Structure-Preserving Motion Estimation for Learned Video Compression
ACM International Conference on Multimedia (MM)(2022)
摘要
ABSTRACTFollowing the conventional hybrid video coding framework, existing learned video compression methods rely on the decoded previous frame as the reference for motion estimation considering that it is available to the decoder. Diving into its essential advantage of strong representation capability with CNNs, however, we find this strategy is suboptimal due to two reasons: (1) Motion estimation based on the decoded (often distorted) frame would damage both the spatial structure of motion information inferred and the corresponding residual for each frame, making it difficult to be spatially encoded on the whole image basis using CNNs; (2) Typically, it would break the consistent nature across frames since the estimated motion information is no longer consistent with the movement in the original video due to the distortion in the decoded video, lowering the overall temporal coding efficiency. To overcome these problems, a novel asymmetric Structure-Preserving Motion Estimation (SPME) method is proposed, with the aim to fully explore the ignored original previous frame at the encoder side while complying with the decoded previous frame at the decoder side. Concretely, SPME estimates superior spatially structure-preserving and temporally consistent motion field by aggregating the motion prediction of both the original and the decoded reference frames w.r.t the current frame. Critically, our method can be universally applied to the existing feature prediction based video compression methods. Extensive experiments on several standard test datasets show that our SPME can significantly enhance the state-of-the-art methods.
更多查看译文
关键词
compression,motion,video,structure-preserving
AI 理解论文
溯源树
样例
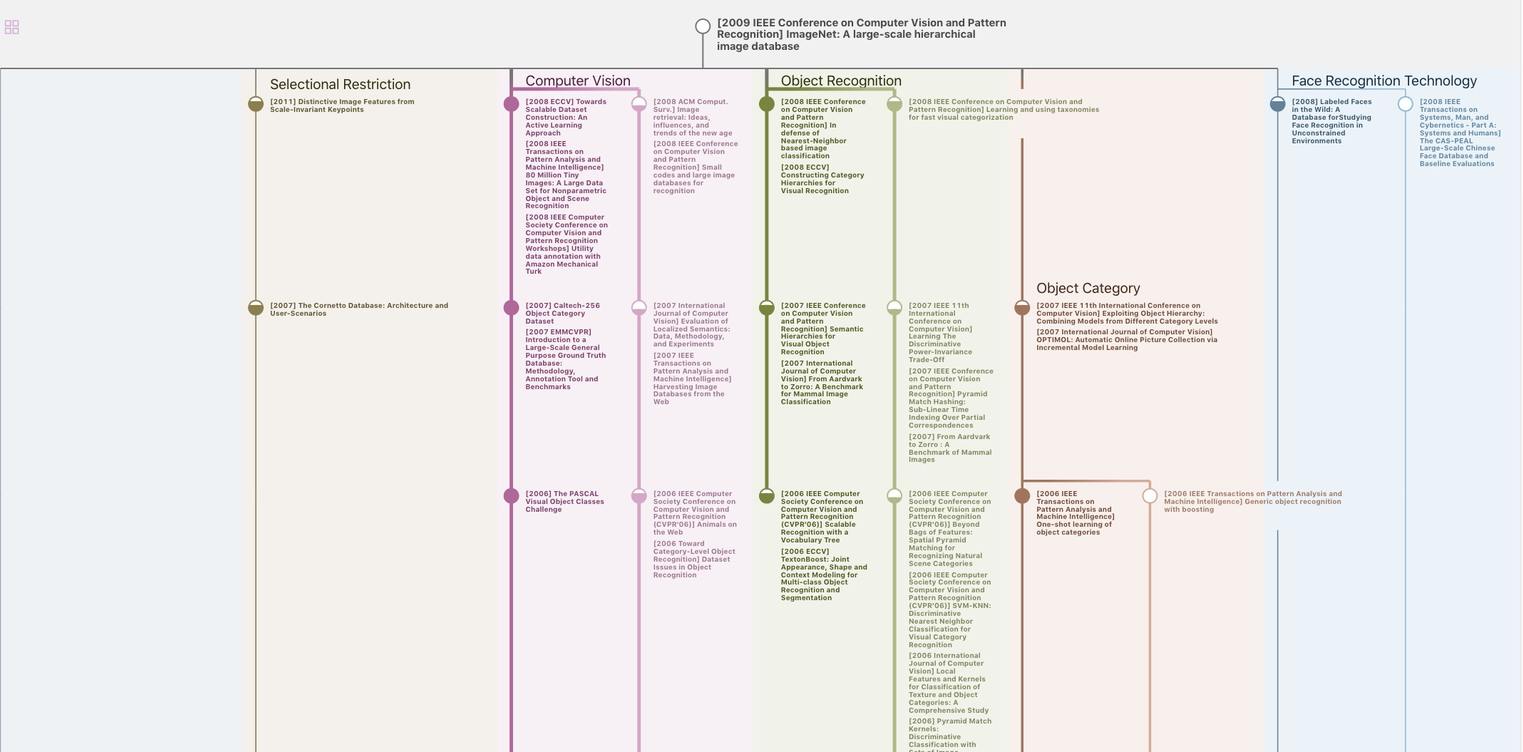
生成溯源树,研究论文发展脉络
Chat Paper
正在生成论文摘要