Fast Bayesian inference on spectral analysis of multivariate stationary time series
Computational Statistics & Data Analysis(2023)
摘要
Spectral analysis discovers trends, periodic and other characteristics of a time series by representing these features in the frequency domain. However, when multivariate time series are considered, and the number of components increases, the size of the spectral density matrix grows quadratically, making estimation and inference rather challenging. The proposed novel Bayesian framework considers a Whittle likelihood -based spectral modeling approach and imposes a discounted regularized horseshoe prior on the coefficients that define a spline representation of each of the components of a Cholesky factorization of the inverse spectral density matrix. The proposed prior structure leads to a model that provides higher posterior accuracy when compared to alternative currently available approaches. To achieve fast inference that takes advantage of the massive power of modern hardware (e.g., GPU), a stochastic gradient variational Bayes approach is proposed for the highly parallelizable posterior inference that provides computational flexibility for modeling high-dimensional time series. The accurate empirical performance of the proposed method is illustrated via extensive simulation studies and the analysis of two datasets: a wind speed data from 6 locations in California, and a 61 -channel electroencephalogram data recorded on two contrasting subjects under specific experimental conditions. (C) 2022 Elsevier B.V. All rights reserved.
更多查看译文
关键词
Multivariate time series,Spectral analysis,Stochastic gradient variational Bayes,Global -local shrinkage prior
AI 理解论文
溯源树
样例
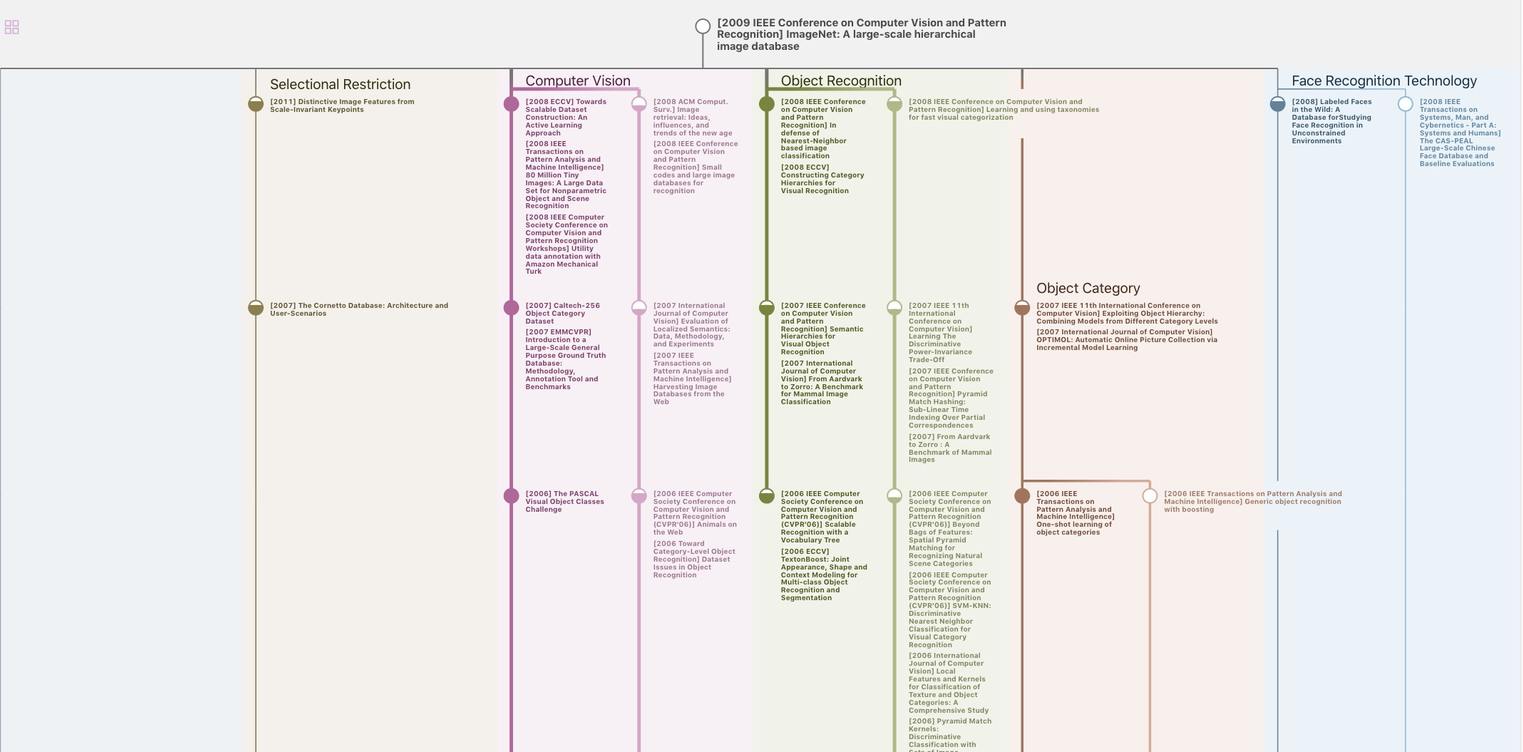
生成溯源树,研究论文发展脉络
Chat Paper
正在生成论文摘要