Accelerating Robot Reinforcement Learning with Samples of Different Simulation Precision
2021 IEEE 23rd Int Conf on High Performance Computing & Communications; 7th Int Conf on Data Science & Systems; 19th Int Conf on Smart City; 7th Int Conf on Dependability in Sensor, Cloud & Big Data Systems & Application (HPCC/DSS/SmartCity/DependSys)(2021)
摘要
Training an effective model via reinforcement learning method in a simulated environment demands considerable computational cost. To improve the efficiency of reinforcement learning, most existing efforts have been focusing on learning algorithms or sampling techniques, but little attention has been spent on better utilizing the simulators themselves. In this paper, we propose a novel method to accelerate the training process by sampling with different simulation precisions in the simulator. Specifically, a sequential training mode and a joint training mode are proposed. In the sequential training mode, a basic model is first learned by using samples from a low-precision simulation environment, and then further fine-tuned in a high-precision simulation environment. In the joint training mode, the training procedure is conducted by sampling from the low and high-precision simulation environments simultaneously. Extensive experiments in different robotic scenarios demonstrate that our method achieves almost the same performance as conventional methods, but requires much less training cost, where 35% to 63% of the training time is saved without loss of performance.
更多查看译文
关键词
accelerate training,reinforcement learning,robot simulator,simulation precision
AI 理解论文
溯源树
样例
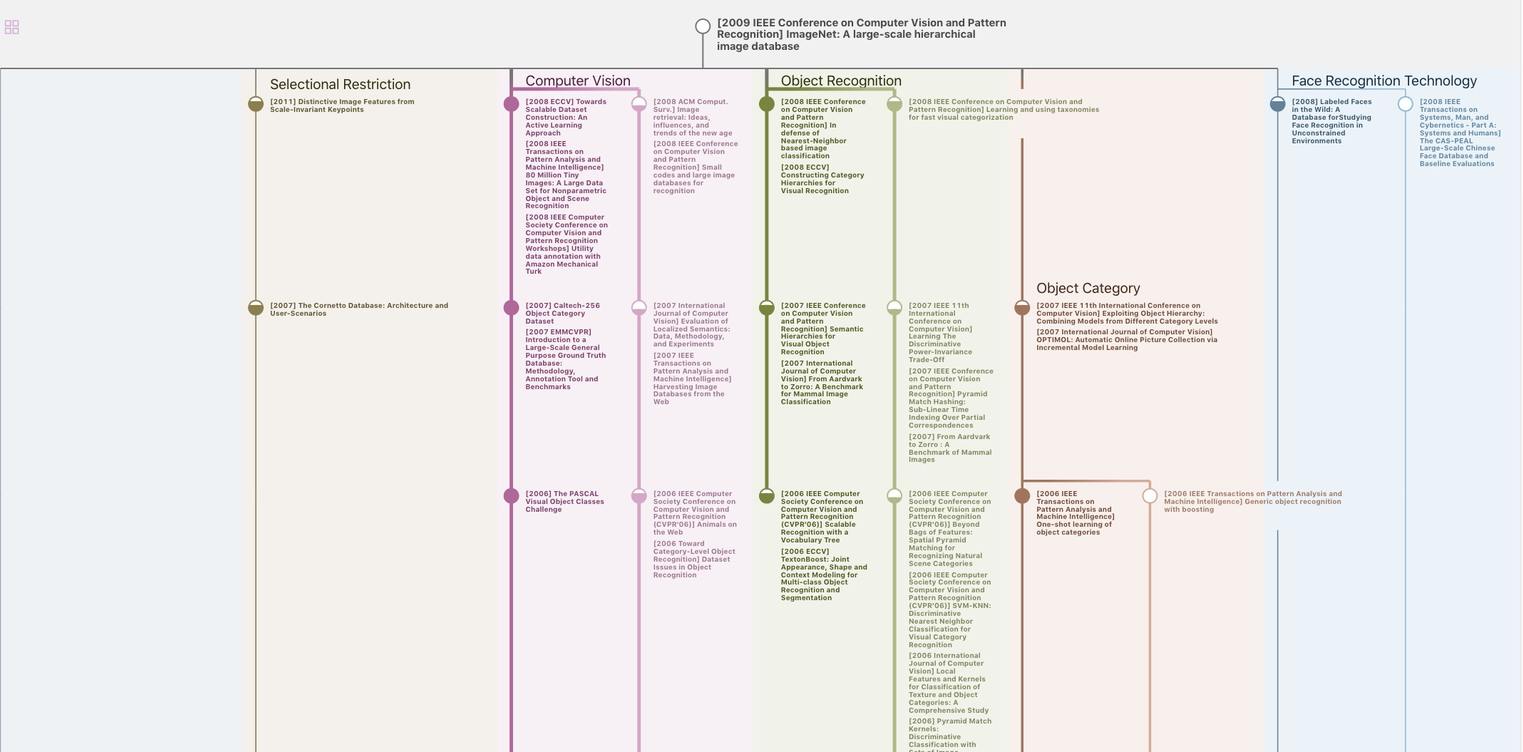
生成溯源树,研究论文发展脉络
Chat Paper
正在生成论文摘要