Siamese visual tracking combining granular level multi-scale features and global information
Knowledge-Based Systems(2022)
摘要
Despite the great success achieved in visual tracking, it is still hard for most trackers to address scenes with targets subject to large-scale changes and similar objects. The capacity of existing methods is first insufficient to efficiently extract multi-scale features. Then, convolutional neural networks focus primarily on local characteristics while easily ignoring global characteristics, which is essential for visual tracking. Furthermore, the recently popular tracking methods based on Siamese-like networks can perform the image matching of two branches through simple cross-correlation operations, and cannot effectively establish their connection. An improved Siamese tracking network, called GSiamMS, is proposed to address these challenges via the integration of Res2Net blocks and transformer modules. Within this network, a feature extraction module based on Res2Net blocks is constructed to obtain multi-scale information from the granular level without relying on multi-layer outputs. Then, the cross-attention mechanism is utilized to learn the connection between template features and search features while the self-attention mechanism focusing on the global information establishes long-range dependencies between the object and the background. Finally, numerous experiments on visual tracking benchmarks including TrackingNet, GOT-10k, LaSOT, NFS, UAV123, and TNL2K are implemented to verify that the developed method running at 38fps achieves the superior performance compared with several state-of-the-art methods.
更多查看译文
关键词
Visual tracking,Siamese network,Multi-scale feature,Self attention,Transformer
AI 理解论文
溯源树
样例
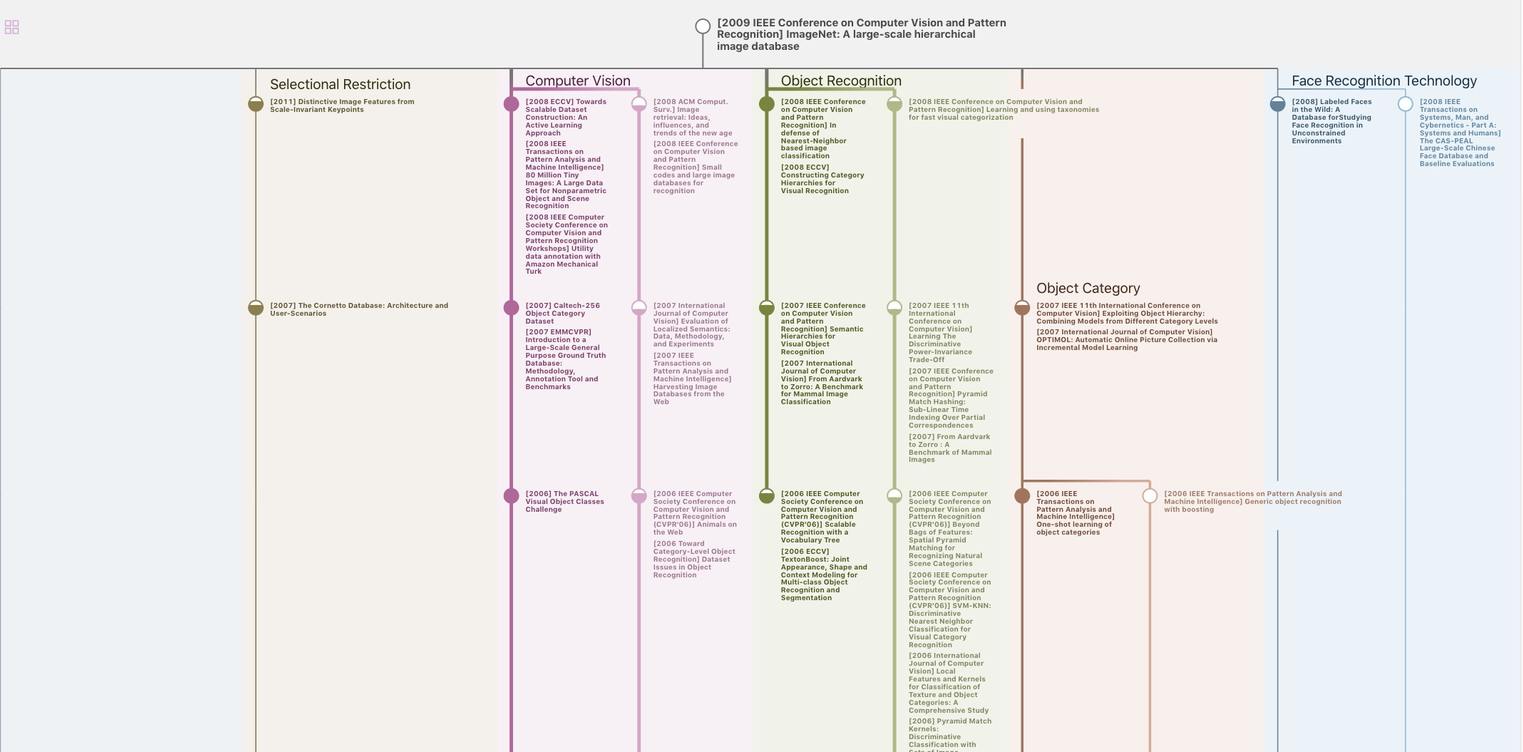
生成溯源树,研究论文发展脉络
Chat Paper
正在生成论文摘要