Deep Neural Network and Transfer Learning for Accurate Hardware-Based Zero-Day Malware Detection
ACM Great Lakes Symposium on VLSI (GLSVLSI)(2022)
摘要
In recent years, security researchers have shifted their attentions to the underlying processors' architecture and proposed Hardware-Based Malware Detection (HMD) countermeasures to address inefficiencies of software-based detection methods. HMD techniques apply standard Machine Learning (ML) algorithms to the processors' low-level events collected from Hardware Performance Counter (HPC) registers. However, despite obtaining promising results for detecting known malware, the challenge of accurate zero-day (unknown) malware detection has remained an unresolved problem in existing HPC-based countermeasures. Our comprehensive analysis shows that standard ML classifiers are not effective in recognizing zero-day malware traces using HPC events. In response, we propose Deep-HMD, a two-stage intelligent and flexible approach based on deep neural network and transfer learning, for accurate zero-day malware detection based on image-based hardware events. The experimental results indicate that our proposed solution outperforms existing ML-based methods by achieving a 97% detection rate (F-Measure and Area Under the Curve) for detecting zero-day malware signatures at run-time using the top 4 hardware events with a minimal false positive rate and no hardware redesign overhead.
更多查看译文
AI 理解论文
溯源树
样例
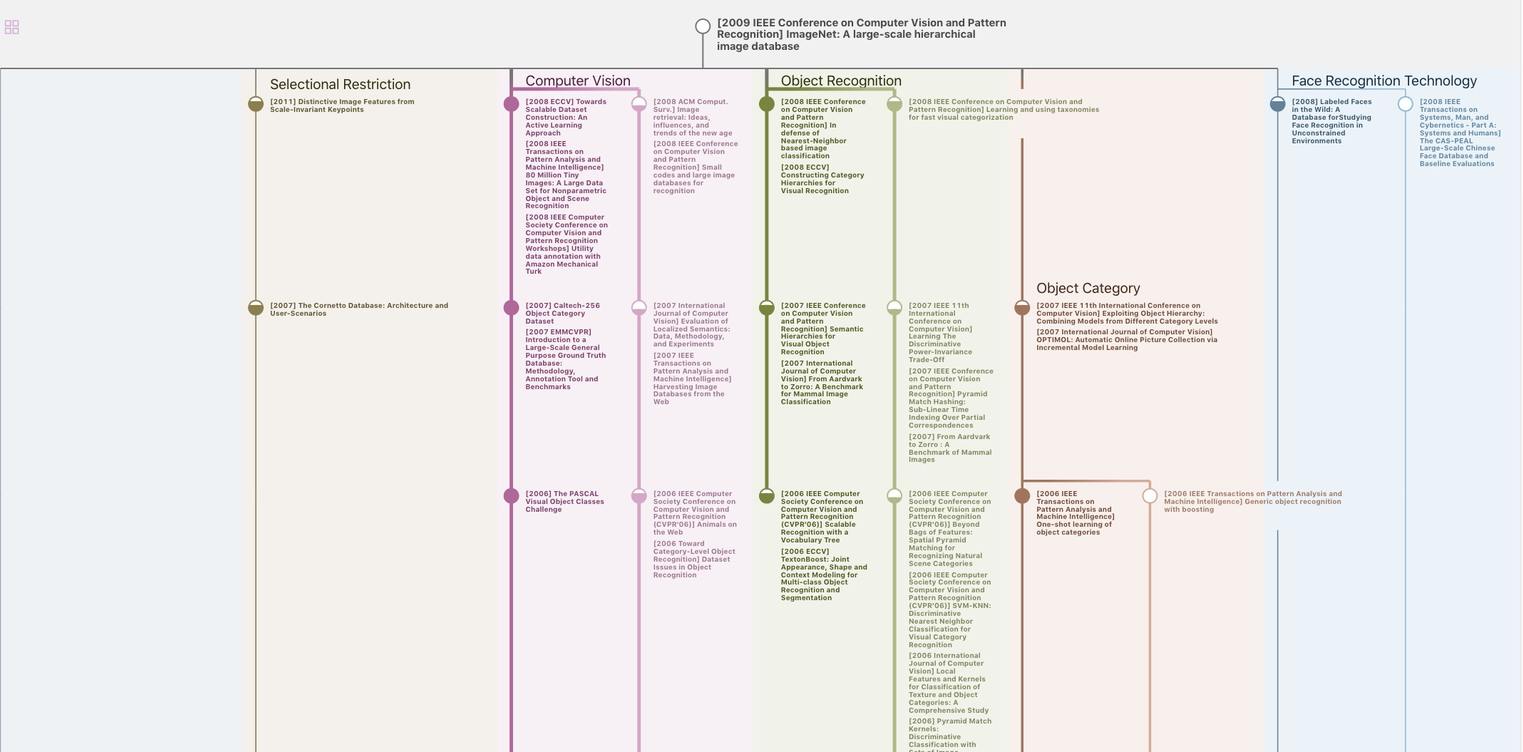
生成溯源树,研究论文发展脉络
Chat Paper
正在生成论文摘要