Bipolar vector classifier for fault-tolerant deep neural networks
Design Automation Conference (DAC)(2022)
摘要
Deep Neural Networks (DNNs) surpass the human-level performance on specific tasks. The outperforming capability accelerate an adoption of DNNs to safety-critical applications such as autonomous vehicles and medical diagnosis. Millions of parameters in DNN requires a high memory capacity. A process technology scaling allows increasing memory density, however, the memory reliability confronts significant reliability issues causing errors in the memory. This can make stored weights in memory erroneous. Studies show that the erroneous weights can cause a significant accuracy loss. This motivates research on fault-tolerant DNN architectures. Despite of these efforts, DNNs are still vulnerable to errors, especially error in DNN classifier. In the worst case, because a classifier in convolutional neural network (CNN) is the last stage determining an input class, a single error in the classifier can cause a significant accuracy drop. To enhance the fault tolerance in CNN, this paper proposes a novel bipolar vector classifier which can be easily integrated with any CNN structures and can be incorporated with other fault tolerance approaches. Experimental results show that the proposed method stably maintains an accuracy with a high bit error rate up to 10(-3) in the classifier.
更多查看译文
关键词
Deep neural networks, Fault-tolerance, Classifier
AI 理解论文
溯源树
样例
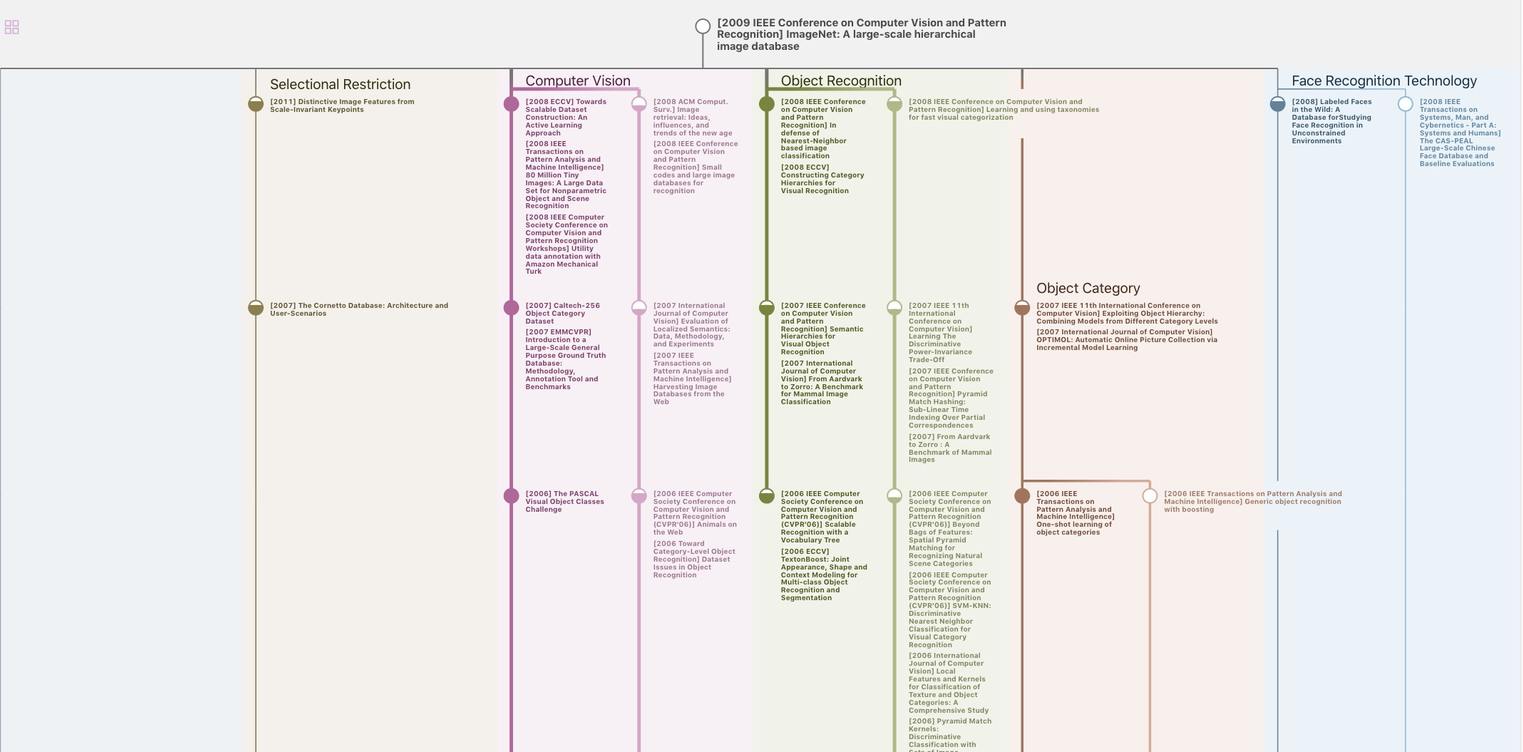
生成溯源树,研究论文发展脉络
Chat Paper
正在生成论文摘要