LidarNAS: Unifying and Searching Neural Architectures for 3D Point Clouds.
European Conference on Computer Vision(2022)
摘要
Developing neural models that accurately understand objects in 3D point clouds is essential for the success of robotics and autonomous driving. However, arguably due to the higher-dimensional nature of the data (as compared to images), existing neural architectures exhibit a large variety in their designs, including but not limited to the views considered, the format of the neural features, and the neural operations used. Lack of a unified framework and interpretation makes it hard to put these designs in perspective, as well as systematically explore new ones. In this paper, we begin by proposing a unified framework of such, with the key idea being factorizing the neural networks into a series of view transforms and neural layers. We demonstrate that this modular framework can reproduce a variety of existing works while allowing a fair comparison of backbone designs. Then, we show how this framework can easily materialize into a concrete neural architecture search (NAS) space, allowing a principled NAS-for-3D exploration. In performing evolutionary NAS on the 3D object detection task on the Waymo Open Dataset, not only do we outperform the state-of-the-art models, but also report the interesting finding that NAS tends to discover the same macro-level architecture concept for both the vehicle and pedestrian classes.
更多查看译文
关键词
searching neural architectures,clouds
AI 理解论文
溯源树
样例
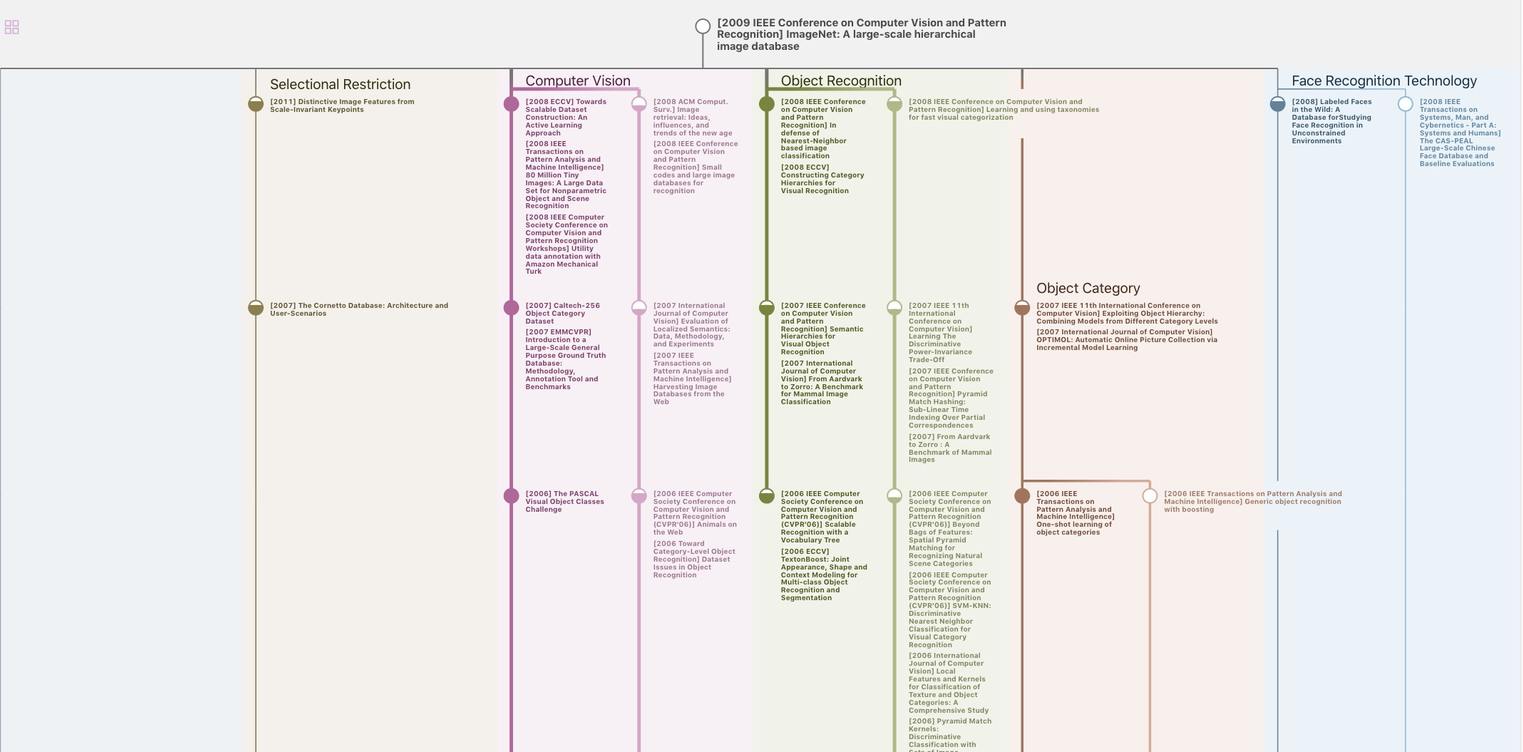
生成溯源树,研究论文发展脉络
Chat Paper
正在生成论文摘要