A reliability analysis method based on adaptive Kriging and partial least squares
Probabilistic Engineering Mechanics(2022)
摘要
In recent years, reliability analysis based on adaptive Kriging (AK) has been extensively studied. However, constructing the Kriging model of high-dimensional systems during adaptive learning faces huge computational challenges. This paper combines partial least squares (PLS) with AK to develop an efficient method for reliability analysis of high-dimensional systems, which is named as PLS-AK. The proposed method takes PLS algorithm as the dimensionality reduction (DR) technique of high-dimensional input variables. Then, in the reduced space of input principal components (PCs), a low-dimensional Kriging model is constructed with U learning function for reliability analysis. Since PLS usually requires adequate samples to determine the latent relationship between input and output variables, using too many initial samples before adaptive learning will weaken the advantages of AK. To avoid this problem, this paper also proposes a synchronous updating method for both the projection direction and AK model. In this way, only a few initial samples are required to determine the initial input projection, and the input projection will be updated and converge gradually as the number of training samples increases. At last, three examples can prove the effectiveness of the proposed PLS-AK method.
更多查看译文
关键词
Kriging model,Reliability analysis,Partial least squares (PLS),Adaptive learning,Dimensionality reduction (DR)
AI 理解论文
溯源树
样例
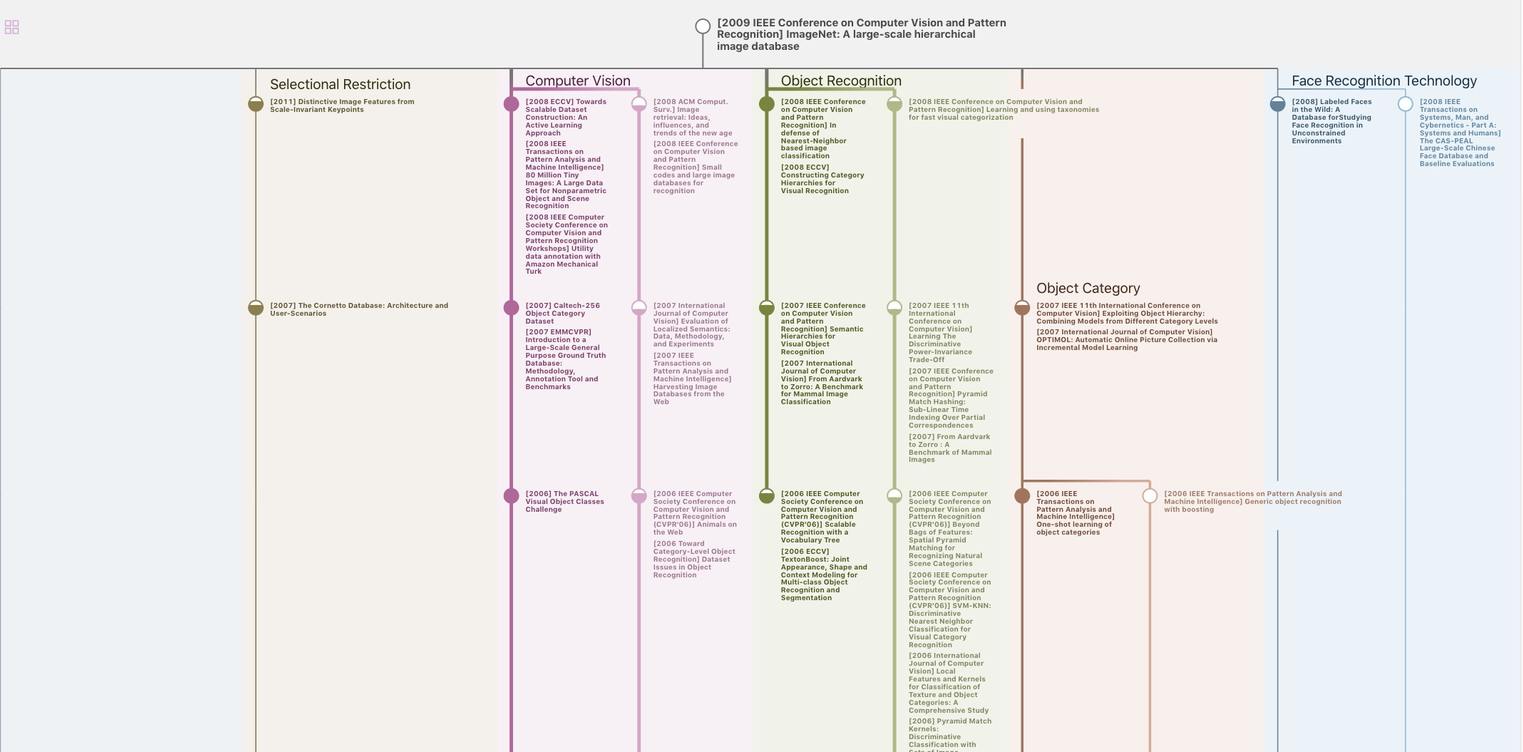
生成溯源树,研究论文发展脉络
Chat Paper
正在生成论文摘要