What constrains food webs? A maximum entropy framework for predicting their structure with minimal biases
PLoS Computational Biology(2023)
摘要
Food webs are complex ecological networks whose structure is both ecologically and statistically constrained, with many network properties being correlated with each other. Despite the recognition of these invariable relationships in food webs, the use of the principle of maximum entropy (MaxEnt) in network ecology is still rare. This is surprising considering that MaxEnt is a statistical tool precisely designed for understanding and predicting many types of constrained systems. This principle asserts that the least-biased probability distribution of a system's property, constrained by prior knowledge about that system, is the one with maximum information entropy. MaxEnt has been proven useful in many ecological modeling problems, but its application in food webs and other ecological networks is limited. Here we show how MaxEnt can be used to derive many food-web properties both analytically and heuristically. First, we show how the joint degree distribution (the joint probability distribution of the numbers of prey and predators for each species in the network) can be derived analytically using the number of species and the number of interactions in food webs. Second, we present a heuristic and flexible approach of finding a network's adjacency matrix (the network's representation in matrix format) based on simulated annealing and SVD entropy. We built two heuristic models using the connectance and the joint degree sequence as statistical constraints, respectively. We compared both models' predictions against corresponding null and neutral models commonly used in network ecology using open access data of terrestrial and aquatic food webs sampled globally (N = 257). We found that the heuristic model constrained by the joint degree sequence was a good predictor of many measures of food-web structure, especially the nestedness and motifs distribution. Specifically, our results suggest that the structure of terrestrial and aquatic food webs is mainly driven by their joint degree distribution. Predator and prey species form complex networks of energy flow that drive the functioning and dynamics of ecosystems. Because of their high number and variability, sampling and documenting these feeding relationships are particularly challenging, especially at large spatial and temporal scales. Fortunately, many properties of food webs can be studied without knowing every trophic interaction in a network by using suitable computational methods. This is notably the case of their emerging structure, which could be driven by just a few ecological and biological variables despite the large number of mechanisms shaping ecological networks. In this contribution, we present a novel framework to identify these variables by applying the principle of maximum entropy to the analysis of food-web structure. We show that the number of prey and predators for each species in a food web is a fundamental property that shapes many aspects of the network, while also being predictable by our model and available data. Our approach, which can be applied to a wide range of biological and ecological networks, can be used to make better predictions of food webs across space, in addition to contributing to our understanding of the ecological processes shaping predatory interactions at the community level.
更多查看译文
AI 理解论文
溯源树
样例
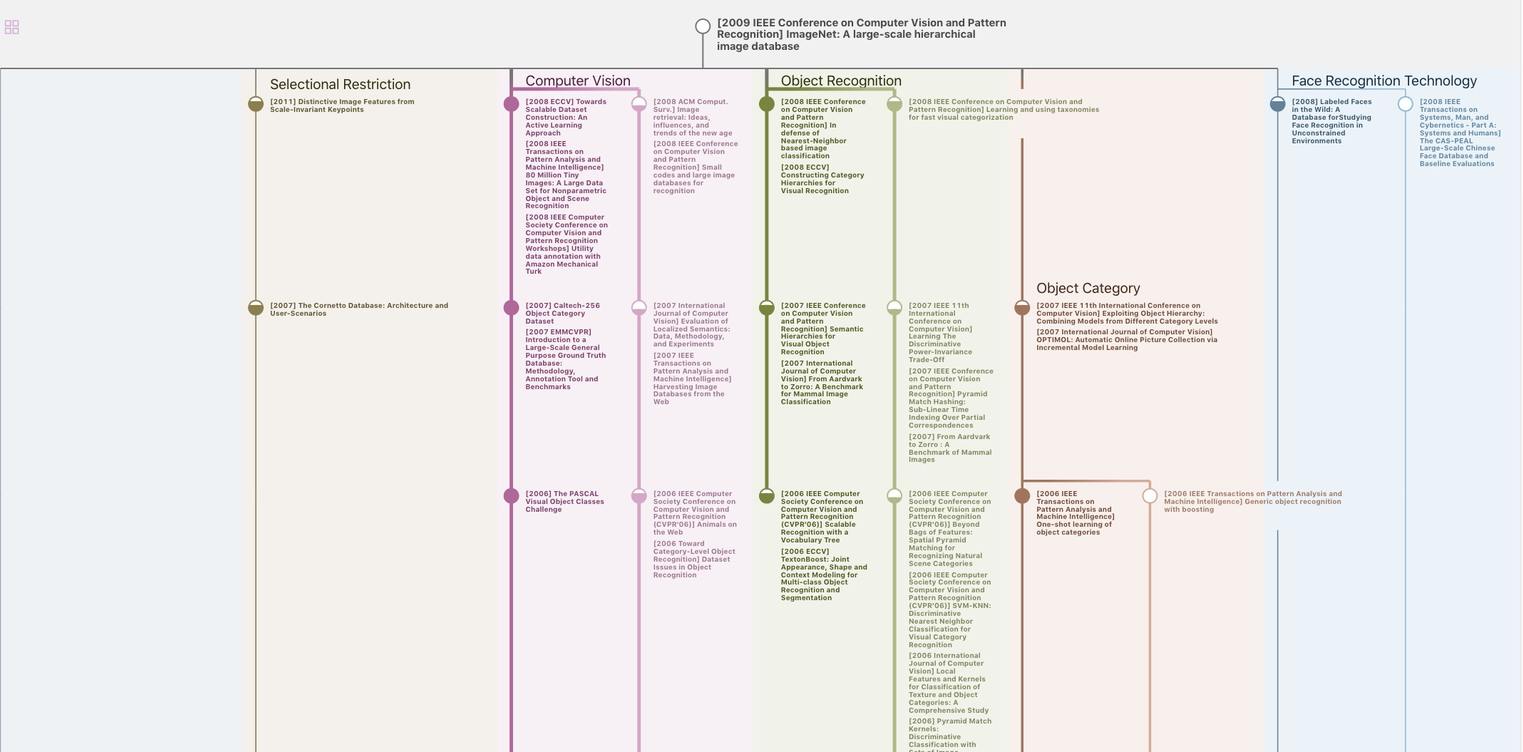
生成溯源树,研究论文发展脉络
Chat Paper
正在生成论文摘要