Machine-learning model for prediction of martensitic transformation temperature in NiMnSn-based ferromagnetic shape memory alloys
Computational Materials Science(2022)
摘要
Martensitic transformation temperature (TM) of NiMnSn-based ferromagnetic shape memory alloys (FSMAs) is crucial to identifying the operating range of an application. From a materials design point of view, an efficient method that can predict the TM accurately should be strongly pursued, to meet various applications with different operating temperatures. In this paper, we demonstrate that machine learning (ML) can rapidly and accurately predict the TM in NiMnSn-based FSMAs. We evaluate the performance of four machine learning models, including Random Forest Regressor (RFR), Support Vector Regression (SVR), Linear Regression (LR), and XGBRegressor (XGBR) model. Three important features of Numa, Arc, and avg Ven are selected as the optimal feature combination for building the model. Moreover, to ensure the best generalization ability of the model, multiple methods of cross-validation (Leave-One-Out Cross-Validation, 3-fold Cross-Validation, and 5-fold Cross-Validation) are used. Finally, the XGBR model exhibits the best performance for predicting the TM (R2 = 0.903 and RMSE = 5.4, R5f2 = 0.869 and R3f2 = 0.838). The results of small deviation and variance proven that the XGBR model, proposed in this work, is suitable to be used to predict the TM of unknown NiMnSn-based FSMAs. This work is expected to promote the targeted design of FSMAs.
更多查看译文
关键词
Ferromagnetic shape memory alloys,Martensitic transformation temperature,Machine learning,NiMnSn-based alloys,XGBRegressor
AI 理解论文
溯源树
样例
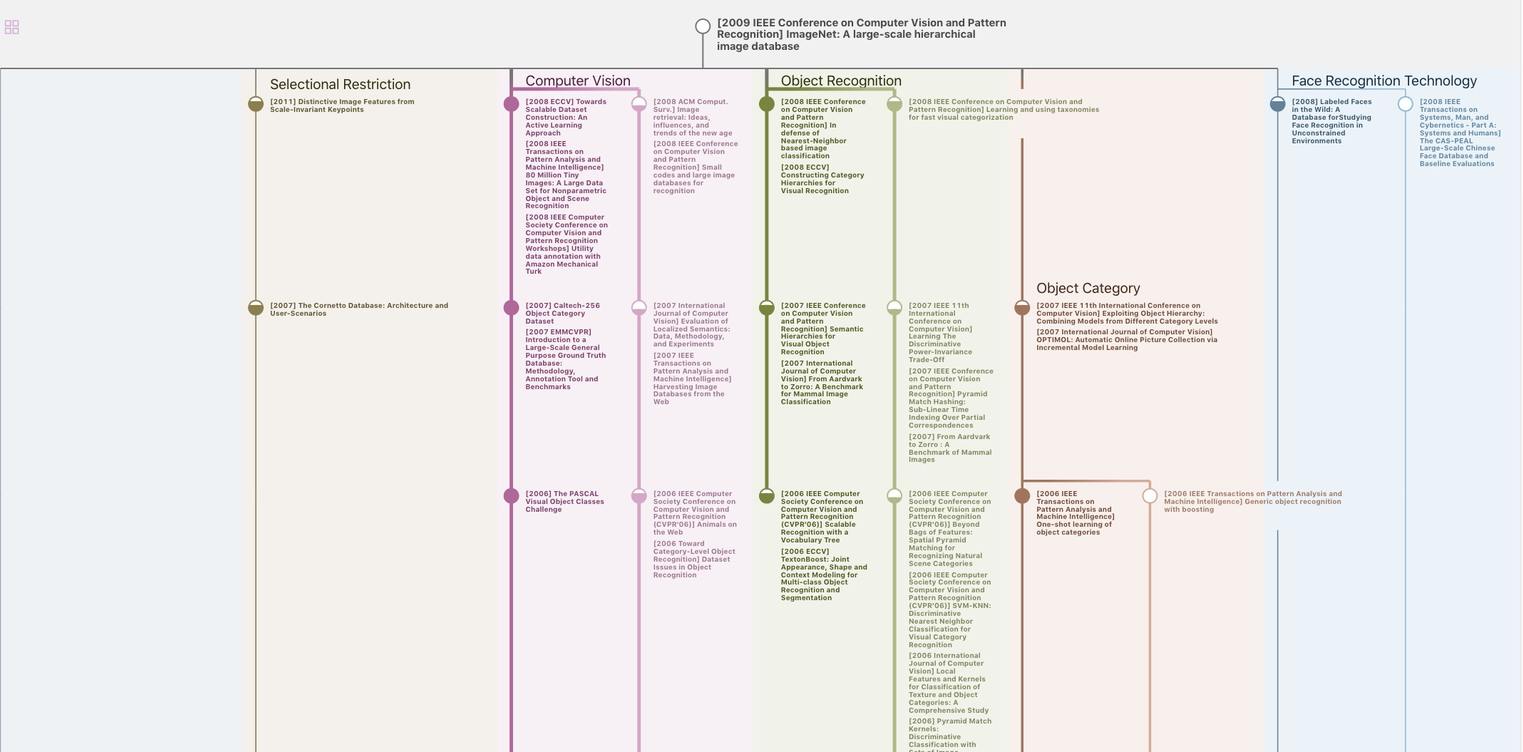
生成溯源树,研究论文发展脉络
Chat Paper
正在生成论文摘要