Data-driven multi-objective optimization of ultralight hierarchical origami-corrugation meta-sandwich structures
Composite Structures(2023)
摘要
With advantages in stiffness, strength, and energy absorption, hierarchical origami-corrugation meta-sandwich (HOCM) structures are envisioned as a novel ultralight multifunctional sandwich construction for various practical applications. Firstly, the macro-equivalent compressive modulus is analytically derived using a two -level homogenization approach. The HOCM samples manufactured by selective laser melting are then tested under quasi-static out-of-plane compressive loading. A representative volume element model is proposed for finite element simulations to explore further the compressive performance, with good agreement achieved be-tween measurements and simulations. Influences of critical geometric parameters on compressive properties, including initial failure modes, specific peak strength (SPS) and specific energy absorption (SEA), are numeri-cally analyzed. Subsequently, the surrogate model based on a fully connected neural network algorithm is selected as the machine learning strategy to approximate the SPS and SEA, with cross-validation conducted to verify its accuracy. Finally, a multi-objective optimization method incorporating the surrogate model and the non-dominated sorting genetic algorithm II is implemented to carry out optimal design for HOCM structures possessing simultaneous superior SPS, SEA with assured stiffness. Such a data-driven optimization procedure based on the machine learning method exhibits high accuracy for strongly nonlinear problems, especially for SEA in current work, leading to highly efficient optimization.
更多查看译文
关键词
Origami -corrugation meta -sandwich,Compressive performance,Fully connected neural networks,Optimal design
AI 理解论文
溯源树
样例
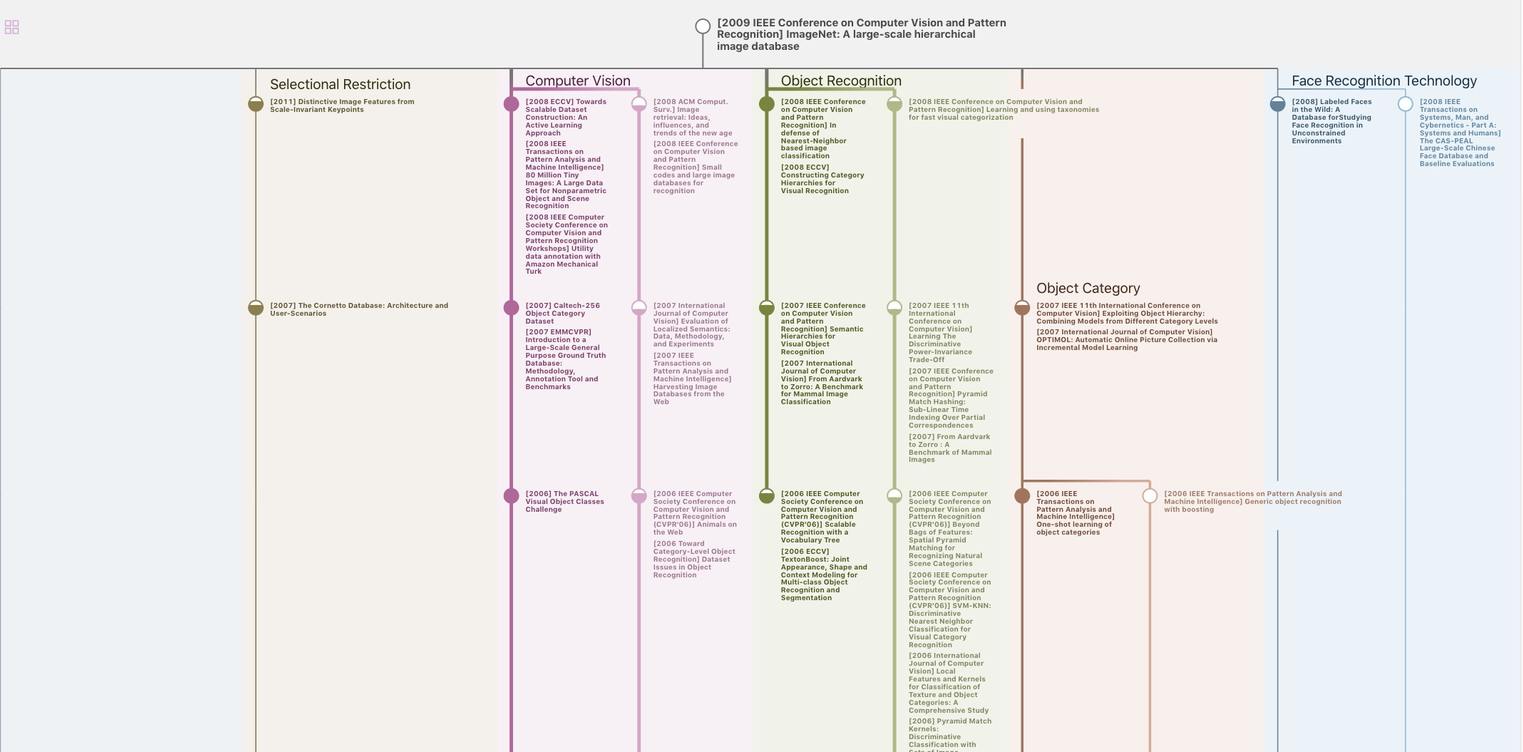
生成溯源树,研究论文发展脉络
Chat Paper
正在生成论文摘要