Gradient harmonized loss: Improving the performance of intelligent diagnosis models in large imbalance scenarios
2022 IEEE International Conference on Prognostics and Health Management (ICPHM)(2022)
摘要
The natural distribution of monitoring data is imbalanced, which has a negative impact on the training of intelligent diagnosis models. Although researchers have proposed data-level and algorithm-level methods to solve this problem, these methods are only applicable to small imbalance scenarios. In order to correct the anomalies of model training under large imbalance scenarios, this paper proposes a gradient harmonized loss that coordinates the gradients of each class to prevent the majority class in the imbalanced data from dominating the training. The coordination of gradients is based on the similarity of the sample gradients, and the compression of similar gradients is achieved by defining different penalty rules for each class. Taking into account the computational efficiency and the training difficulty, the proposed method is further optimized in terms of gradient dimensionality reduction and parameter simplification respectively. The proposed method was verified using two sample sets with different imbalance ratios and compared with traditional methods. The results showed that the proposed method greatly improved the performance of the DCNN model in large imbalance scenarios.
更多查看译文
关键词
rotating machinery,intelligent fault diagnosis,imbalanced data,large imbalance scenarios,gradient harmonization
AI 理解论文
溯源树
样例
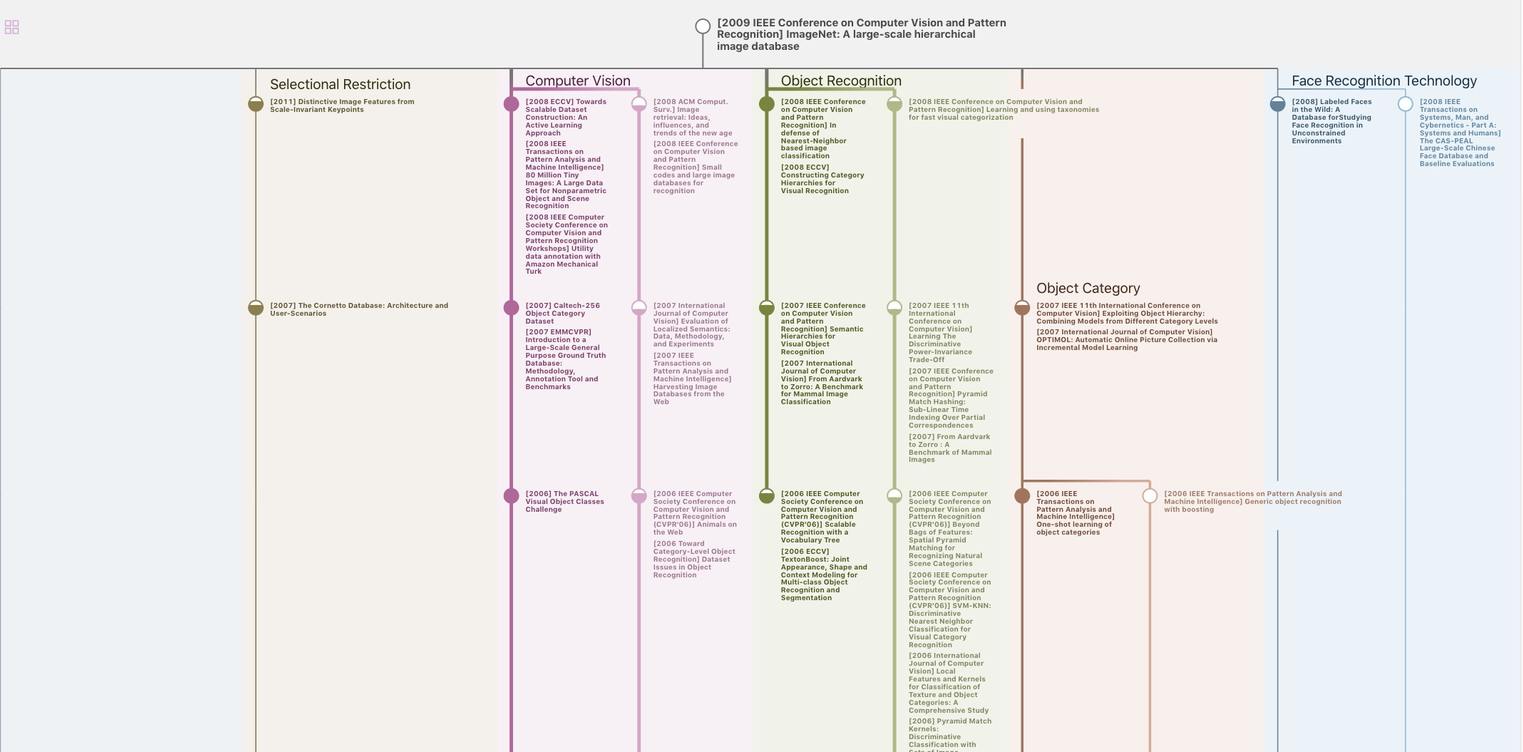
生成溯源树,研究论文发展脉络
Chat Paper
正在生成论文摘要