Conditional interval reduction method: A possible new direction for the optimization of process based models.
Environ. Model. Softw.(2022)
摘要
Application of process-based models at different spatial scales requires their proper parameterization. This task is typically executed using trial-and-error parameter adjustment or a probabilistic method. Practical application of the probabilistic methods is hampered by methodological complexity and lack of interpretability. Here we present a novel approach for the parameterization of process-based models that we call as conditional interval refinement method (CIRM). The method can be best described as the combination of a probabilistic approach and the advantages of the expert-based parameter adjustment. CIRM was demonstrated by optimizing the BiomeBGCMuSo biogeochemical model using maize yield observations. The proposed approach uses the General Likelihood Uncertainty Estimation (GLUE) method with additional expert knowledge, supplemented by the construction and interpretation of decision trees. It was demonstrated that the iterative, fully automatic method successfully constrained the parameter intervals meanwhile our confidence on the parameters increased. The algorithm can easily be implemented with other process-based models.
更多查看译文
关键词
Model optimization, Bayesian calibration, Parameter constraints, Decision tree
AI 理解论文
溯源树
样例
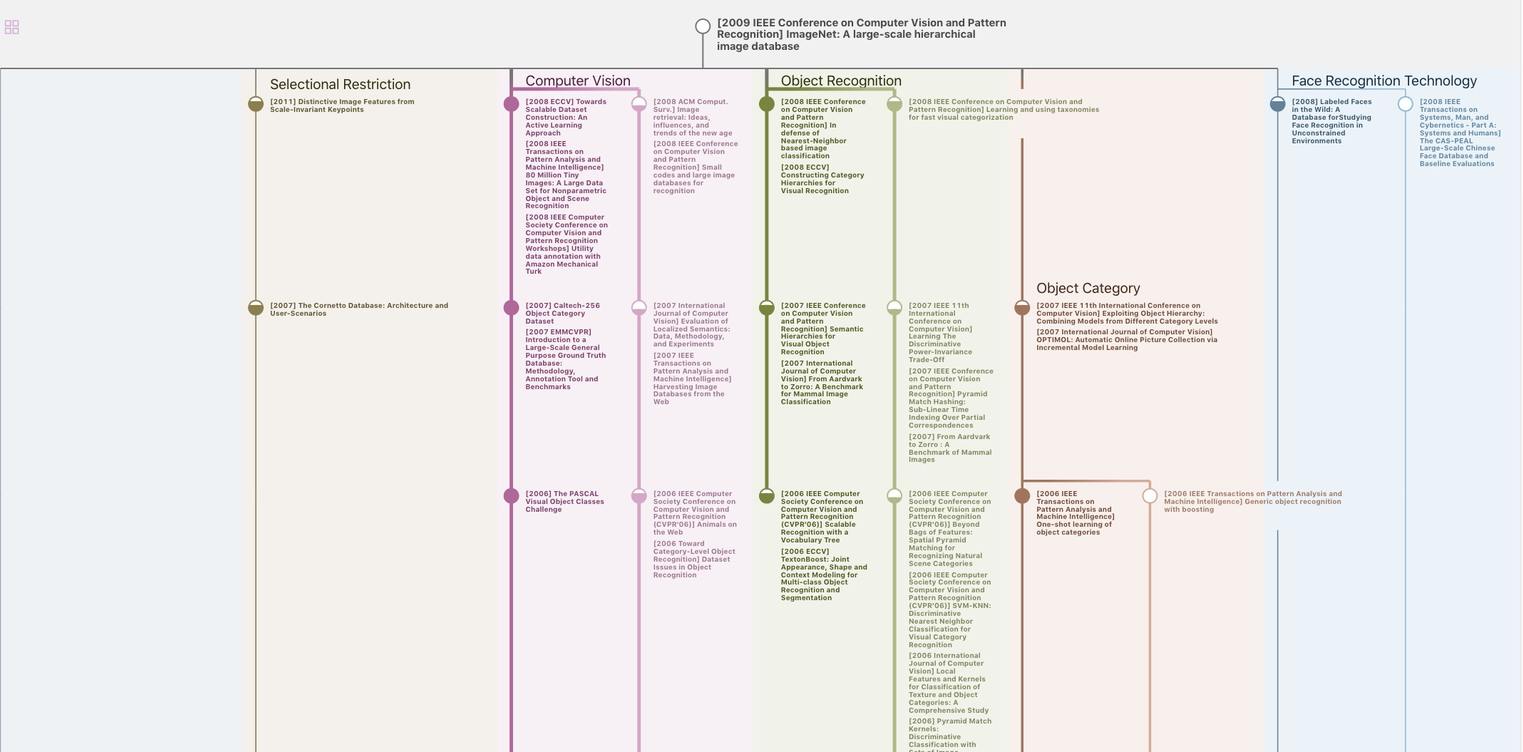
生成溯源树,研究论文发展脉络
Chat Paper
正在生成论文摘要