Evaluation of machine learning approaches for surface water monitoring using Sentinel-1 data
Journal of Applied Remote Sensing(2022)
摘要
The monitoring of mosquito breeding habitats requires the production of surface water maps on a regular basis and at a high-resolution using mapping algorithms. To map surface water, several machine learning (ML) algorithms were evaluated, taking advantage of frequently available synthetic aperture radar imagery from the Sentinel-1 mission with a 10-m spatial resolution and a large dataset of field observations of the water state (inundated/dry) in rice paddies and wetlands. One-class support vector machine, one-class self-organizing map, and multilayer perceptron with automatic relevance determination (MLP-ARD) algorithms were trained and assessed to examine their accuracy in detecting surface water. Results show the robustness of the MLP-ARD algorithm, which provides an overall accuracy of 0.974 for a single date and 0.892 for a 5-month study period from May to September. The accuracy of water detection was found to be mainly affected by the presence of dense and high vegetation in inundated fields and the presence of floating vegetation or algae. (c) 2022 Society of Photo-Optical Instrumentation Engineers (SPIE)
更多查看译文
关键词
Sentinel-1, surface water, support vector machine, one-class classifier, multilayer, perceptron with automatic relevance determination
AI 理解论文
溯源树
样例
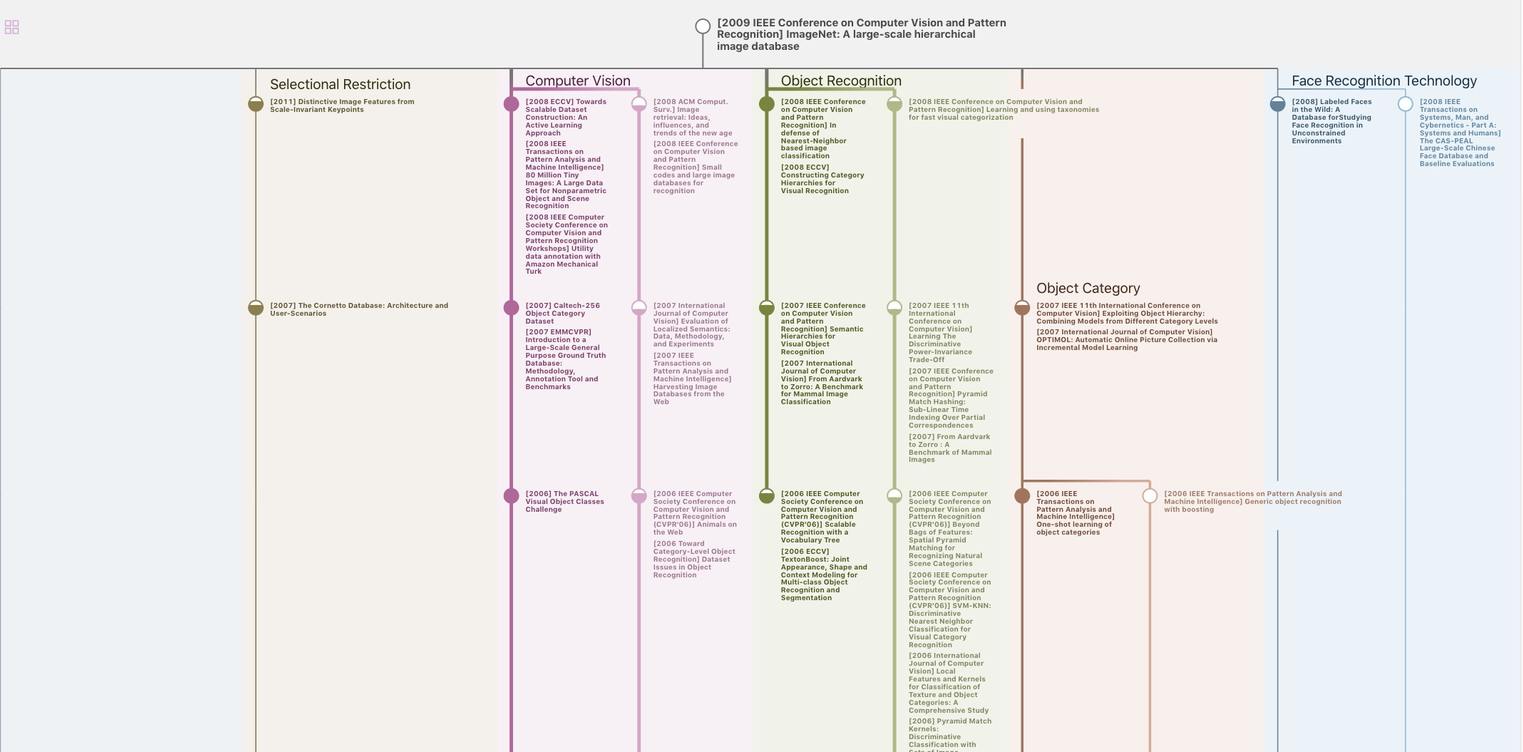
生成溯源树,研究论文发展脉络
Chat Paper
正在生成论文摘要