Predicting Volatile Fatty Acid Synthesis from Palm Oil Mill Effluent on an Industrial Scale
Biochemical Engineering Journal(2022)
摘要
To determine the optimal conditions of anaerobic digestion of palm oil mill effluent (POME) for volatile fatty acid (VFA) synthesis on an industrial scale can be costly and time-consuming due to the large number of variables needed to be tested. In this study, variational autoencoder (VAE) was used to generate simulated data to expand the small size dataset. Then, machine learning models were developed to predict VFA synthesis. Using VAE, 250 simulated data were generated from 25 real data, and a total of 275 samples used for training/validation/testing of machine learning models. The dataset consists of features such as POME volume, COD loading, organic loading rate, temperature, pH. Random forest (RF) and artificial neural network (ANN) were trained and tested on predictions of VFA synthesis. Generally, ANN performed better than RF. To improve the performance, the 3 best performing ANN models were selected for ensemble neural network, where it has robust performances with RMSE of 55.80, R2 of 0.968 on test data. Features importance analysis indicated that temperature was the most important factor influencing VFA synthesis, suggesting that temperature control could improve VFA synthesis. Overall, ANN is robust to predict VFA synthesis while features importance analysis could provide the guidance for adjustment of optimal conditions, reducing the resources and workloads of experiments in real situations.
更多查看译文
关键词
Machine learning,Artificial neural networks,Anaerobic digestion,Waste treatment
AI 理解论文
溯源树
样例
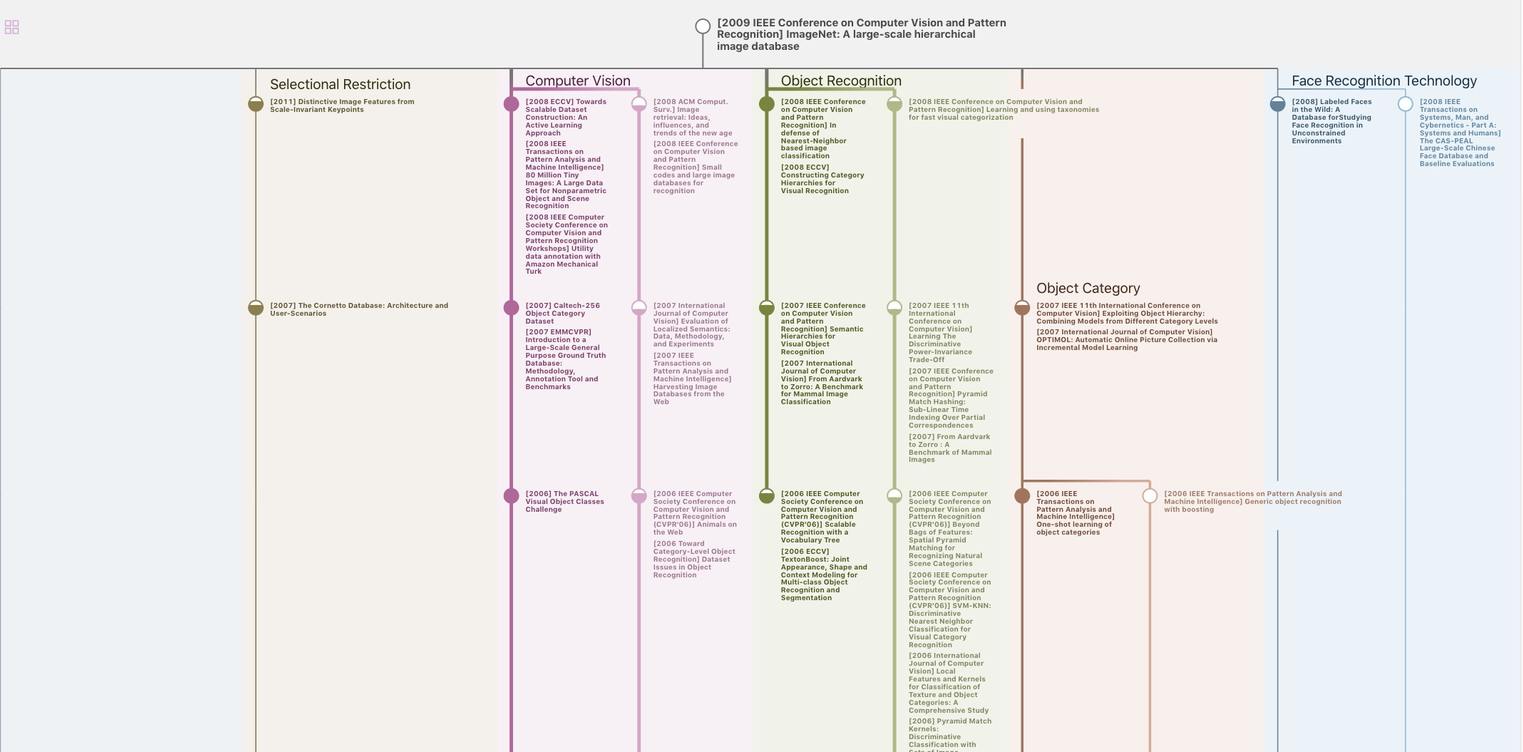
生成溯源树,研究论文发展脉络
Chat Paper
正在生成论文摘要