Cloud identification in Mars daily global maps with deep learning
Icarus(2023)
摘要
Cloud identification on Mars is an important tool for climatology studies, making it possible to analyze the distribution, patterns and variability of clouds both spatially and temporally. Traditionally, cloud data on Mars has been extracted through manual or semi-automated processes which can be time consuming, and currently there is limited spatial and temporal cloud data coverage. In this paper we demonstrate the successful use of convolutional neural networks (CNNs) to extract cloud masks from Mars Daily Global Maps (MDGMs) composed from the Mars Color Imager (MARCI) on the Mars Reconnaissance Orbiter (MRO). The fully automated model reports an F1 score of 0.7925 compared to the prior semi-automatic technique, and in some occasions the model performs better at extracting the full extent of the cloud. We also introduce several image pre- and post-processing techniques to improve the model's performance and usability. The model is configured to provide cloud masks at 0.1° longitude by 0.1° latitude resolution. It also automatically bounds the MDGM by northern and southern polar extents depending on solar longitude. The results suggest that our deep learning model is a useful tool to automatically and quickly extract Martian water ice cloud masks and make it possible to generate cloud mask data across the complete set of MDGMs and future ones. The model and related techniques also have potential extensions to Martian dust storm identification. Our code, model, and data are publicly available.
更多查看译文
关键词
Mars,Atmosphere,Clouds,Machine learning,Deep learning,Mars daily global maps
AI 理解论文
溯源树
样例
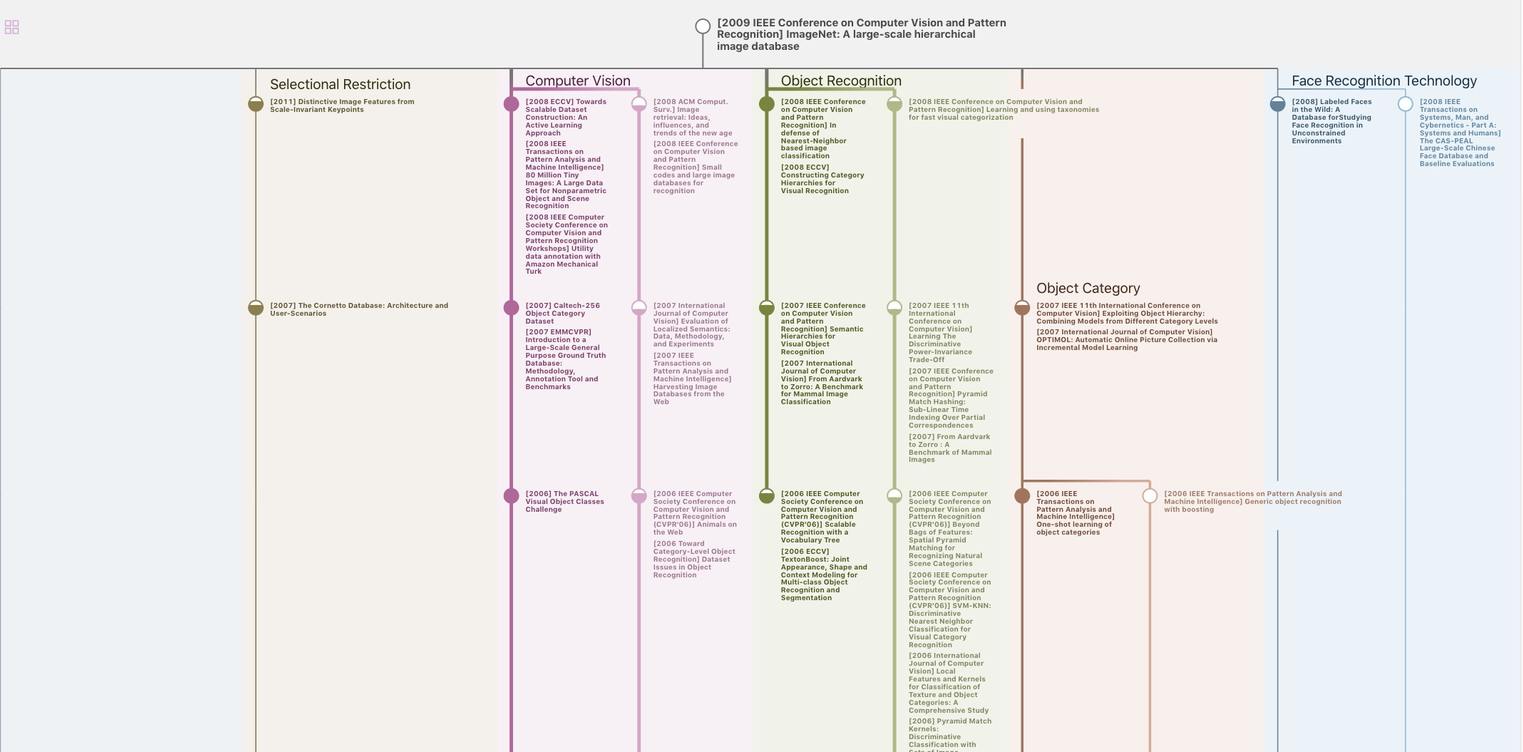
生成溯源树,研究论文发展脉络
Chat Paper
正在生成论文摘要