Min-Max Cost and Information Control in Multi-layered Neural Networks
Proceedings of the Future Technologies Conference (FTC) 2022, Volume 1(2022)
摘要
The present paper aims to propose a new method to minimize and maximize information and its cost, accompanied by the ordinary error minimization. All these computational procedures are operated as independently as possible from each other. This method aims to solve the contradiction in conventional computational methods in which many procedures are intertwined with each other, making it hard to compromise among them. In particular, we try to minimize information at the expense of cost, followed by information maximization, to reduce humanly biased information obtained through artificially created input variables. The new method was applied to the detection of relations between mission statements and firms’ financial performance. Though the relation between them has been considered one of the main factors for strategic planning in management, the past studies could only confirm very small positive relations between them. In addition, those results turned out to be very dependent on the operationalization and variable selection. The studies suggest that there may be some indirect and mediating variables or factors to internalize the mission statements in organizational members. If neural networks have an ability to infer those mediating variables or factors, new insight into the relation can be obtained. Keeping this in mind, the experiments were performed to infer some positive relations. The new method, based on minimizing the humanly biased effects from inputs, could produce linear, non-linear, and indirect relations, which could not be extracted by the conventional methods. Thus, this study shows a possibility for neural networks to interpret complex phenomena in human and social sciences, which, in principle, conventional models cannot deal with.
更多查看译文
关键词
Min-max property, Cost, Information, Generalization, Interpretation, Mission statements
AI 理解论文
溯源树
样例
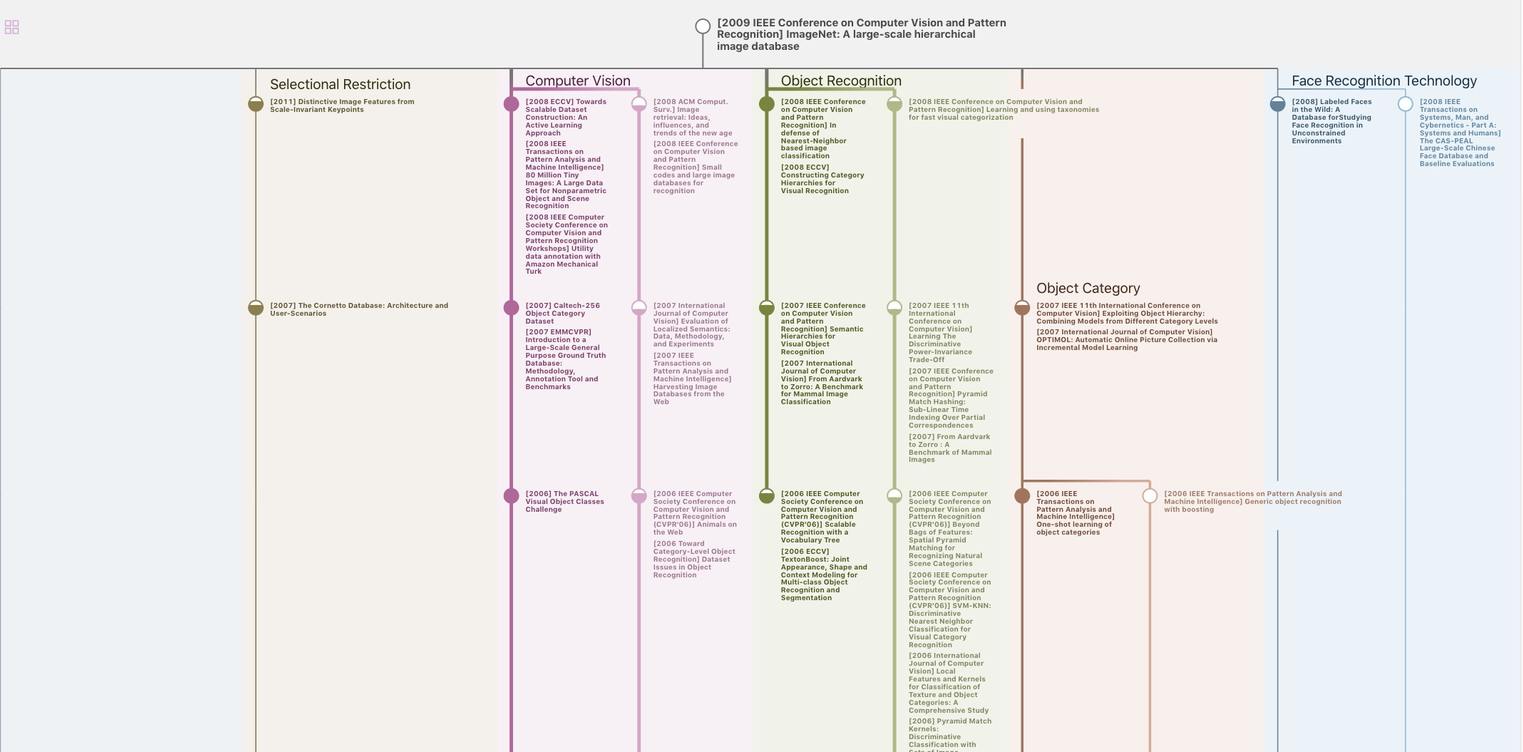
生成溯源树,研究论文发展脉络
Chat Paper
正在生成论文摘要