A soft-sensor for sustainable operation of coagulation and flocculation units
Engineering Applications of Artificial Intelligence(2022)
摘要
Nowadays, Machine Learning (ML) techniques have become one of the most widely used engineering tools due to their numerous advantages, including their continuous improvement. This study proposes a smart soft sensor using various ML algorithms to control and predict the Coagulation and Flocculation Process (CFP). Optimizing and predicting the behaviour of a CFP is difficult due to its non-linear and complex behaviour. Therefore, ML computations is a proper method to overcome this challenge. However, one of the challenges of ML studies is the lack of sufficient data which we overcome by using an 8-year database of experiments. For prediction, this study compares different ML methods, including Random Tree, Random Forest (RF), Artificial Neural Networks (ANN), Quinlan’s M5 algorithm with regression function (M5P), Linear Regression (LR), Simple LR, Gaussian method, Decision Stump method, Smola and Scholkopf’s Sequential Minimal Optimization algorithm with LR (SMOreg), and the Adaptive Neuro-Fuzzy Inference System (ANFIS). Also, for optimization of the studied system, Central Composite Design designed the experimental data with the Response Surface Methodology (CCD-RSM). The most significant factors in turbidity removal are related to FeCl3 dosage, and slow mixing speed with < 0.0001 and 0.005 P-values. The present research findings show that the maximum removal efficiency of 92% is predicted using CCD-RSM under the optimal condition. In addition, ANFIS and RF models with R2 of 0.96 and 0.92, have shown the highest accuracy levels for removing water turbidity. Finally, a Petri-Net model establishes a Conceptual model to intelligently conduct managerial insights for water treatments.
更多查看译文
关键词
Water quality,Coagulation modelling,Turbidity,Machine learning,Petri-net,CCD-RSM
AI 理解论文
溯源树
样例
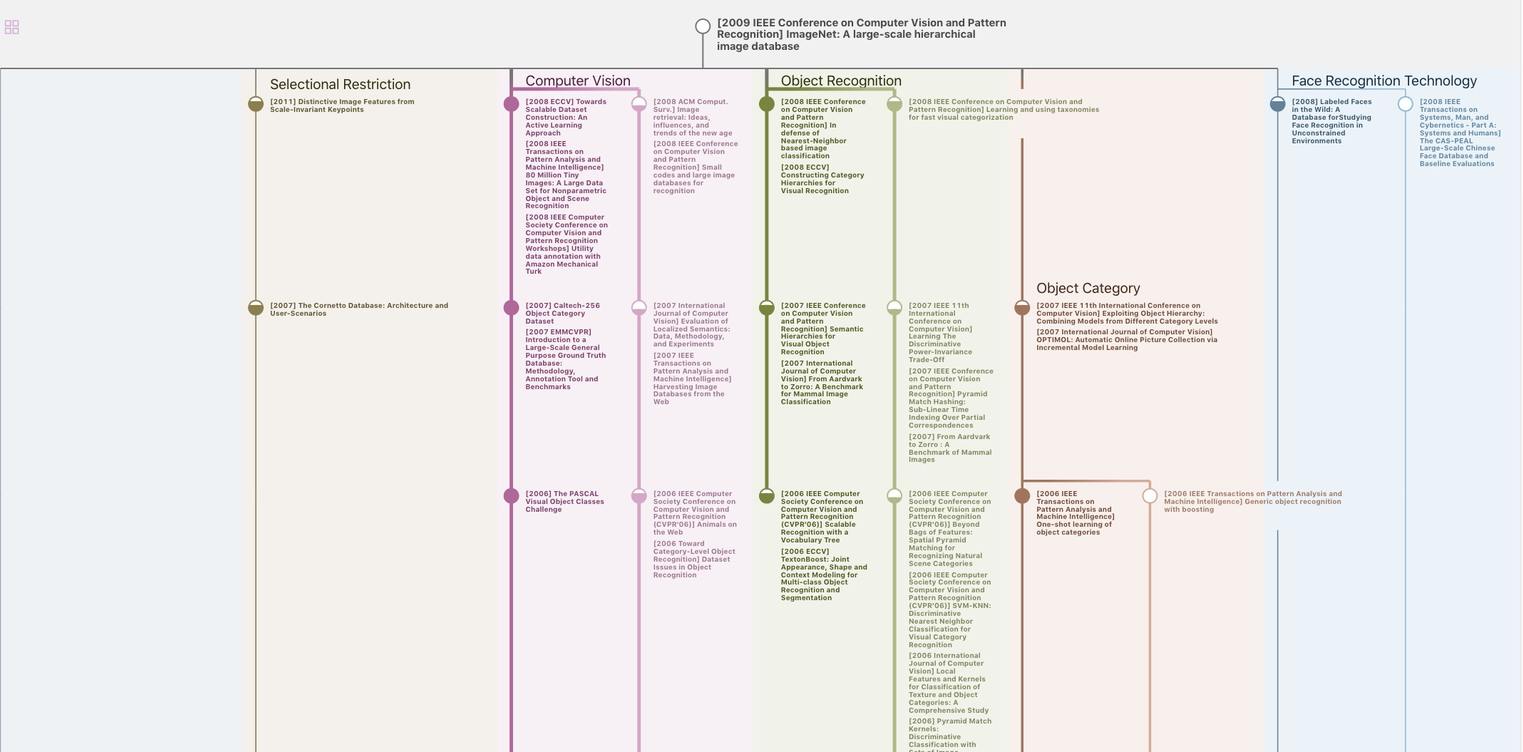
生成溯源树,研究论文发展脉络
Chat Paper
正在生成论文摘要