Semantically-Consistent Dynamic Blurry Image Generation for Image Deblurring
International Multimedia Conference(2022)
摘要
ABSTRACTThe training of deep learning-based image deblurring models heavily relies on the paired sharp/blurry image dataset. Although many works verified that synthesized blurry-sharp pairs contribute to improving the deblurring performance, it is still an open problem about how to synthesize realistic and diverse dynamic blurry images. Instead of directly synthesizing blurry images, in this paper, we propose a novel method to generate semantic-aware dense dynamic motion, and employ the generated motion to synthesize blurry images. Specifically, for each sharp image, both the global motion (camera shake) and local motion (object moving) are considered given the depth information as the condition. Then, a blur creation module takes the spatial-variant motion information and the sharp image as input to synthesize a motion-blurred image. A relativistic GAN loss is employed to assure the synthesized blurry image is as realistic as possible. Experiments show that our method can generate diverse dynamic motion and visually realistic blurry images. Also, the generated image pairs can further improve the quantitative performance and generalization ability of the existing deblurring method on several test sets.
更多查看译文
关键词
semantically-consistent
AI 理解论文
溯源树
样例
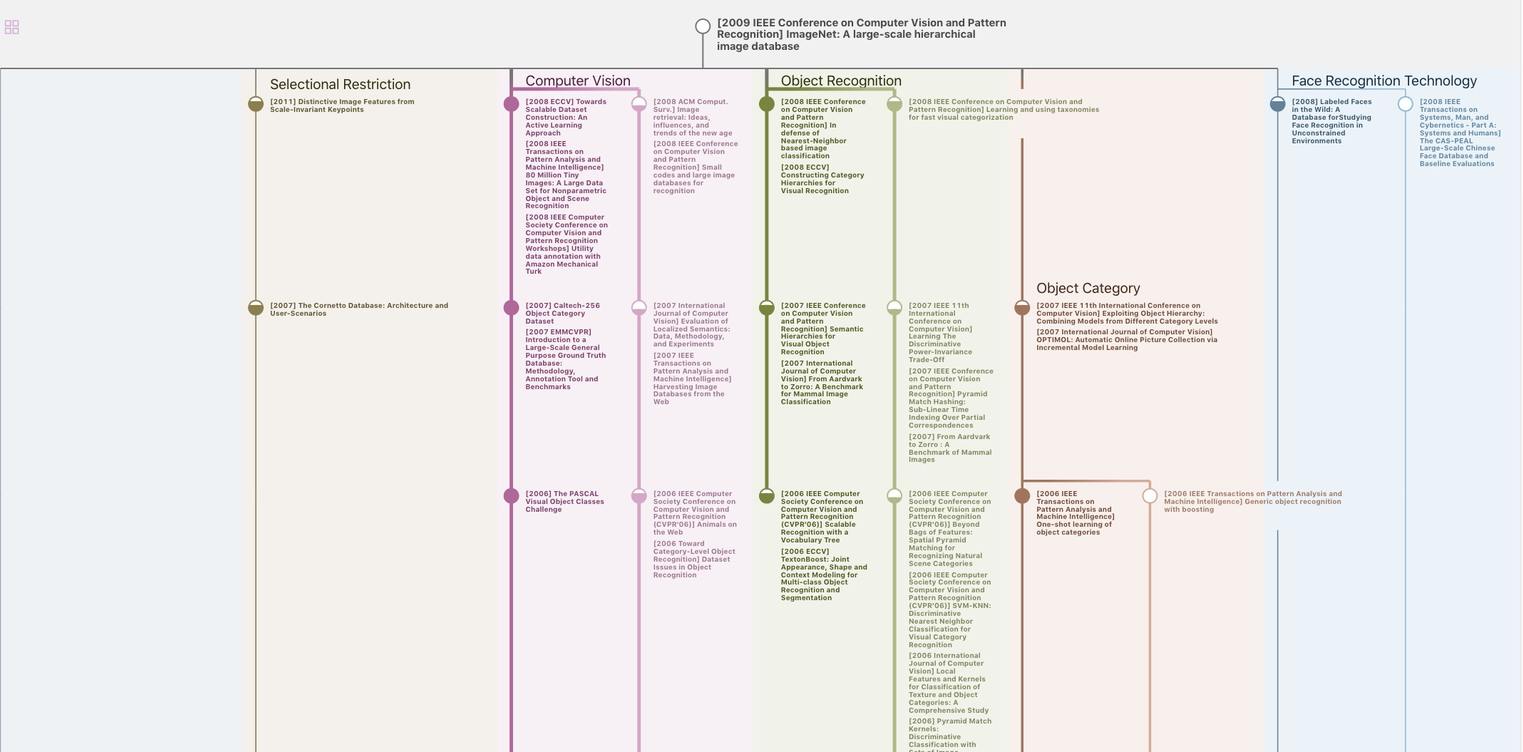
生成溯源树,研究论文发展脉络
Chat Paper
正在生成论文摘要