Reprogramming Large Pretrained Language Models for Antibody Sequence Infilling
arxiv(2022)
摘要
Antibodies comprise the most versatile class of binding molecules, with numerous applications in biomedicine. Therapeutic antibody development requires designing novel and diverse sequences with improved properties, while maintaining the structural consistency. Recently, deep language models and graph neural networks have shown impressive success in antibody sequence generation. Since only a limited number of antibody structures are known, training a model using this limited data can lead to degraded performance, particularly lacking diversity in the generated samples. To address such issues, we leverage the method of Model Reprogramming (MR), which focuses on repurposing pretrained machine learning models for target domain tasks with scarce data, where it may be difficult to train a high-performing model from scratch. We introduce Reprogramming for Protein Sequence Infilling, a framework in which pretrained natural language models are repurposed for protein sequence infilling via reprogramming, to infill protein sequence templates as a method of novel protein generation. For variable CDR sequence design, we formulate the task as text infilling that uses the constant region of an antibody as the sequence template. Results on antibody design benchmarks show that our reprogrammed model on low resourced antibody sequence dataset provides highly diverse CDR sequences, up to more than a two-fold increase of diversity over the baselines, without losing structural integrity and naturalness. The performance benefit of the reprogrammed model learning only from antibody sequences is more evident for longer CDR design or for multiple loop infilling at once, compared to existing graph-based models that require additional structural information. The generated sequences also demonstrate enhanced antigen binding specificity or virus neutralization ability.
更多查看译文
关键词
Antibody,Protein,CDR,Infilling
AI 理解论文
溯源树
样例
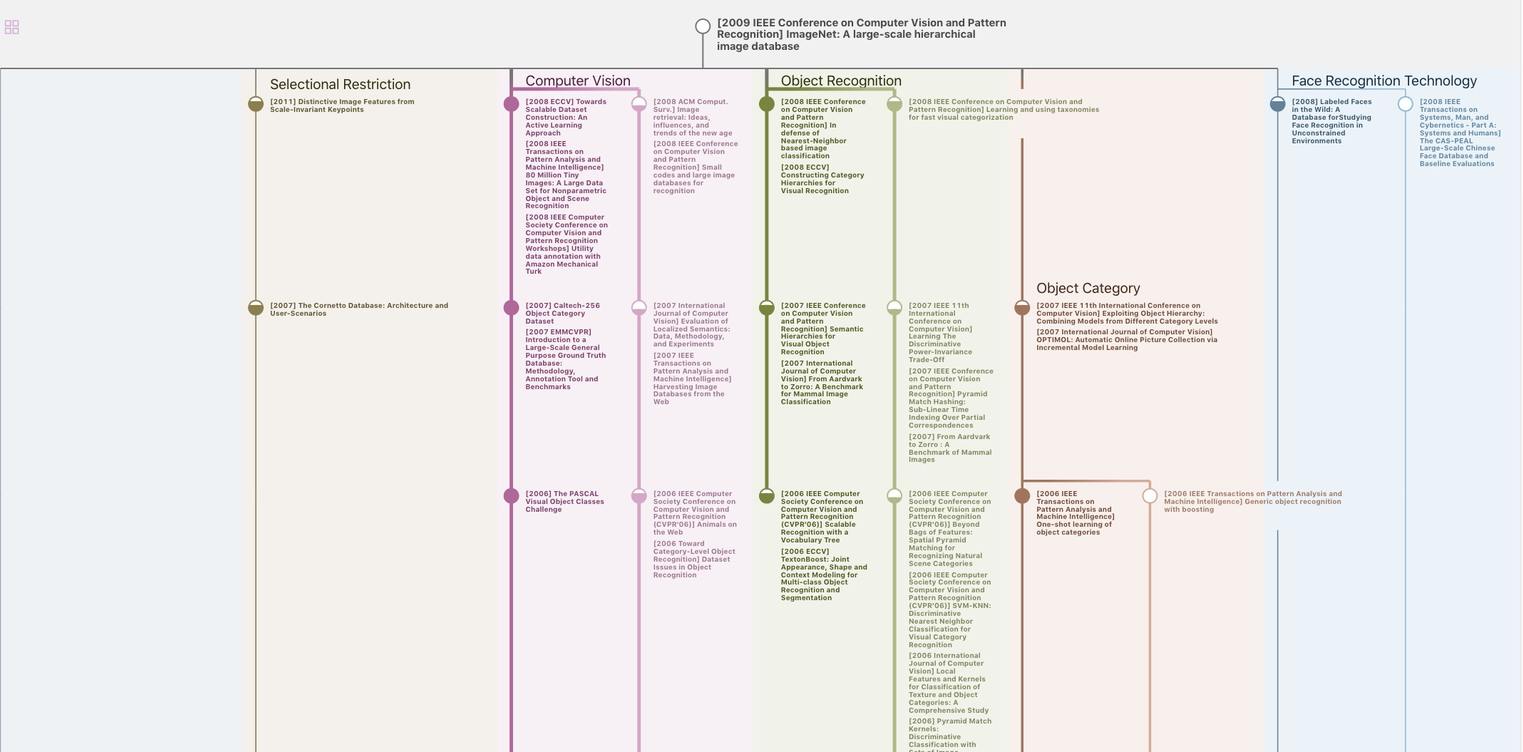
生成溯源树,研究论文发展脉络
Chat Paper
正在生成论文摘要