CL-ETC: A Contrastive Learning Method for Encrypted Traffic Classification
2022 IFIP Networking Conference (IFIP Networking)(2022)
摘要
In the fields of network management and cyber security, encrypted network traffic classification is a critical task. Although Deep Learning (DL) models have been used in this field, they lack explicit control over data feature extraction, resulting in the retention of low-value features, which confuses the training and negatively impacts the classification performance. In this paper, we design a Contrastive Learning (CL) based encoder for extracting robust representation vectors with valuable features from unlabeled data. We create multiple augmentation samples for each input data by a unique augmenter. By narrowing representation vectors among similar augmentation samples and alienating them among dissimilar ones, the encoder can capture valuable features. We propose CL-ETC, a semi-supervised method based on the encoder. In CL-ETC, a well-trained encoder can be utilized to guide supervised classifier training to increase classification performance and training speed. We conduct experiments on three datasets, and the findings reveal that CL-ETC outperforms other models in a variety of metrics, including accuracy, precision, recall, F1-score, and classifier training convergence speed.
更多查看译文
关键词
Encrypted traffic classification,Semi-supervised learning,Contrastive learning
AI 理解论文
溯源树
样例
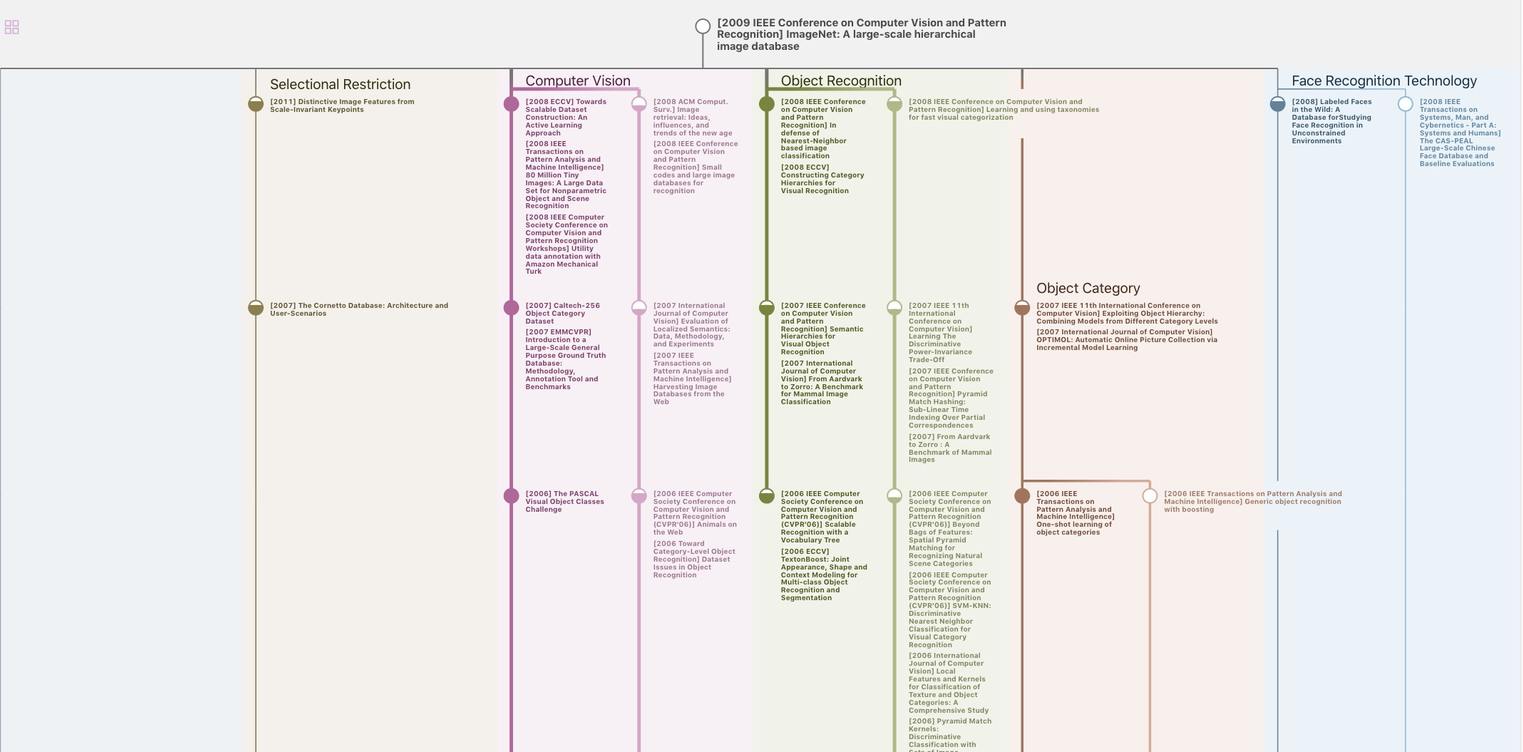
生成溯源树,研究论文发展脉络
Chat Paper
正在生成论文摘要