Eliminating Spatial Ambiguity for Weakly Supervised 3D Object Detection without Spatial Labels
Proceedings of the 30th ACM International Conference on Multimedia(2022)
摘要
ABSTRACTPrevious weakly-supervised methods of 3D object detection in driving scenes mainly rely on spatial labels, which provide the location, dimension, or orientation information. The annotation of 3D spatial labels is time-consuming. There also exist methods that do not require spatial labels, but their detections may fall on object parts rather than entire objects or backgrounds. In this paper, a novel cross-modal weakly-supervised 3D progressive refinement framework (WS3DPR) for 3D object detection that only needs image-level class annotations is introduced. The proposed framework consists of two stages: 1) classification refinement for potential objects localization and 2) regression refinement for spatial pseudo labels reasoning. In the first stage, a region proposal network is trained by cross-modal class knowledge transferred from 2D image to 3D point cloud and class information propagation. In the second stage, the locations, dimensions, and orientations of 3D bounding boxes are further refined with geometric reasoning based on 2D frustum and 3D region. When only image-level class labels are available, proposals with different 3D locations become overlapped in 2D, leading to the misclassification of foreground objects. Therefore, a 2D-3D semantic consistency block is proposed to disentangle different 3D proposals after projection. The overall framework progressively learns features in a coarse to fine manner. Comprehensive experiments on the KITTI3D dataset demonstrate that our method achieves competitive performance compared with previous methods with a lightweight labeling process.
更多查看译文
关键词
spatial ambiguity,detection,supervised,3d
AI 理解论文
溯源树
样例
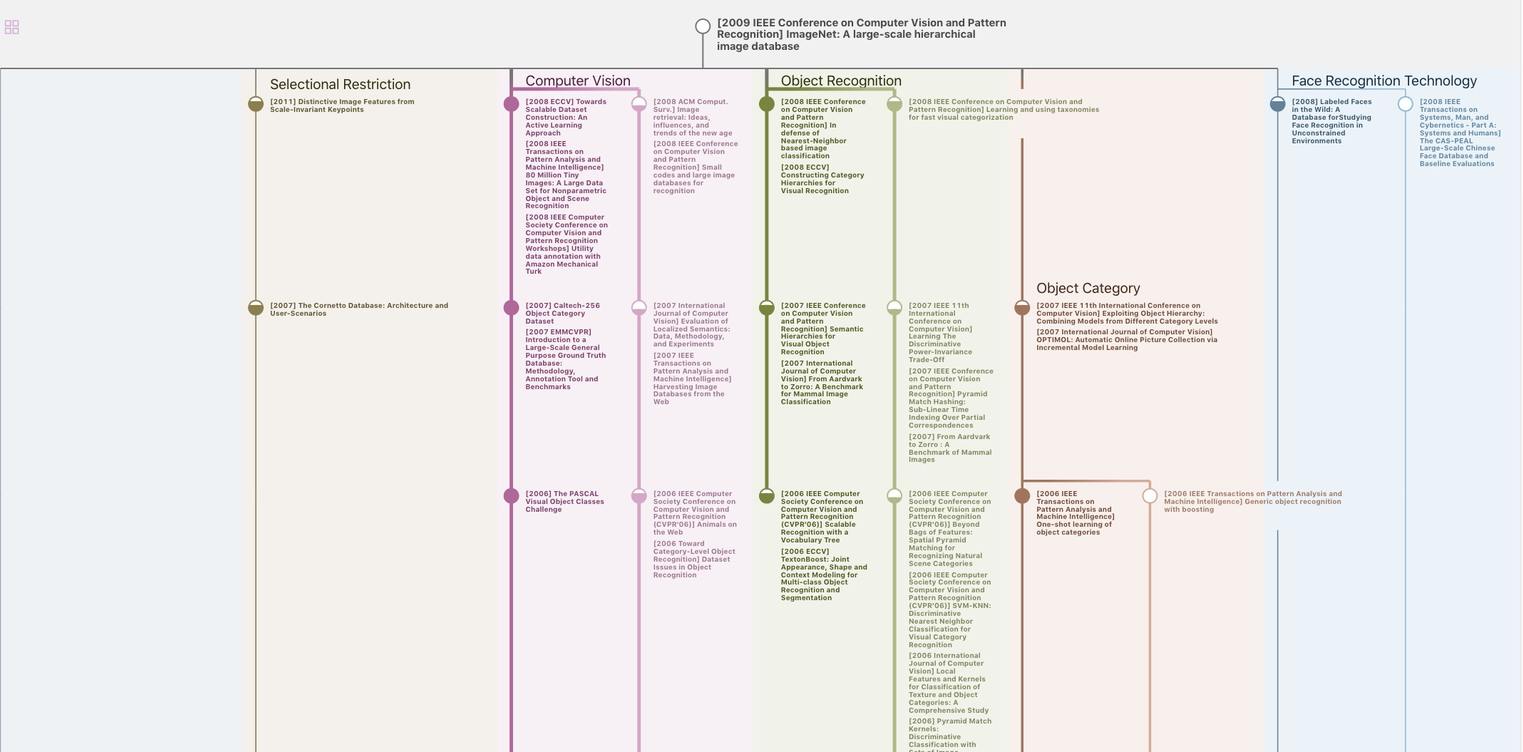
生成溯源树,研究论文发展脉络
Chat Paper
正在生成论文摘要