Measuring and Improving Semantic Diversity of Dialogue Generation
CoRR(2022)
摘要
Response diversity has become an important criterion for evaluating the quality of open-domain dialogue generation models. However, current evaluation metrics for response diversity often fail to capture the semantic diversity of generated responses, as they mainly consider lexical aspects of the generated responses. In this paper, we introduce a new automatic evaluation metric to measure the semantic diversity of generated responses. Through human evaluation, we demonstrate that our proposed metric captures human judgments on response diversity better than existing lexical-level diversity metrics. Furthermore, motivated by analyzing an existing dialogue dataset, we propose a simple yet effective learning method that improves the semantic diversity of generated responses. Our learning method weights training samples based on the semantic distribution of the training set. We show that our learning method improves response diversity and coherency better than other baseline methods through automatic and human evaluation.
更多查看译文
关键词
dialogue generation,semantic diversity
AI 理解论文
溯源树
样例
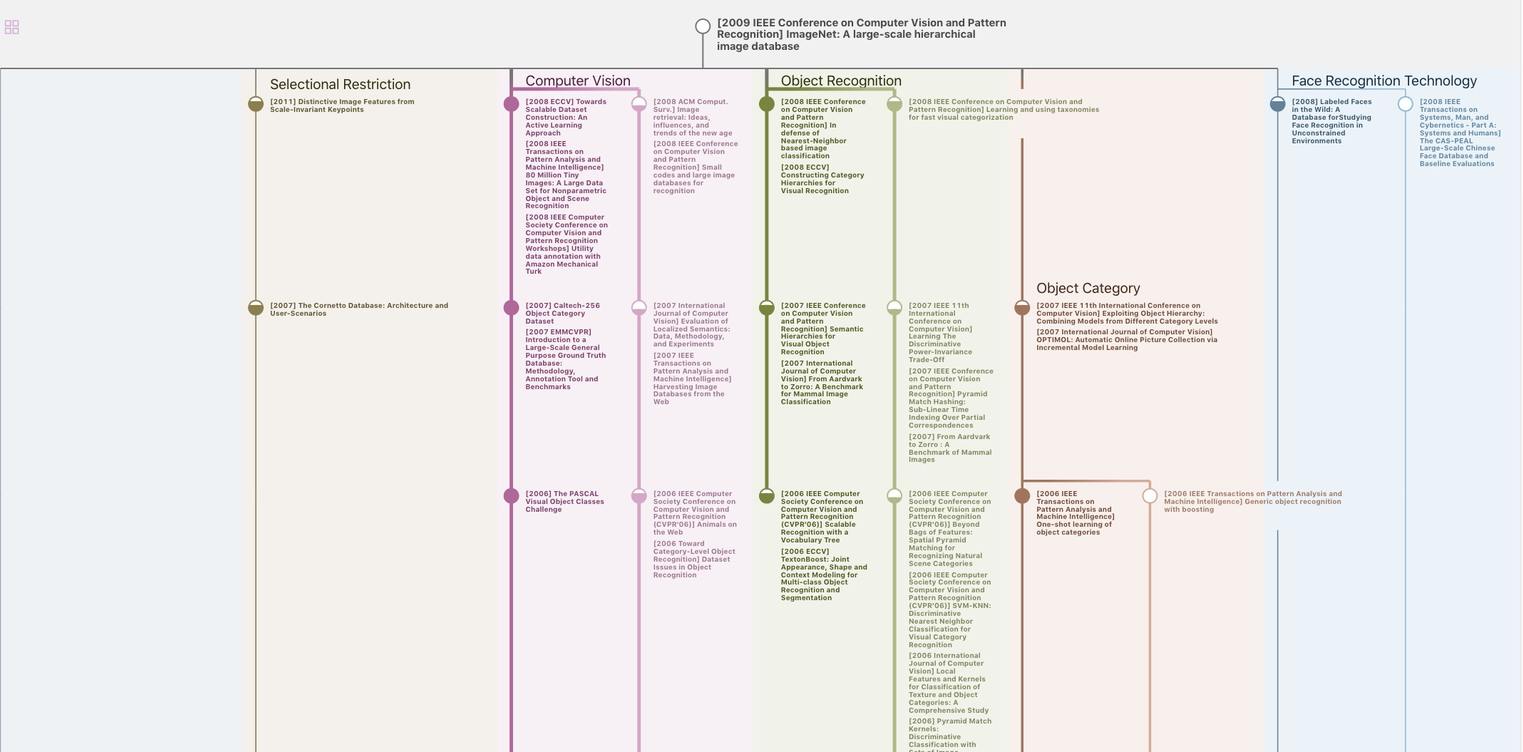
生成溯源树,研究论文发展脉络
Chat Paper
正在生成论文摘要