Pattern‐based clustering of daily weigh‐in trajectories using dynamic time warping
Biometrics(2022)
摘要
"Smart"-scales are a new tool for frequent monitoring of weight change as well as weigh-in behavior. These scales give researchers the opportunity to discover patterns in the frequency that individuals weigh themselves over time, and how these patterns are associated with overall weight loss. Our motivating data come from an 18-month behavioral weight loss study of 55 adults classified as overweight or obese who were instructed to weigh themselves daily. Adherence to daily weigh-in routines produces a binary times series for each subject, indicating whether a participant weighed in on a given day. To characterize weigh-in by time-invariant patterns rather than overall adherence, we propose using hierarchical clustering with dynamic time warping (DTW). We perform an extensive simulation study to evaluate the performance of DTW compared to Euclidean and Jaccard distances to recover underlying patterns in adherence time series. In addition, we compare cluster performance using cluster validation indices (CVIs) under the single, average, complete, and Ward linkages and evaluate how internal and external CVIs compare for clustering binary time series. We apply conclusions from the simulation to cluster our real data and summarize observed weigh-in patterns. Our analysis finds that the adherence trajectory pattern is significantly associated with weight loss.
更多查看译文
关键词
binary time series,dynamic time warping,hierarchical clustering,"smart"-scales
AI 理解论文
溯源树
样例
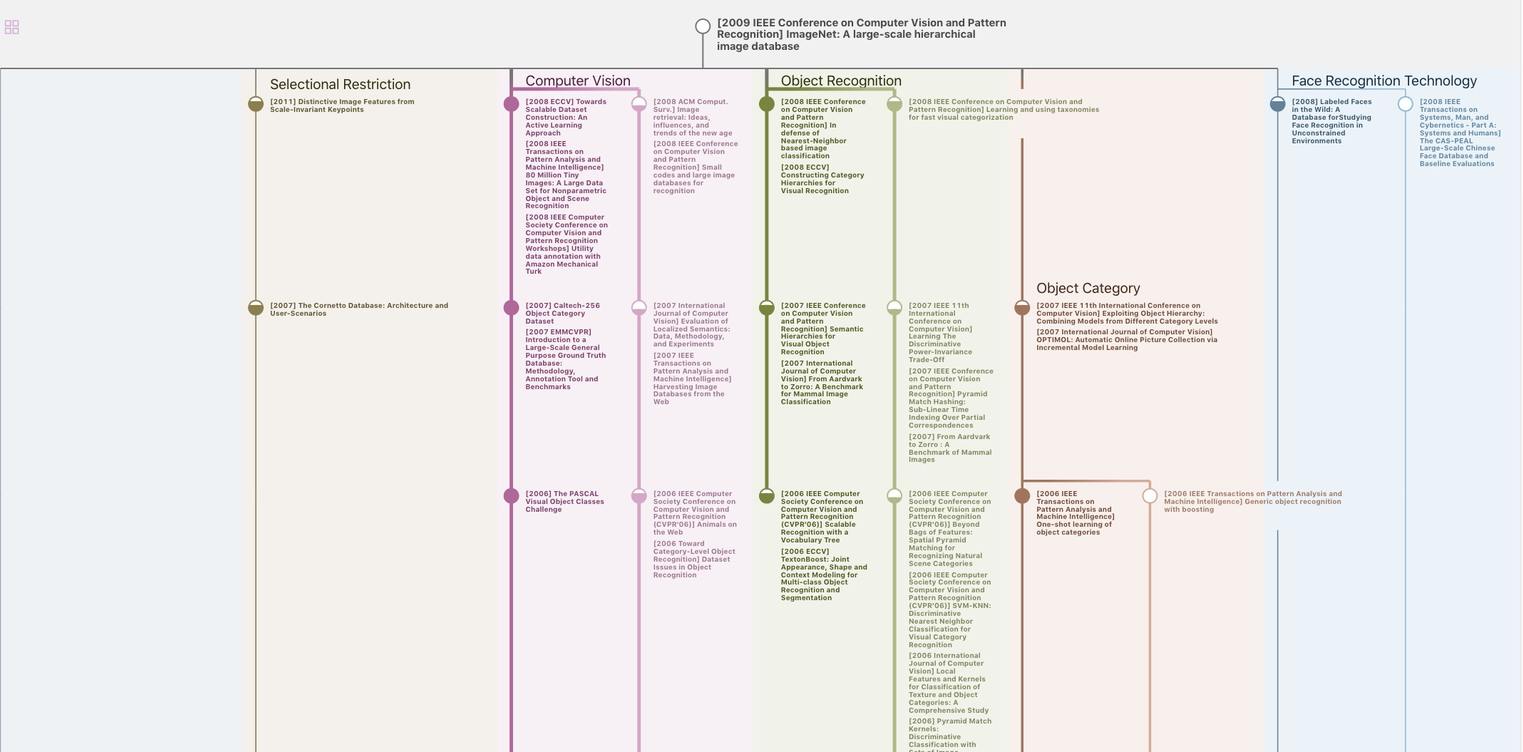
生成溯源树,研究论文发展脉络
Chat Paper
正在生成论文摘要