Application of machine learning for improved accuracy of simultaneous temperature and strain measurements of carbon fiber-reinforced plastic laminates using an embedded tilted fiber Bragg grating sensor
Composites Part A: Applied Science and Manufacturing(2022)
摘要
Tilted FBG (TFBG) sensors exhibit capability for simultaneous measurement of temperature and strain. However, improving the measurement accuracy is difficult. This study examined the applicability of machine learning techniques for improving the accuracy of simultaneous measurement of temperature and strain of a TFBG sensor, and of a carbon fiber-reinforced plastic (CFRP) composite laminate using an embedded TFBG sensor. Temperature and strain were ascertained using machine learning methods, i.e. deep neural networks (deep NN) and support vector regression (SVR), from multiple peaks in the transmission spectrum. The results obtained using the machine learning methods were compared directly to those found using a conventional method with the simultaneous linear equation of strain and temperature cross-sensitivity by extracting two peaks from the transmission spectrum. Results demonstrated that the accuracy was improved significantly using deep NN method with Bayesian regularization compared to the conventional cross-sensitivity method.
更多查看译文
关键词
CFRP,Deep neural networks,FBG sensor,Machine learning
AI 理解论文
溯源树
样例
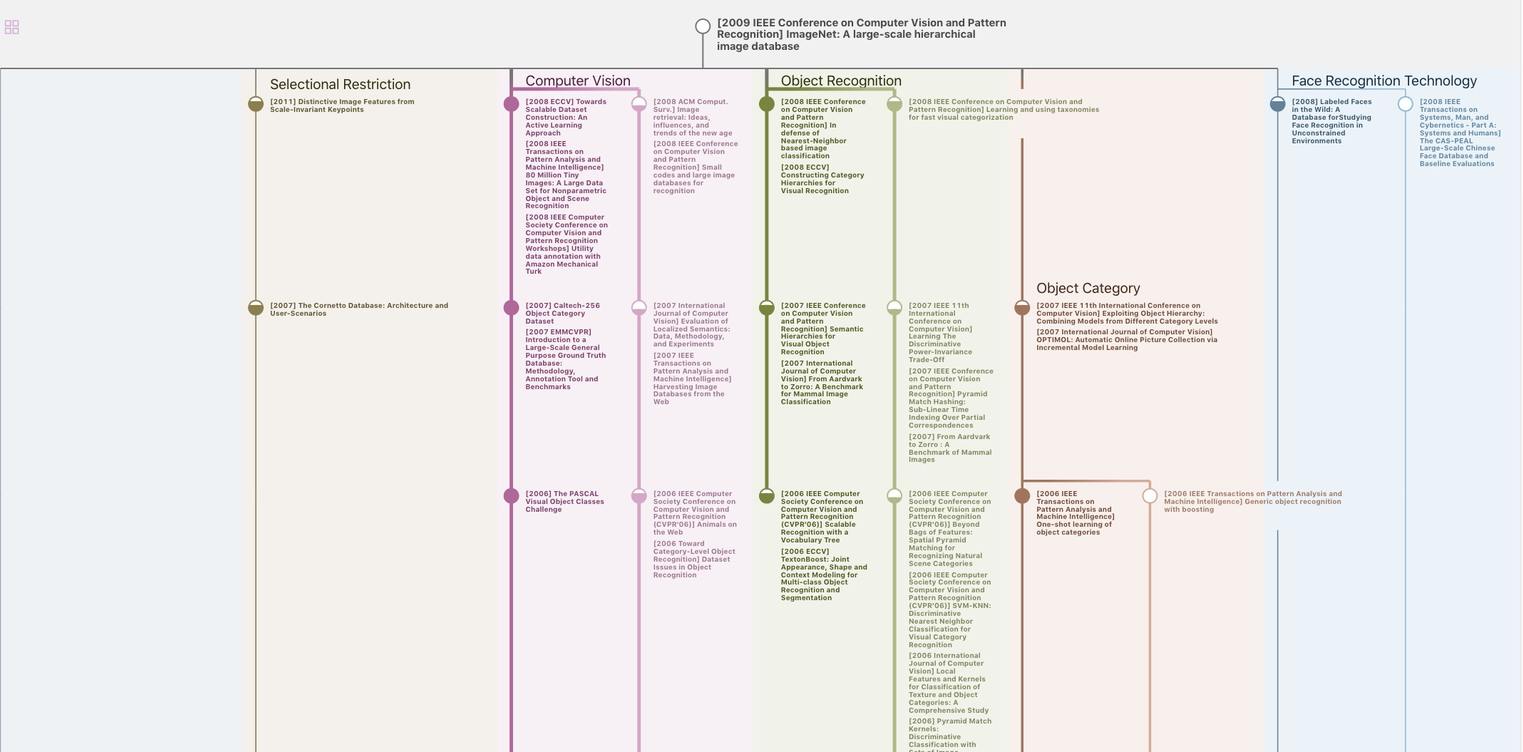
生成溯源树,研究论文发展脉络
Chat Paper
正在生成论文摘要