SIRAJ: A Unified Framework for Aggregation of Malicious Entity Detectors
2022 IEEE Symposium on Security and Privacy (SP)(2022)
摘要
High-quality intelligence of Internet threat (e.g., malware files, malicious domains, phishing URLs and malicious IPs) are important for both security practitioners and the research community. Given the agility of attackers, the scale of the Internet, and the fast-evolving landscape of threats, one could not rely solely on a single source (such as an anti-malware engine or an IP blacklist) for obtaining accurate, up-to-date, and comprehensive threat analysis. Instead, we need to aggregate the analysis from multiple sources. However, it is non-trivial to do such aggregation effectively. A common practice is to label an indicator (malware, domains, URLs, etc.) as malicious if it is marked by a number of sources above an ad-hoc certain threshold. Often, this results in sub-optimal performance as it assumes that all sources are of similar quality/expertise, independent, and temporally stable, which unfortunately are often not true in practice. A natural alternative is to train a supervised machine learning model. However, this approach needs a sufficiently large amount of manually labeled ground truth, which is time-consuming to collect and has to be updated frequently, resulting in substantial recurring costs. In this paper, we propose SIRAJ, a novel framework for aggregating the detection output of various intelligence sources such as anti-malware engines. SIRAJ is based on the pretrain and fine-tune paradigm. Specifically, we use self-supervised learning-based approaches to learn a pre-trained embedding model that converts multi-source inputs into a high-dimensional embedding. The embeddings are learned through three carefully designed pretext tasks that imbue them with knowledge about dependencies between scanners and their temporal dynamics. The learned embeddings could be used for diverse downstream machine learning tasks. SIRAJ is designed to be general and can be used for diverse domains such as URLs, malware, and IPs. Further, SIRAJ works well even when there is limited to no labeled data available. Through extensive experiments, we show that our learned representations can produce results comparable to supervised methods while only requiring as little as 100 labeled samples. Importantly, the results show that SIRAJ accurately detects threat indicators much earlier than the baseline algorithms, a feat that is critical against short-lived indicators like Phishing URLs.
更多查看译文
关键词
threat-intelligence-aggregation,ground-truth-generation,malicious-entities,truth-discovery,self-supervised-learning
AI 理解论文
溯源树
样例
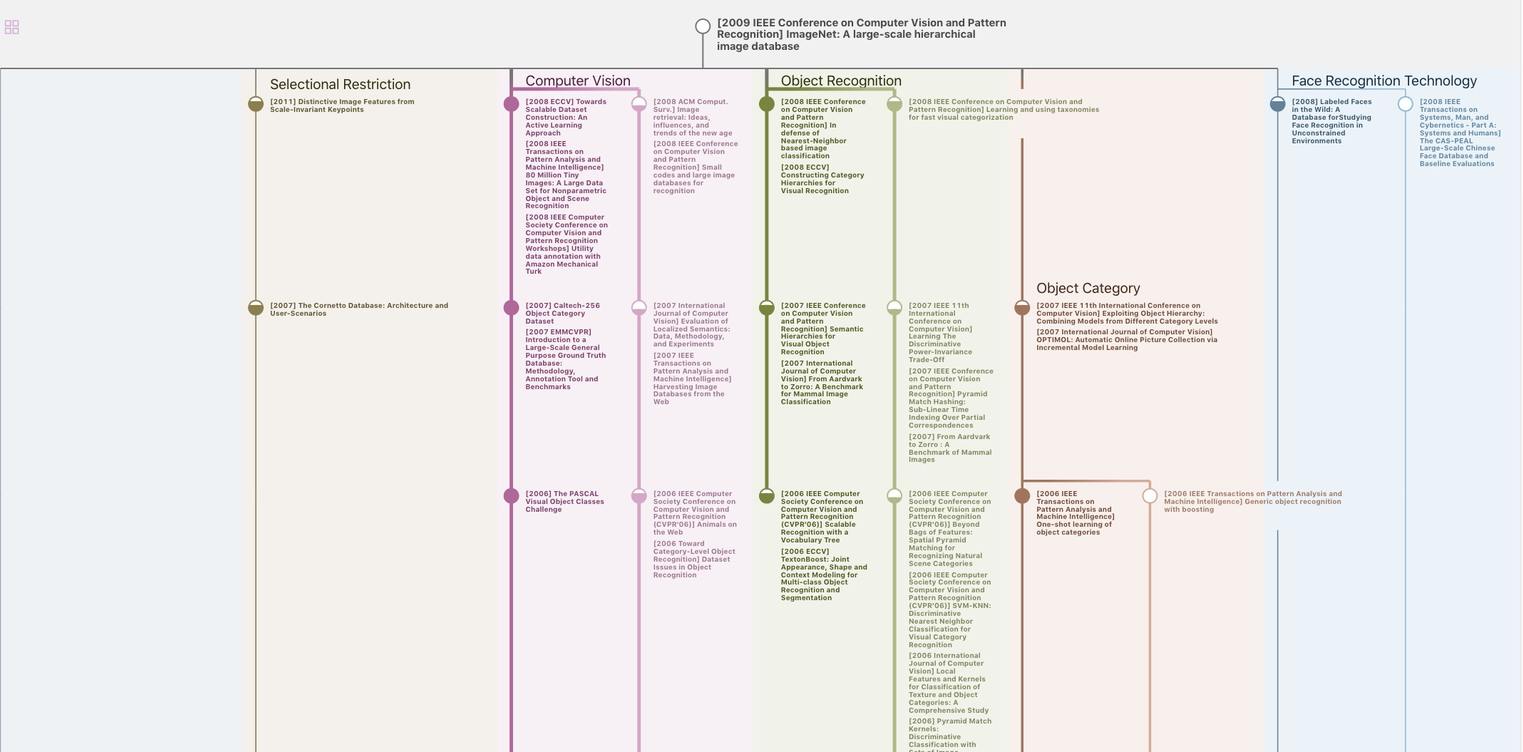
生成溯源树,研究论文发展脉络
Chat Paper
正在生成论文摘要