Classifying Dominant Tree Species Over a Large Mountainous Area Based On Multitemporal Sentinel-2 Data.
IEEE International Conference on High Performance Computing and Communications(2021)
摘要
Trees are vital to the quality of the urban ecological environment. It can improve the quality of air, absorb and reduce carbon dioxide and alleviate urban heat islands. Previous studies that employ multi-spectral imagery have already evaluated different classification algorithms to identify tree species. But often the researches were conducted on small areas and a single image scene. In this study, the study area (Diqing Tibetan Autonomous Prefecture) covered by 73.95% forests, of which approximately 18000 km
2
. Based on the GEE (Google Earth Engine) cloud computing platform, combined multi-temporal Sentinel-2 imagery with topography information, three machine learning classifiers, including Regression Tree, Random Forest, Gradient Boosting Decision Tree, were used to obtain nine tree species 10m meters of mapping products. An overall accuracy of 81.4% (a Cohen's kappa of 78.5%) was obtained for nine tree species classes. The addition of multi-temporal images improved the OA (over all accuracy) by 8.01%∼12.40%, and Kappa by 8.91%∼12.96%. After combining the terrain features, OA is improved by 9.39%∼14.72%, and Kappa by 10.95%∼18.19%. Obtaining high-quality cloud-free images is difficult for the cloudy and rainy southwest of China, so the composites of multi-temporal images and the combination of other morphoclimatic characteristics are of great significance for large-scale tree species classification.
更多查看译文
关键词
Tree species,Multi-temporal,Google Earth Engine,Machine Learning
AI 理解论文
溯源树
样例
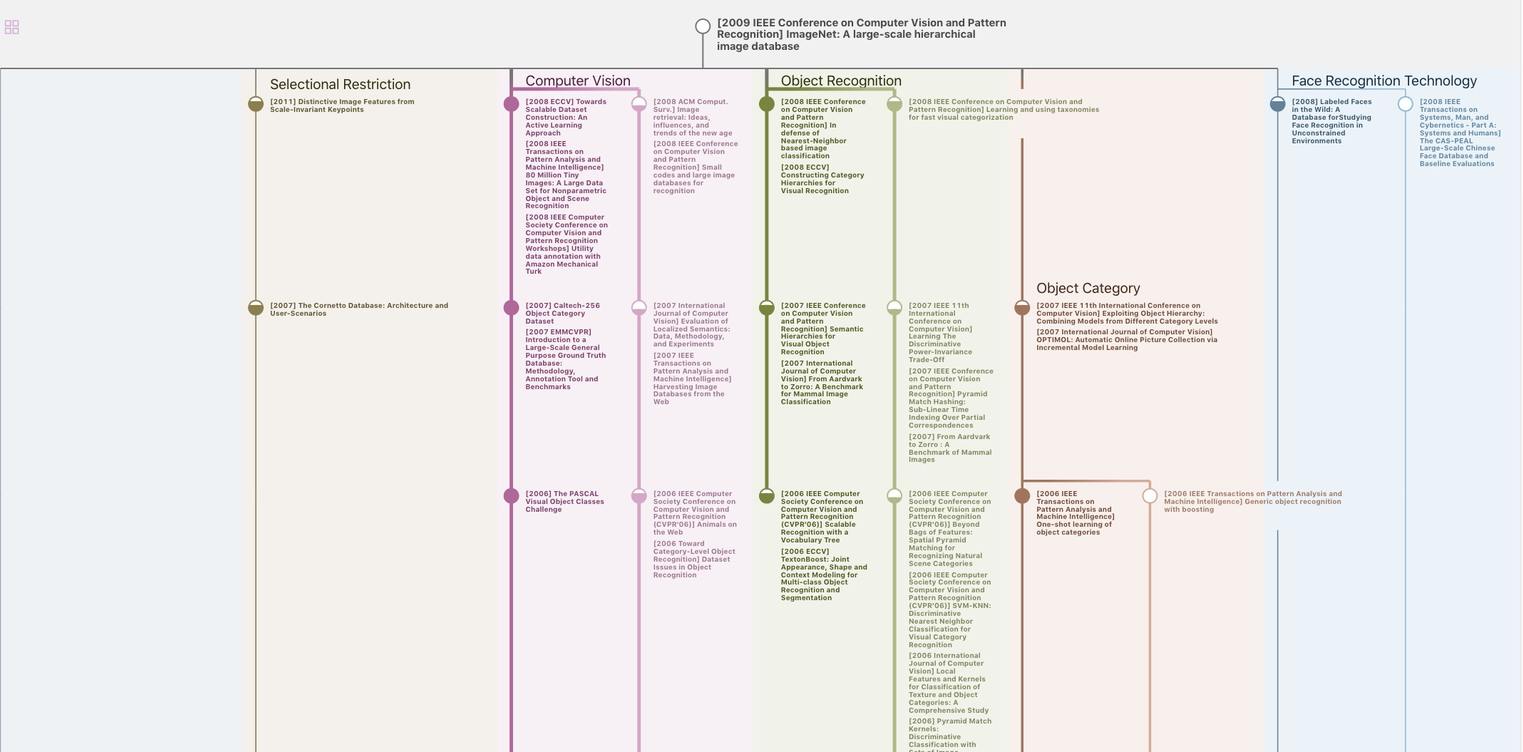
生成溯源树,研究论文发展脉络
Chat Paper
正在生成论文摘要