Efficient Multiple Kernel Clustering via Spectral Perturbation
International Multimedia Conference(2022)
摘要
ABSTRACTClustering is a fundamental task in the machine learning and data mining community. Among existing clustering methods, multiple kernel clustering (MKC) has been widely investigated due to its effectiveness to capture non-linear relationships among samples. However, most of the existing MKC methods bear intensive computational complexity in learning an optimal kernel and seeking the final clustering partition. In this paper, based on the spectral perturbation theory, we propose an efficient MKC method that reduces the computational complexity from O(n3) to O(nk2 + k3), with n and k denoting the number of data samples and the number of clusters, respectively. The proposed method recovers the optimal clustering partition from base partitions by maximizing the eigen gaps to approximate the perturbation errors. An equivalent optimization objective function is introduced to obtain base partitions. Furthermore, a kernel weighting scheme is embedded to capture the diversity among multiple kernels. Finally, the optimal partition, base partitions, and kernel weights are jointly learned in a unified framework. An efficient alternate iterative optimization algorithm is designed to solve the resultant optimization problem. Experimental results on various benchmark datasets demonstrate the superiority of the proposed method when compared to other state-of-the-art ones in terms of both clustering efficacy and efficiency.
更多查看译文
关键词
spectral perturbation
AI 理解论文
溯源树
样例
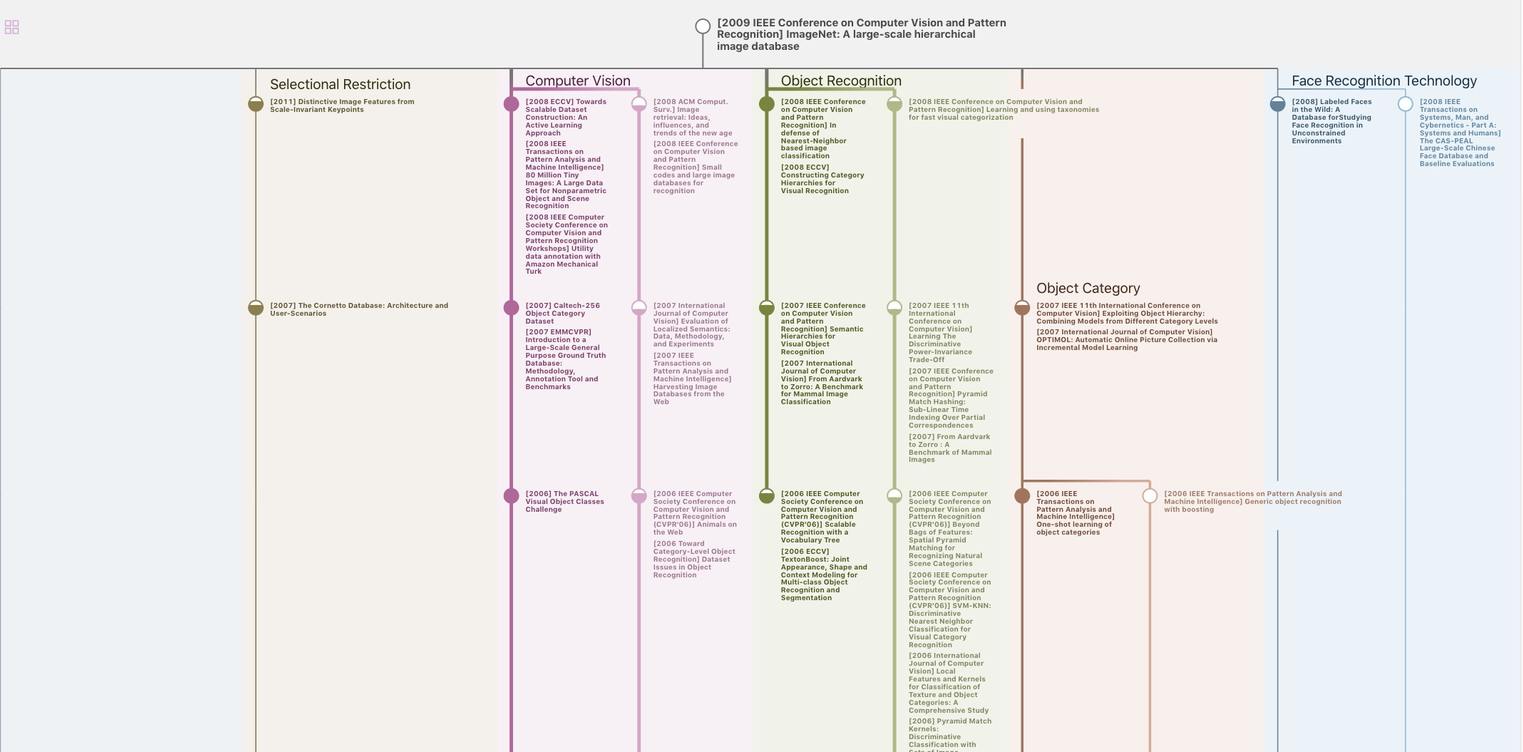
生成溯源树,研究论文发展脉络
Chat Paper
正在生成论文摘要