Improved sales time series predictions using deep neural networks with spatiotemporal dynamic pattern acquisition mechanism
Information Processing & Management(2022)
摘要
The ability to predict product sales is invaluable for improving many of the routine decisions essential for the running of an enterprise. One significant challenge of sales prediction is that it is hard to dynamically capture changing dependent patterns along the sales time line, because sales are often influenced by complicated and changeable market environment. To address this issue, we model sales prediction as a task of multivariate time series (MTS) prediction, and propose a Spatiotemporal Dynamic Pattern Acquisition Mechanism (SDPA), which comprises four components, described below: (1) In the processing of input data: A Spatiotemporal Dynamic Kernel (SDK) component is designed for MTS to effectively capture different dependent correlation patterns during different time periods. (2) In terms of model design: A Simultaneous Regression (SR) component is proposed to dynamically detect stable correlations by using co-integration based dynamic programming over different time periods. (3) A novel Hierarchical Attention (HA) component is designed to incorporate SDK to detect spatiotemporal attention patterns from the captured dynamic correlations. (4) In the design of loss function, A Change Sensitive and Alignment component (DC) is proposed to provide more future information based on future trend correlations for better model training. The four components are incorporated into a unified framework by considering Homovariance Uncertainty (HU). This is referred to as SDPANet and contributes to model training and sales prediction. Extensive experiments were conducted on two real-world datasets: Galanz and Cainiao, and experimental results show that the proposed method achieves statistically significant improvements compared to the most state-of-the-art baselines, with average 41.5% reduction on RMAE, average 39.5% reduction on RRSE and average 46% improvement on CORR. Experiments are also conducted on two new datasets, which are Traffic and Exchange-Rate from other fields, to further verify the effectiveness of the proposed model. Case studies show that the model is capable of capturing dynamic changing patterns and of predicting future sales trends with greater accuracy.
更多查看译文
关键词
Sales prediction,Spatiotemporal dynamic kernel,Simultaneous regression,Hierarchical attention,Dynamic detection and alignment
AI 理解论文
溯源树
样例
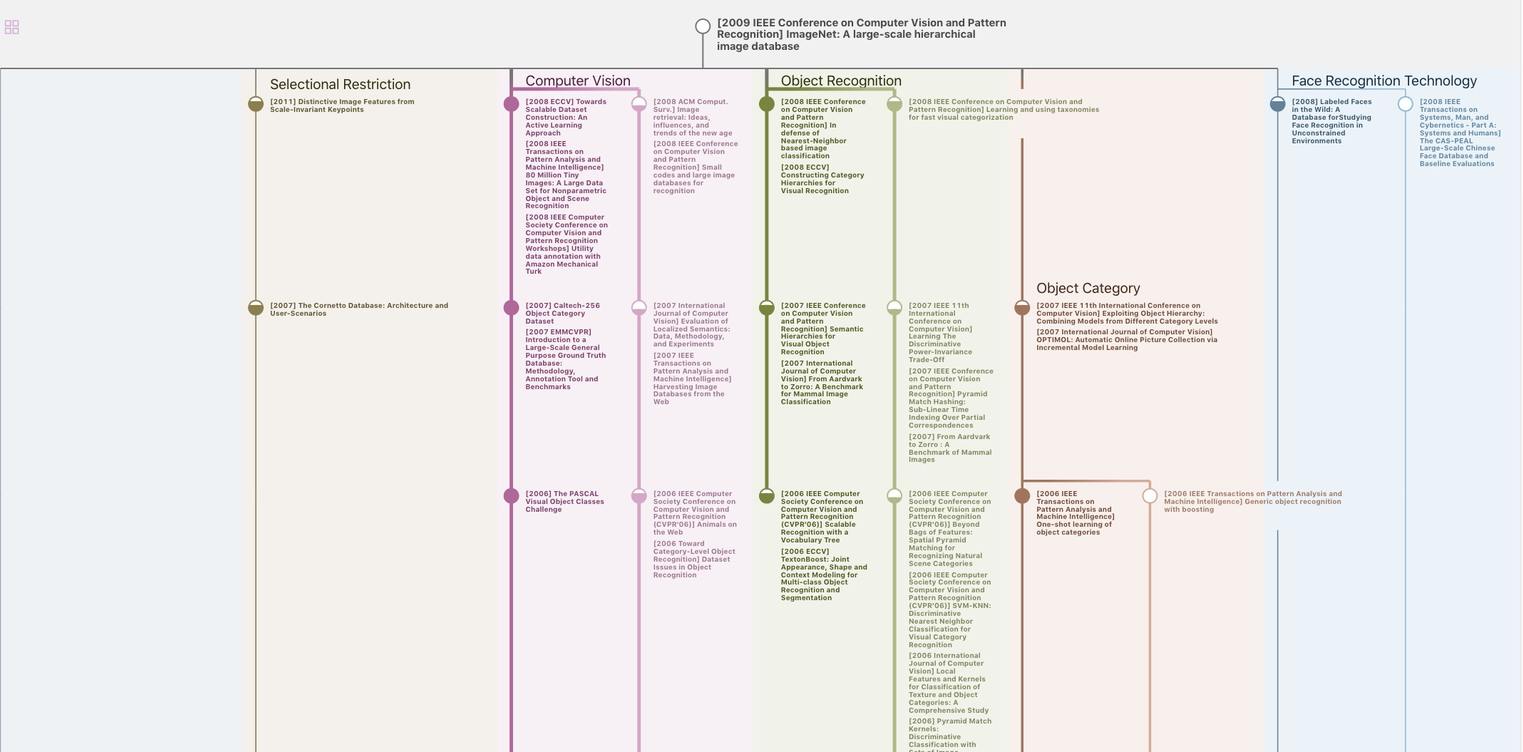
生成溯源树,研究论文发展脉络
Chat Paper
正在生成论文摘要