Probabilistic Framework of Howard's Policy Iteration: BML Evaluation and Robust Convergence Analysis
arxiv(2022)
摘要
This paper aims to build a probabilistic framework for Howard's policy iteration algorithm using the language of forward-backward stochastic differential equations (FBSDEs). As opposed to conventional formulations based on partial differential equations, our FBSDE-based formulation can be easily implemented by optimizing criteria over sample data, and is therefore less sensitive to the state dimension. In particular, both on-policy and off-policy evaluation methods are discussed by constructing different FBSDEs. The backward-measurability-loss (BML) criterion is then proposed for solving these equations. By choosing specific weight functions in the proposed criterion, we can recover the popular Deep BSDE method or the martingale approach for BSDEs. The convergence results are established under both ideal and practical conditions, depending on whether the optimization criteria are decreased to zero. In the ideal case, we prove that the policy sequences produced by proposed FBSDE-based algorithms and the standard policy iteration have the same performance, and thus have the same convergence rate. In the practical case, the proposed algorithm is still proved to converge robustly under mild assumptions on optimization errors.
更多查看译文
关键词
forward-backward stochastic differential equations,policy iteration,stochastic optimal control
AI 理解论文
溯源树
样例
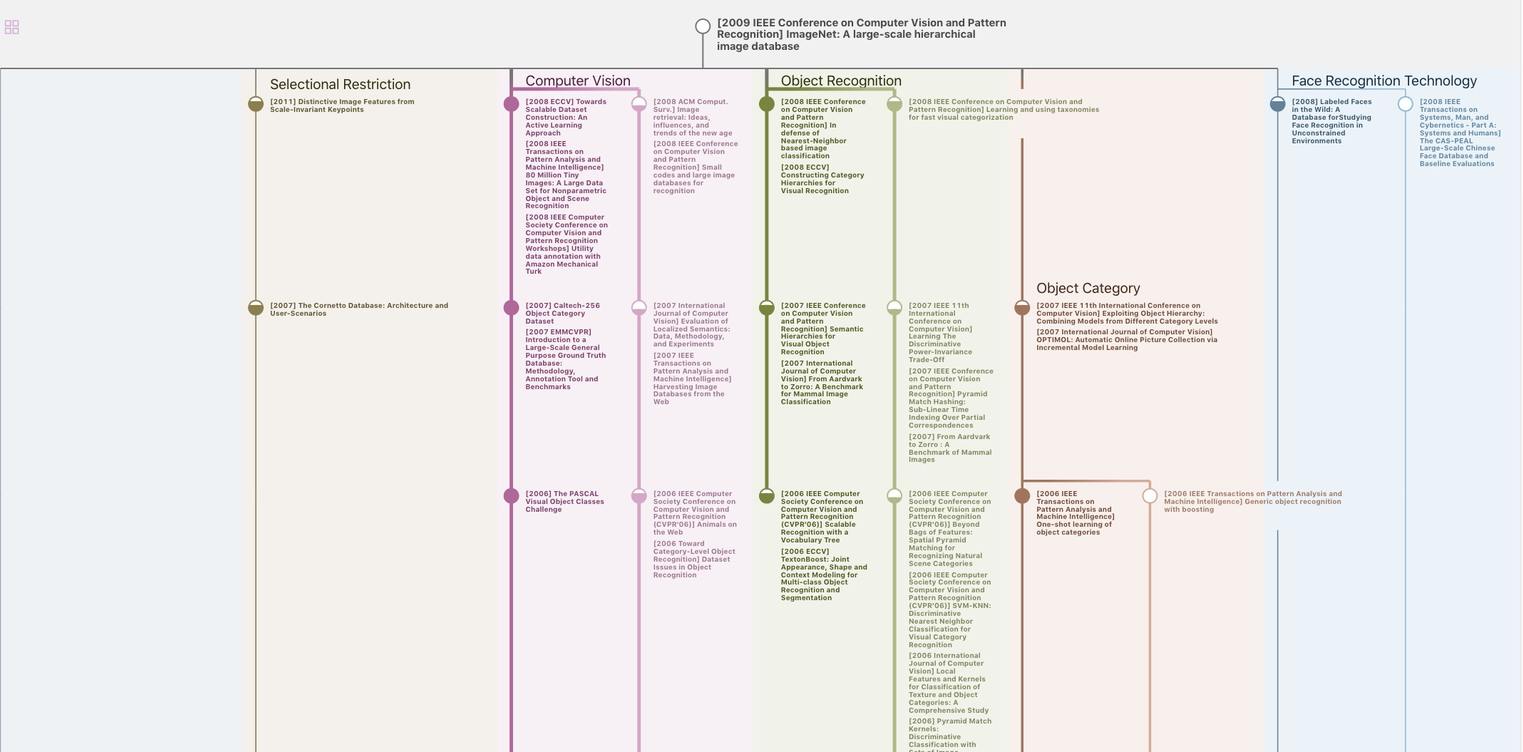
生成溯源树,研究论文发展脉络
Chat Paper
正在生成论文摘要