Evaluation and Development of a Predictive Model for Geophysical Well Log Data Analysis and Reservoir Characterization: Machine Learning Applications to Lithology Prediction
Natural Resources Research(2022)
摘要
This work critically evaluated the applicability of machine learning methodology applied to automated well log creation towards reliable lithology prediction and subsequent reservoir characterization to overcome the computationally intensive and laborious manual analysis of well logs for improving the cost of exploration, better accuracy of predictions, as well as improved efficiency of production operations. We propose an approach by deploying a correlation tool for parametric correlation between parameters and implementation of machine learning algorithms for understanding hidden meaningful relationships between the feature and target variables for the development of a predictive model. Clustering classification and label extraction followed by dimensionality reduction using principal components analysis were performed in the unsupervised mode. The calibration of the functional relationship between the predictor attributes and target properties by determining the most powerful predictor influencing the target parameters helped to derive interpretations with greater degree of certainty to develop a reliable predictive model using regression analysis in supervised mode. The results were based on a set of five drilled core holes, namely, Rutgers, Somerset, Martinsville, Princeton, and Weston Canal of Newark South Basin, USA, a publicly available set of well logs at the Southern Newark Basin section at the Lamont-Doherty Earth Observatory. The data were split 80:20 into a training set and a test set for evaluation. The evaluation carried out using the R-squared and the root mean squared error to validate the proposed approach revealed values of 0.726 and 0.232, respectively, for the training set and 0.723 and 0.186, respectively, for the testing set. A comparative analysis with state-of-the-art methods such as k-nearest neighbor, support vector regression (SVR), and multivariate regression was also performed. This implementation demonstrated the proposed model’s efficacy well beyond the conventional methods.
更多查看译文
关键词
Machine learning,Data visualization,Well logs,Reservoir characterization,Lithology prediction,Petro-physics
AI 理解论文
溯源树
样例
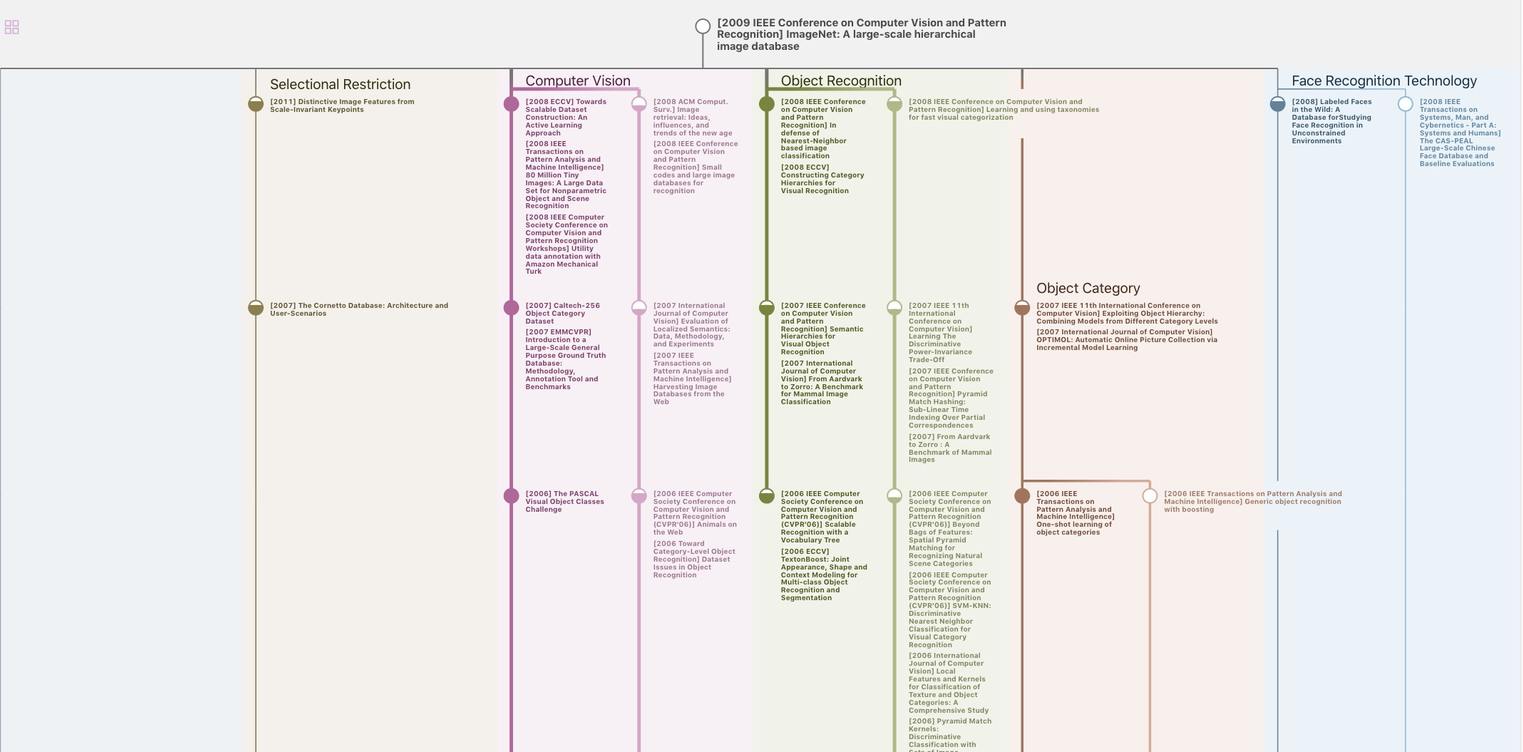
生成溯源树,研究论文发展脉络
Chat Paper
正在生成论文摘要