Can Abnormality be Detected by Graph Neural Networks?
European Conference on Artificial Intelligence (ECAI)(2022)
Abstract
Hypergraphs are natural and expressive modeling tools to encode high-order relationships among entities. Several variations of Hypergraph Neural Networks (HGNNs) are proposed to learn the node representations and complex relationships in the hypergraphs. Most current approaches assume that the input hypergraph structure accurately depicts the relations in the hypergraphs. However, the input hypergraph structure inevitably contains noise, task-irrelevant information, or false-negative connections. Treating the input hypergraph structure as ground-truth information unavoidably leads to sub-optimal performance. In this paper, we propose a Hypergraph Structure Learning (HSL) framework, which optimizes the hypergraph structure and the HGNNs simultaneously in an end-to-end way. HSL learns an informative and concise hypergraph structure that is optimized for downstream tasks. To efficiently learn the hypergraph structure, HSL adopts a two-stage sampling process: hyperedge sampling for pruning redundant hyperedges and incident node sampling for pruning irrelevant incident nodes and discovering potential implicit connections. The consistency between the optimized structure and the original structure is maintained by the intra-hyperedge contrastive learning module. The sampling processes are jointly optimized with HGNNs towards the objective of the downstream tasks. Experiments conducted on 7 datasets show shat HSL outperforms the state-of-the-art baselines while adaptively sparsifying hypergraph structures.
MoreTranslated text
Key words
hypergraph neural networks,structure,learning
AI Read Science
Must-Reading Tree
Example
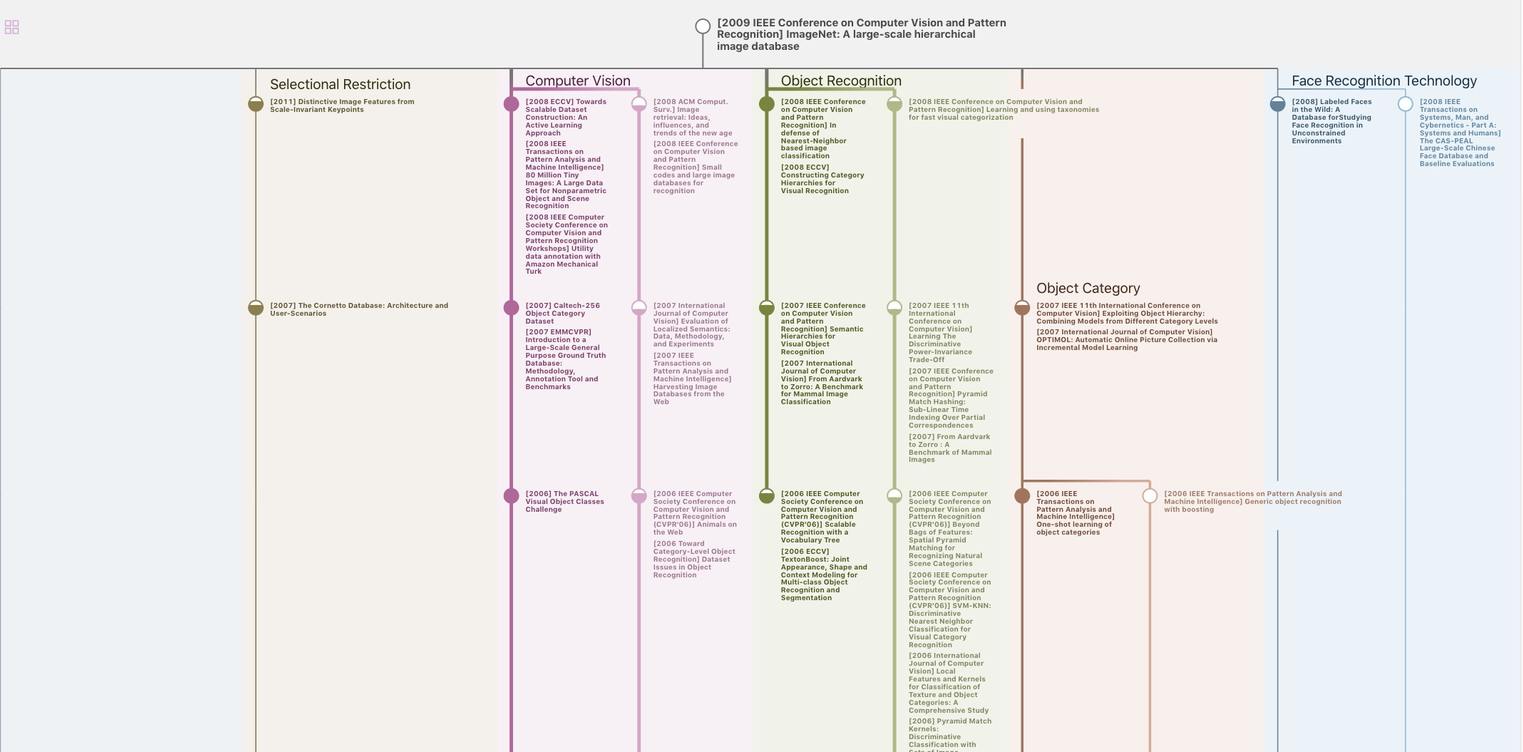
Generate MRT to find the research sequence of this paper
Chat Paper
Summary is being generated by the instructions you defined