Fix Me If You Can: Using Neural Networks to Regenerate Networked Systems’ Monitoring Traces
NOMS 2022-2022 IEEE/IFIP Network Operations and Management Symposium(2022)
摘要
Monitoring principal entities in distributed systems is paramount to understanding the behavior of such systems and replicating their dynamics, e.g., for offline analysis or simulated evaluation. Examples of principal entities include users of distributed applications or online hosts on the internet. In many systems, monitoring can be done via periodic sampling of the entities online at a given instant. However, the monitoring process may be flawed. Some entities may fail to appear in one or more samples even though online when those samples were captured, thus compromising the quality of the monitoring traces. Previous investigations have applied statistical methods to identify such failures and used thresholds to correct them. In this paper, we turn our attention to machine learning methods as a means to regenerate monitoring traces collected via sampling. We propose a deep learning procedural method and two neural network topologies for correcting traces. We provide evidence that precision, accuracy, and recall can be substantially improved compared to existing statistical methods, thus opening an entire research avenue for improving the quality of monitoring traces of live distributed systems.
更多查看译文
关键词
periodic sampling,statistical methods,machine learning,deep learning,neural network topologies,distributed systems,networked systems monitoring,principal entities monitoring,Internet
AI 理解论文
溯源树
样例
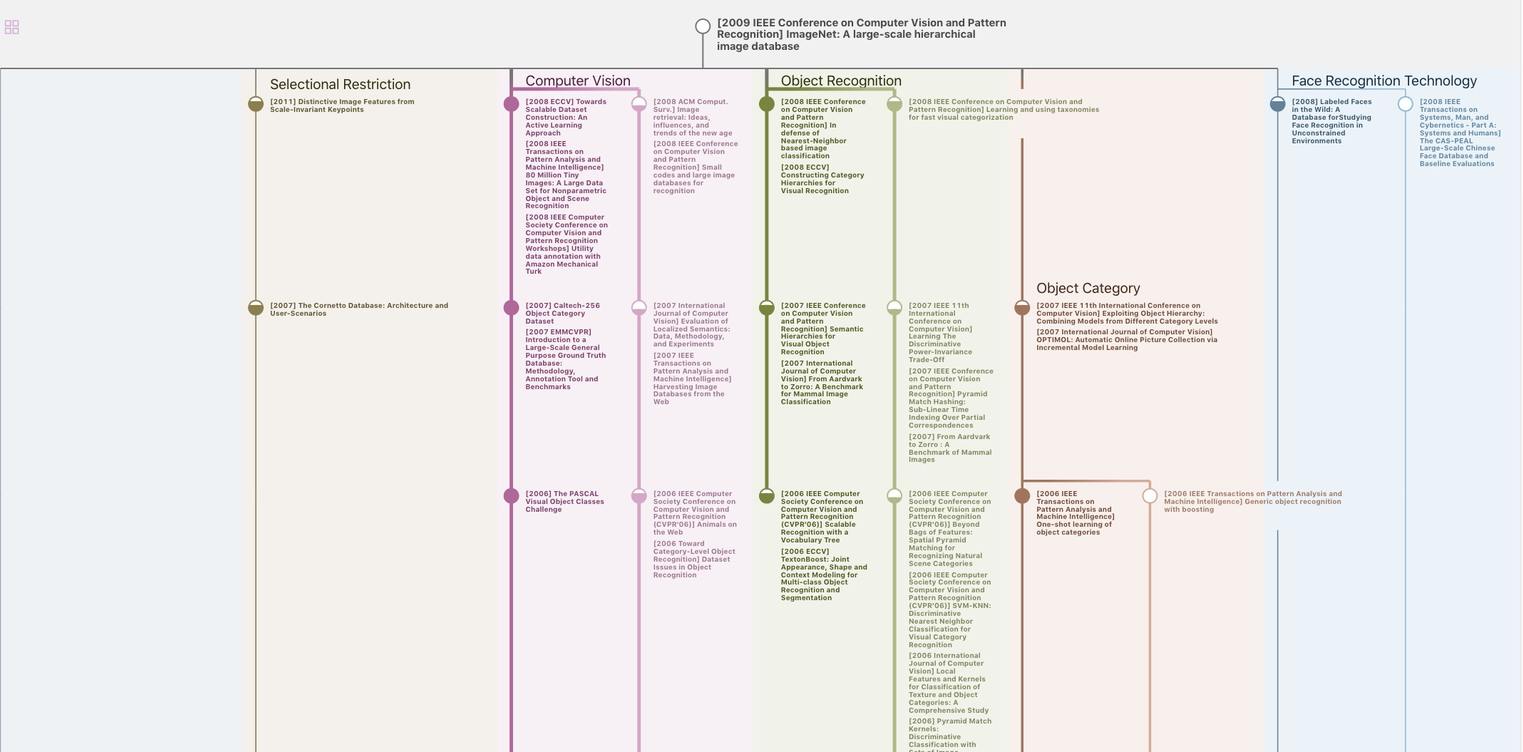
生成溯源树,研究论文发展脉络
Chat Paper
正在生成论文摘要