Bayesian inductive learning in group recommendations for seen and unseen groups
Information Sciences(2022)
摘要
Group recommendation refers to a recommendation of items to a group of users (i.e., members). When predicting relevant items, a model commonly faces unseen groups that do not appear in the training step. Recently, deep neural networks and an attention mechanism were applied to group recommendations by aggregating user preferences. However, current methods are insufficient to handle unseen groups (i.e., transductive models) or struggle to compute cost-effective attention networks and regularizations. In this study, we propose the novel Bayesian inductive learning method, called IndiG, for making recommendations to seen and unseen groups. To learn inductively, a function distribution consisting of efficient attention-based aggregation is used as shared information across groups. By incorporating a transductive model as a posterior into the proposed Bayesian method, an inductive model as a prior can learn robustly. We adopt cost-effective regularization to prevent degenerated solutions by maximizing a correlation between group representations of a transductive model and an inductive model, while decorrelating dimensions of group representations. Through experiments, we demonstrated that the proposed method outperformed other existing methods. The experiments also showed that the utilization of uncertainty on the predicted ratings of items worked effectively to improve the performance.
更多查看译文
关键词
Group recommendation,Unseen group,Bayesian inductive learning,Regularization
AI 理解论文
溯源树
样例
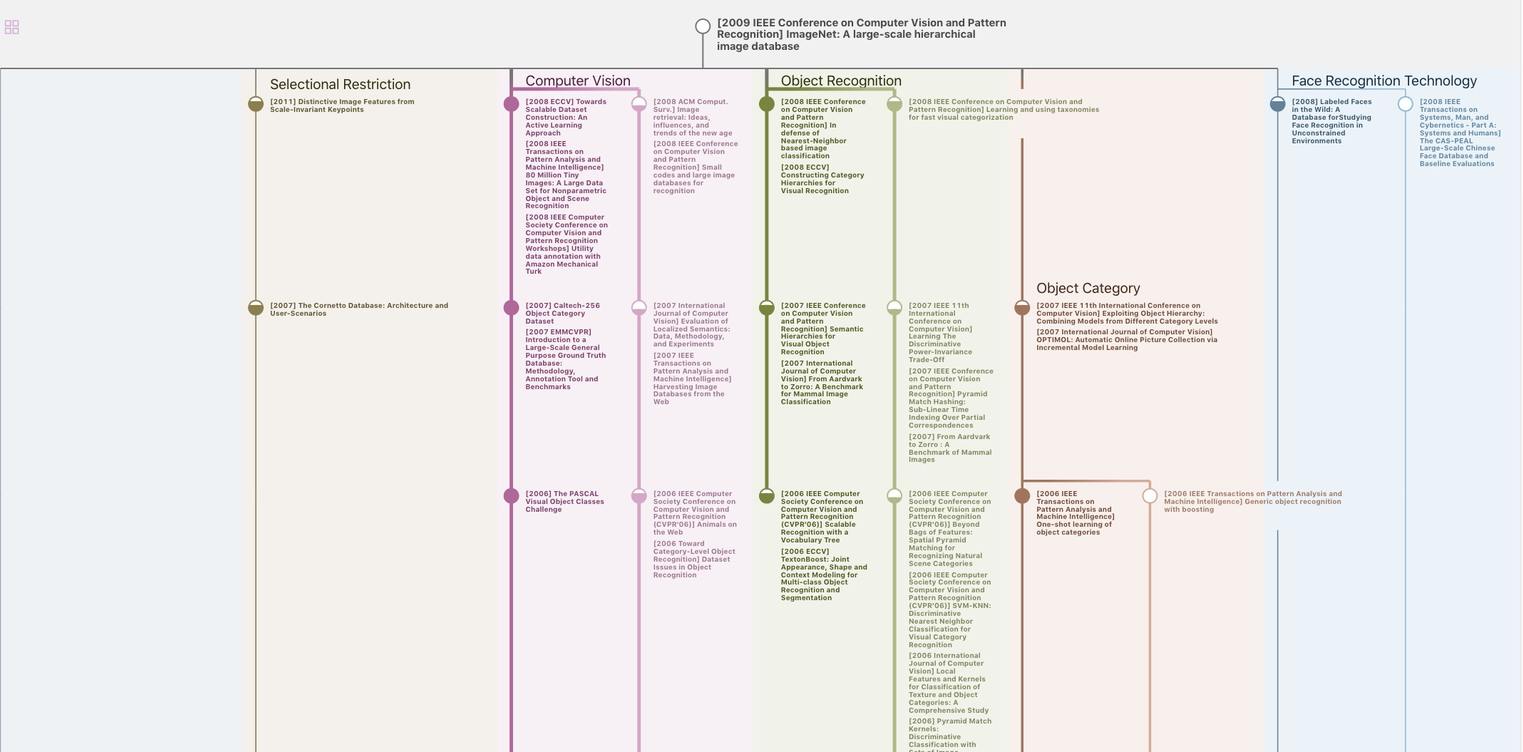
生成溯源树,研究论文发展脉络
Chat Paper
正在生成论文摘要