020)
摘要
We present the Semantic Processing Pipeline (SPP), a component of the larger process of our Uncertainty Handling Workflow [10]. The SPP is a configurable, customizable plugin framework for computing network-wide impact of security tools. In addition, it can be used as a labeled data generation mechanism for leveraging machine learning based security techniques. The SPP takes cyber range experiment results as input, quantifies the tool impact, and produces a connected graph encoding knowledge derived from the experiment. This is then used as input into a quantification mechanism of our choice, be it machine learning algorithms or a Multi-Entity Bayesian Network, as in our current implementation. We quantify the level of uncertainty with respect to five key metrics, which we have termed Derived Attributes: Speed, Success, Detectability, Attribution, and Collateral Damage. We present results from experiments quantifying the effect of Nmap, a host and service discovery tool, configured in various ways. While we use Nmap as an example use case, we demonstrate that the SPP easily be applied to various tool types. In addition, we present results regarding performance and correctness of the SPP. We present runtimes for individual components as well as overall, and show that the processing time for the SPP scales quadratically with increasing input sizes. However, the overall runtime is low: the SPP can compute a connected graph from a 200-host topology in roughly one minute.
更多查看译文
AI 理解论文
溯源树
样例
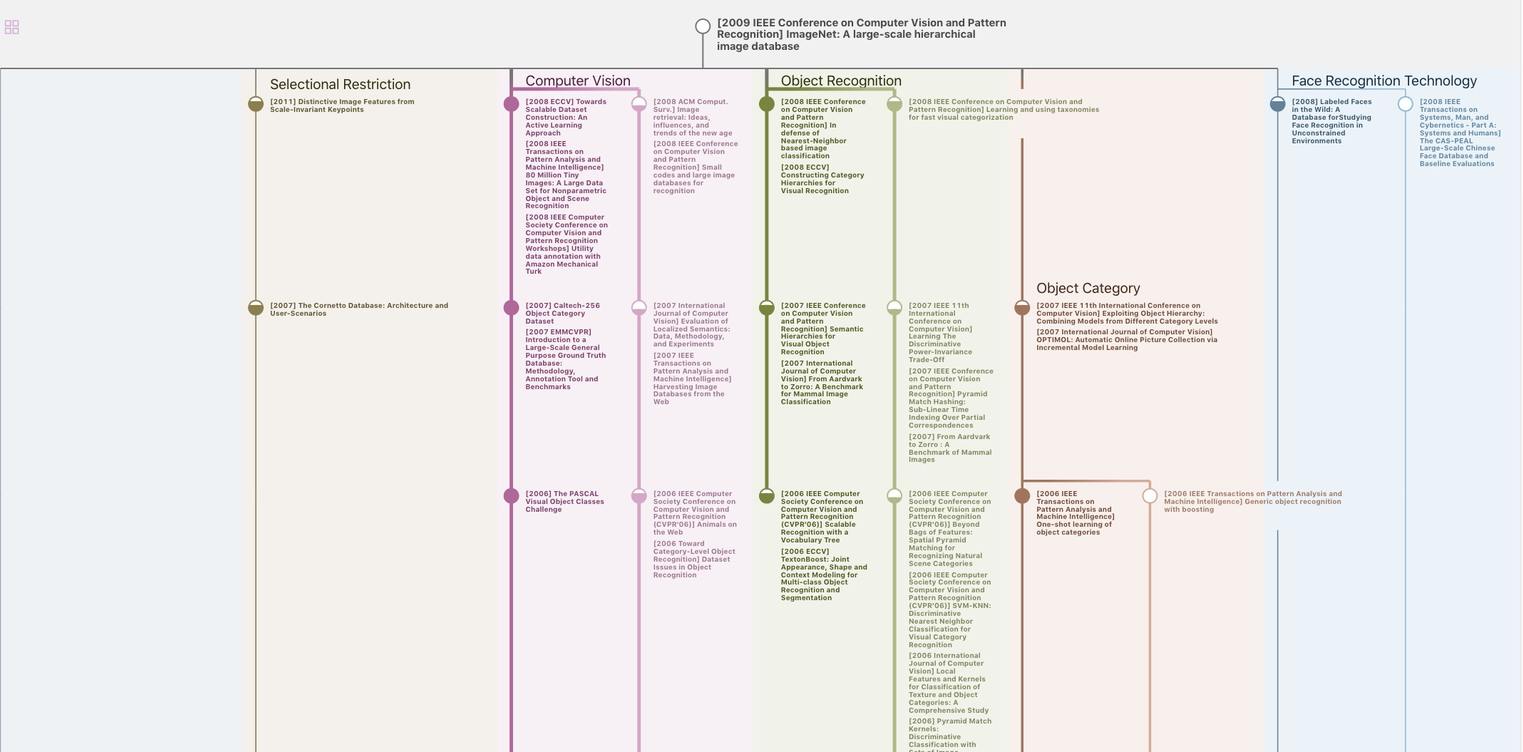
生成溯源树,研究论文发展脉络
Chat Paper
正在生成论文摘要