From Video to Hyperspectral: Hyperspectral Image-Level Feature Extraction with Transfer Learning
REMOTE SENSING(2022)
摘要
Hyperspectral image classification methods based on deep learning have led to remarkable achievements in recent years. However, these methods with outstanding performance are also accompanied by problems such as excessive dependence on the number of samples, poor model generalization, and time-consuming training. Additionally, the previous patch-level feature extraction methods have some limitations, for instance, non-local information is difficult to model, etc. To solve these problems, this paper proposes an image-level feature extraction method with transfer learning. Firstly, we look at a hyperspectral image with hundreds of contiguous spectral bands from a sequential image perspective. We attempt to extract the global spectral variation information between adjacent spectral bands by using the optical flow estimation method. Secondly, we propose an innovative data adaptation strategy to bridge the gap between hyperspectral and video data, and transfer the optical flow estimation network pre-trained with video data to the hyperspectral feature extraction task for the first time. Thirdly, we utilize the traditional classifier to achieve classification. Simultaneously, a vote strategy combined with features at different scales is proposed to improve the classification accuracy further. Extensive, well-designed experiments on four scenes of public hyperspectral images demonstrate that the proposed method (Spe-TL) can obtain results that are competitive with advanced deep learning methods under various sample conditions, with better time effectiveness to adapt to new target tasks. Moreover, it can produce more detailed classification maps that subtly reflect the authentic distribution of ground objects in the original image.
更多查看译文
关键词
hyperspectral image,optical flow,feature extraction,image-level,transfer learning
AI 理解论文
溯源树
样例
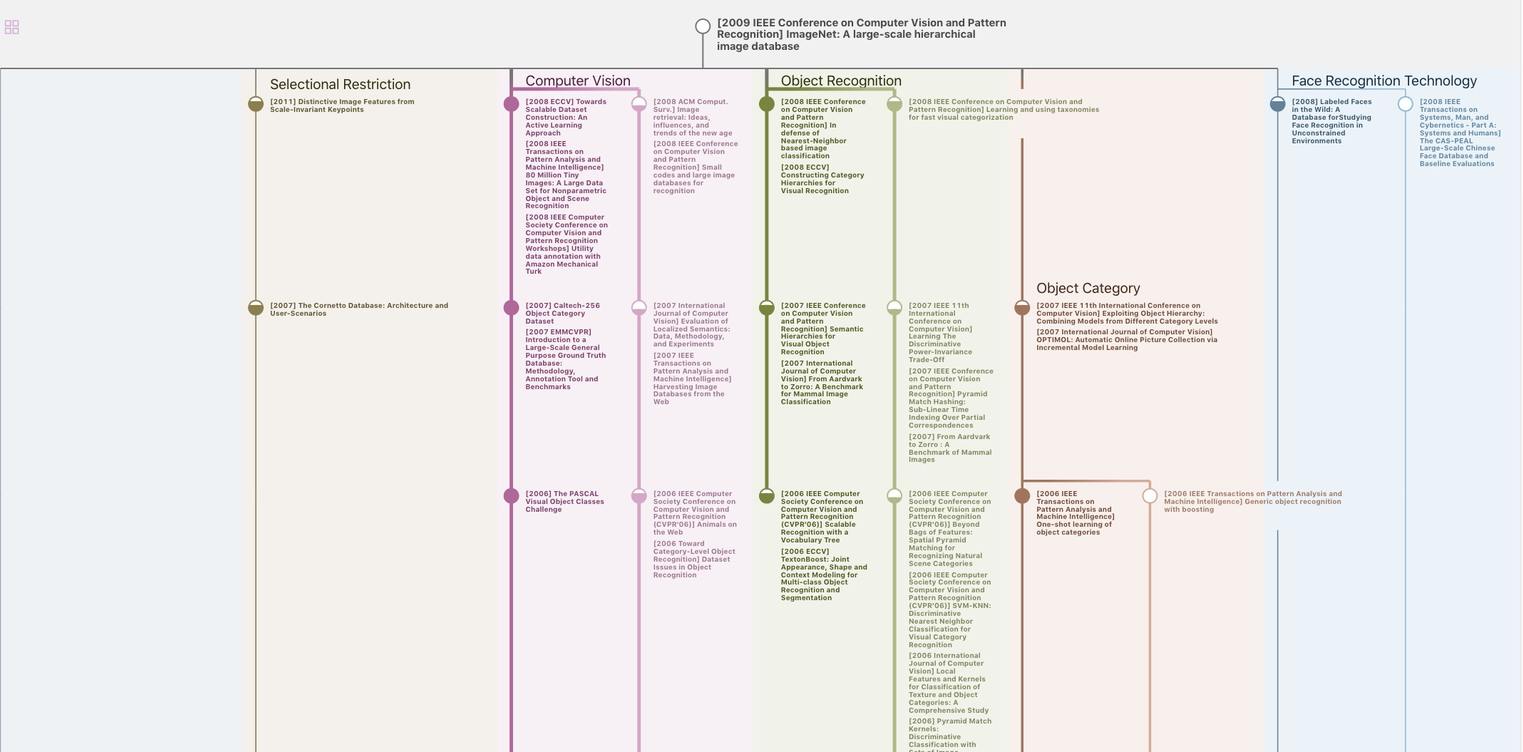
生成溯源树,研究论文发展脉络
Chat Paper
正在生成论文摘要